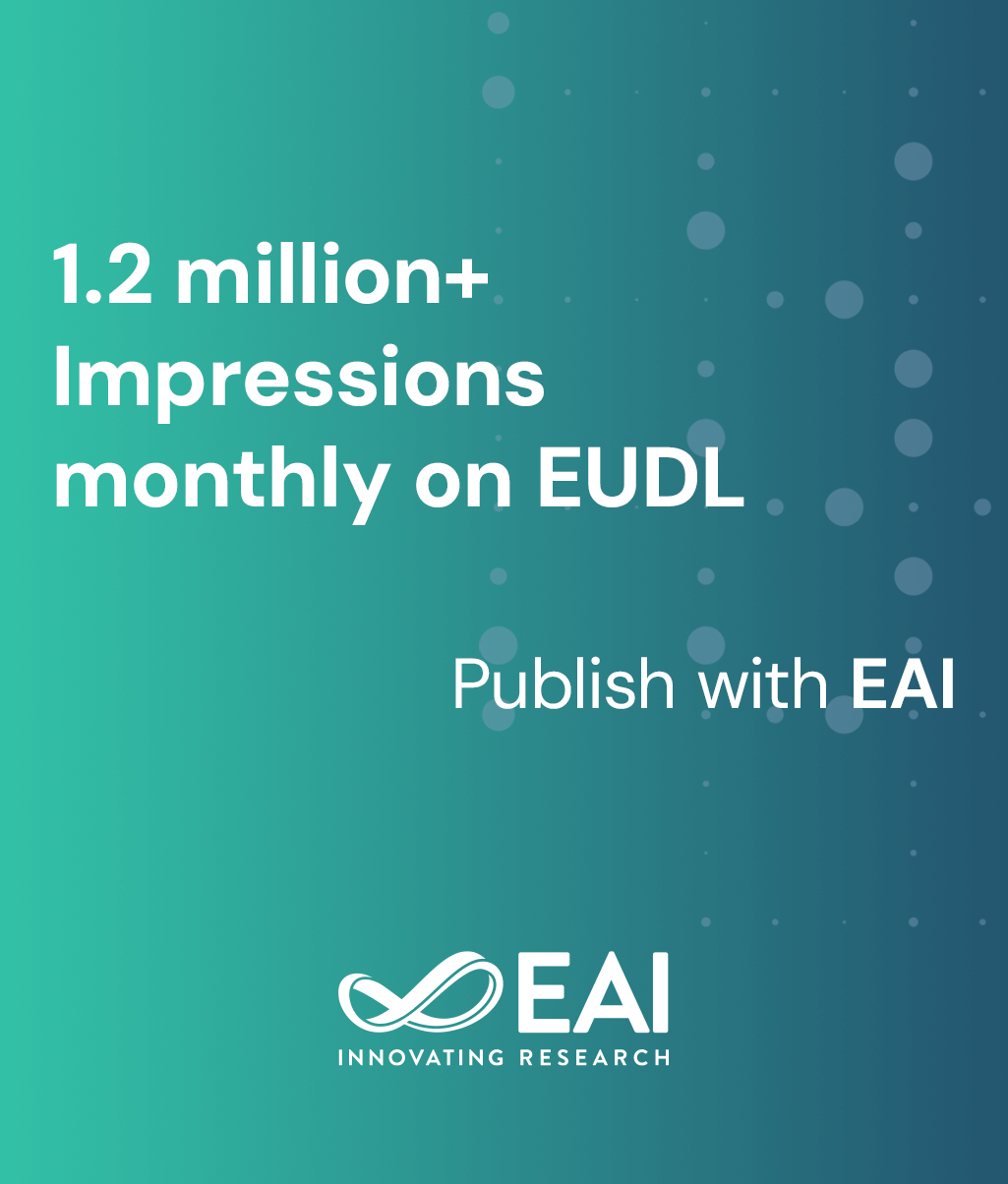
Research Article
Convolutional Neural Network Model for Stratification of LiDAR 3D Point Cloud Data
@INPROCEEDINGS{10.4108/eai.23-11-2023.2343218, author={Chithra PL and Lakshmi Bala S}, title={Convolutional Neural Network Model for Stratification of LiDAR 3D Point Cloud Data}, proceedings={Proceedings of the 1st International Conference on Artificial Intelligence, Communication, IoT, Data Engineering and Security, IACIDS 2023, 23-25 November 2023, Lavasa, Pune, India}, publisher={EAI}, proceedings_a={IACIDS}, year={2024}, month={3}, keywords={3d point cloud data lidar contrastive learning self-supervised learning dynamicedgeconv outlier detection}, doi={10.4108/eai.23-11-2023.2343218} }
- Chithra PL
Lakshmi Bala S
Year: 2024
Convolutional Neural Network Model for Stratification of LiDAR 3D Point Cloud Data
IACIDS
EAI
DOI: 10.4108/eai.23-11-2023.2343218
Abstract
Analysis of the 3D LiDAR point cloud data is the fundamental for computer vision and robotics, with potential applications ranging from object detection to scene understanding. In this proposed work, introduced an approach that leverages the power of contrastive learning combined with self-supervised learning for feature extraction from the LiDAR 3D point cloud data. On-the-fly extensions to include rotation on invariance of 3D point cloud data, jitter adding noise in coordinates of point cloud data, and displacement and shear to improve model robustness and invariance to geometric transformations. Each data point is paired with two extensions to form positive pairs and facilitating the contrastive learning. The focus of proposed method is to implement the point cloud feature learning layer, specifically on DynamicEdgeConv. This layer uses the parameter 'k' to identify nearest neighbors in 3D point cloud data that together create a subgraph, facilitating improved feature learning. Evaluation results determines the efficacy of proposed approach and that shows that significant improvements in clustering performance and outlier detection accuracy compared to common methods. This work has advances of the state of self-supervised learning of LiDAR 3D point cloud data, but also paves the way for more robust 3D object detection, scene understanding, and applications in the fields of robotics.