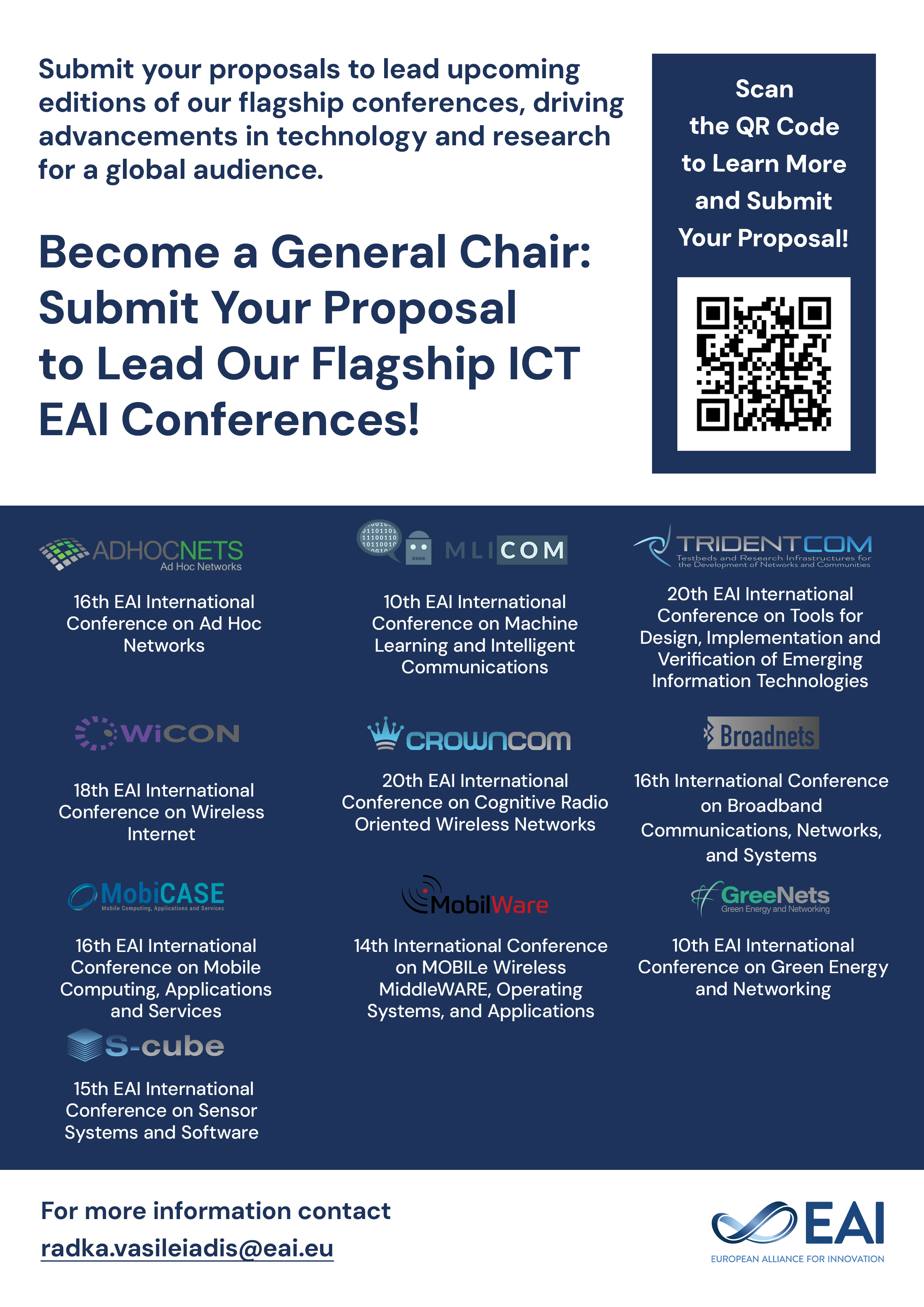
Research Article
Synthesizing MRI Images Using Generative AI and Image Style Transfer GAN
@INPROCEEDINGS{10.4108/eai.23-11-2023.2343219, author={Chithra PL. and Dhivya S.D}, title={Synthesizing MRI Images Using Generative AI and Image Style Transfer GAN}, proceedings={Proceedings of the 1st International Conference on Artificial Intelligence, Communication, IoT, Data Engineering and Security, IACIDS 2023, 23-25 November 2023, Lavasa, Pune, India}, publisher={EAI}, proceedings_a={IACIDS}, year={2024}, month={3}, keywords={generative artificial intelligence dcgan pix2pixgan image style transfer gan modalities class imbalance}, doi={10.4108/eai.23-11-2023.2343219} }
- Chithra PL.
Dhivya S.D
Year: 2024
Synthesizing MRI Images Using Generative AI and Image Style Transfer GAN
IACIDS
EAI
DOI: 10.4108/eai.23-11-2023.2343219
Abstract
This work proposes to realistically synthesize the missing MRI sequence using generative artificial intelligence (GAI). GAI framework learns the patterns, data distribution, and structure of the input MRI images by using training data with those MRI images and then generates new data with similar data distribution as input MRI images. In this proposed work, Deep Convolution Generative Adversarial Network (DCGAN) and Pix2PixGAN generate a synthesized image using a real input image and random noise drawn from the dataset distribution. Its output is approximated to the original image using Image Style Transfer GAN, which gives an impressive outcome with an accuracy of 95.6%. Accuracy and loss are assessed using the performance metrices, for content and style images and the data distribution of real and fake images of DCGAN and Pix2Pix GAN. To treat brain tumor patients, it is vital to examine all four modalities, such as T1, T2, T1w, and Flair, which are often missing due to image artifacts and time constraints. These missing brain tumor modalities lead to difficulties while treating patients, creating class imbalance and reducing performance and accuracy while training the dataset for classification and segmentation in deep learning and machine learning.