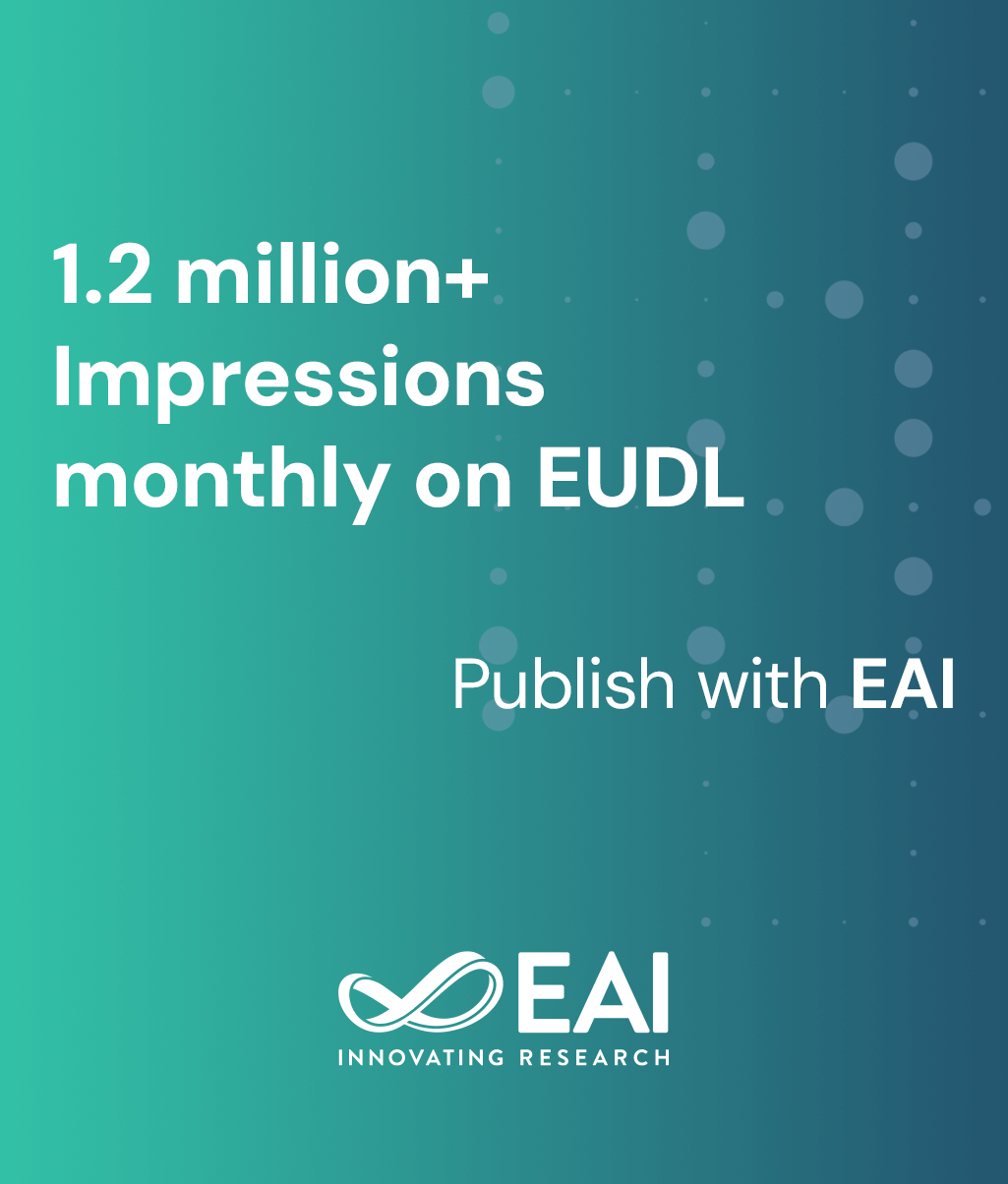
Research Article
Data-Driven Based Model For Predictive Maintenance Applications In Industrial System
@INPROCEEDINGS{10.4108/eai.23-11-2022.2341596, author={Marza Ihsan Marzuki and Soni Prayogi and Muhammad Abdillah}, title={Data-Driven Based Model For Predictive Maintenance Applications In Industrial System }, proceedings={Proceedings of the International Conference on Sustainable Engineering, Infrastructure and Development, ICO-SEID 2022, 23-24 November 2022, Jakarta, Indonesia}, publisher={EAI}, proceedings_a={ICO-SEID}, year={2023}, month={12}, keywords={predictive maintenance gradient-boosted trees machine learning random forest data driven based model}, doi={10.4108/eai.23-11-2022.2341596} }
- Marza Ihsan Marzuki
Soni Prayogi
Muhammad Abdillah
Year: 2023
Data-Driven Based Model For Predictive Maintenance Applications In Industrial System
ICO-SEID
EAI
DOI: 10.4108/eai.23-11-2022.2341596
Abstract
Predictive maintenance involves using data to anticipate when equipment will need maintenance, rather than adhering to a set schedule or reacting to equipment breakdowns. It is a proactive approach to maintenance that aims to prevent issues before they occur. This approach has the potential to improve the efficiency and effectiveness of maintenance operations, reducing downtime and maintenance costs and improving equipment performance and reliability. In this paper, we proposed a model to predict machine failure based on a synthetic dataset from the UCI machine learning repository. We used the gradient-boosted trees (GBT) method to model the PdM application and found that the model had an accuracy of 97.3%. We also compared the performance of the GBT model to other machine learning algorithms, including random forests (RF) and support vector machines (SVM), and found that the GBT model had the highest accuracy. These results suggest that GBT is a valuable tool for implementing PdM and improving the efficiency and effectiveness of maintenance operations.