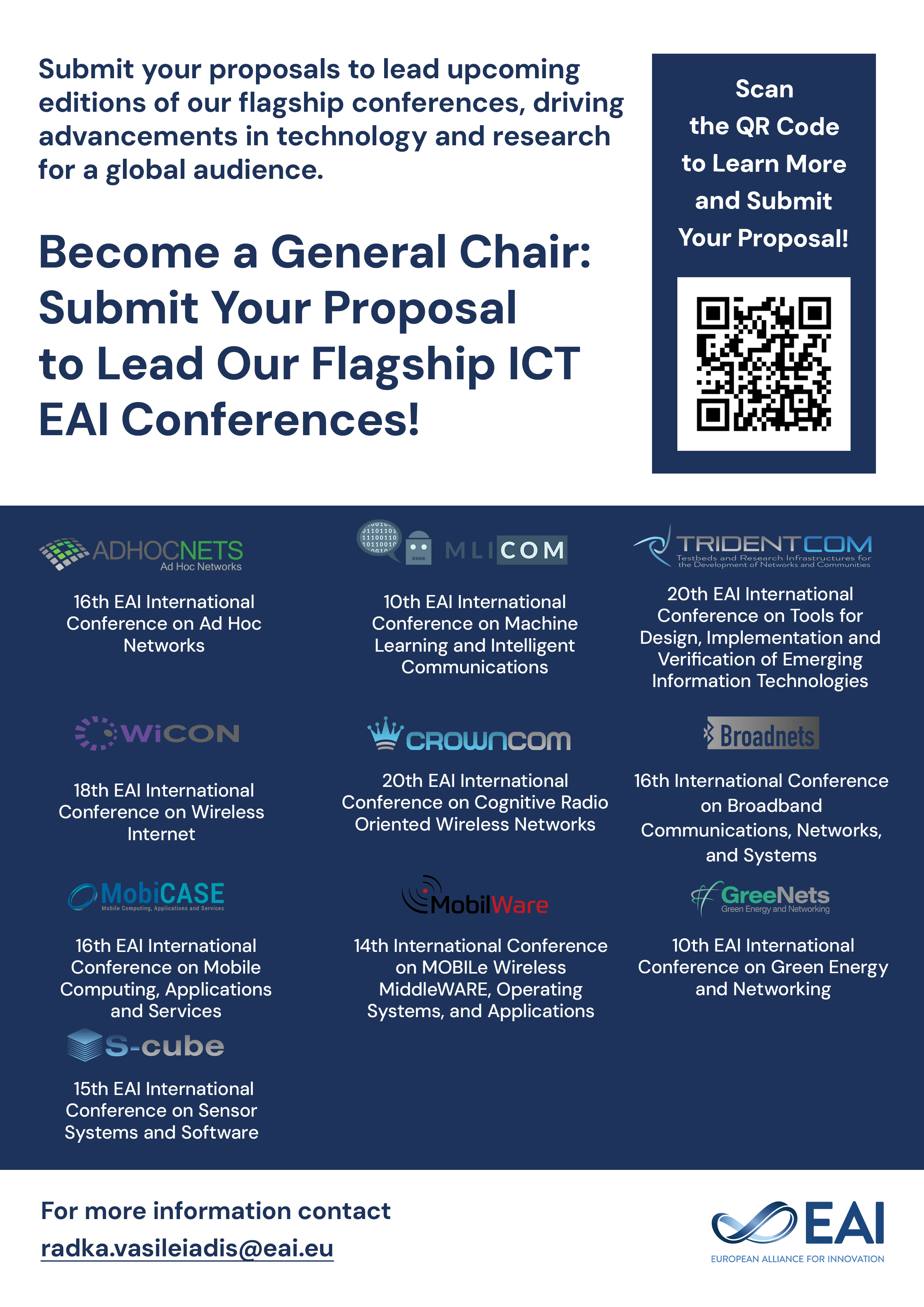
Research Article
Mitigating Training Bias in Cattle Breed Identification through Orientation-Aware Framework
@INPROCEEDINGS{10.4108/eai.23-11-2023.2343289, author={Vijayalakshmi A and Shanmugavadivu P and Vijayalakshmi S and Shreyansh Padarha and Sivaranjani R}, title={Mitigating Training Bias in Cattle Breed Identification through Orientation-Aware Framework}, proceedings={Proceedings of the 1st International Conference on Artificial Intelligence, Communication, IoT, Data Engineering and Security, IACIDS 2023, 23-25 November 2023, Lavasa, Pune, India}, publisher={EAI}, proceedings_a={IACIDS}, year={2024}, month={3}, keywords={orientation labelling data augmentation convolutional neural networks (cnn) cattle breed identification}, doi={10.4108/eai.23-11-2023.2343289} }
- Vijayalakshmi A
Shanmugavadivu P
Vijayalakshmi S
Shreyansh Padarha
Sivaranjani R
Year: 2024
Mitigating Training Bias in Cattle Breed Identification through Orientation-Aware Framework
IACIDS
EAI
DOI: 10.4108/eai.23-11-2023.2343289
Abstract
This paper introduces a comprehensive strategy to mitigate training bias in cattle breed identification models, focusing on cow orientation in still frames. Leveraging YOLOv7 for face detection, our methodology incorporates a novel orientation-aware pre-processing step that categorizes images into right-oriented, left-oriented, original, and original with inverted orientations. The breed identification model, based on Convolutional Neural Networks (CNN), is trained on this augmented dataset. While the left-oriented approach achieves the highest accuracy, the original and inverted orientation strategy demonstrates superior validation accuracy, showcasing its effectiveness in addressing bias during real-world applications with varied cow orientations. These findings underscore the importance of orientation-aware training for robust cattle breed identification, providing a solution for an overlooked niche