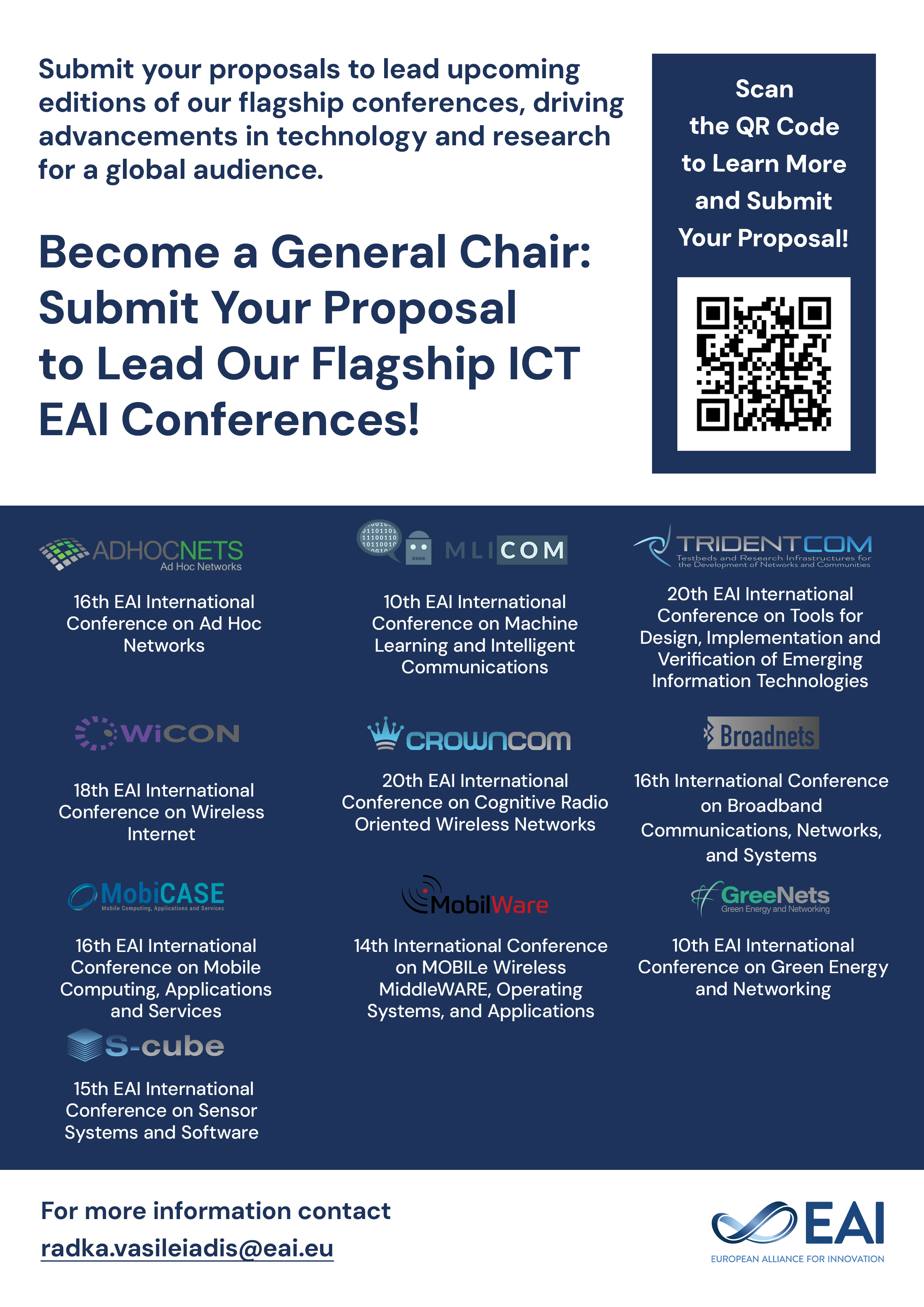
Research Article
This Malware Looks Familiar: Laymen Identify Malware Run-time Similarity with Chernoff faces and Stick Figures
@INPROCEEDINGS{10.4108/eai.22-3-2017.152417, author={Nathan VanHoudnos and William Casey and David French and Brian Lindauer and Eliezer Kanal and Evan Wright and Bronwyn Woods and Seungwhan Moon and Peter Jansen and Jamie Carbonell}, title={This Malware Looks Familiar: Laymen Identify Malware Run-time Similarity with Chernoff faces and Stick Figures}, proceedings={10th EAI International Conference on Bio-inspired Information and Communications Technologies (formerly BIONETICS)}, publisher={EAI}, proceedings_a={BICT}, year={2017}, month={3}, keywords={malware classification chernoff faces active learning machine learning}, doi={10.4108/eai.22-3-2017.152417} }
- Nathan VanHoudnos
William Casey
David French
Brian Lindauer
Eliezer Kanal
Evan Wright
Bronwyn Woods
Seungwhan Moon
Peter Jansen
Jamie Carbonell
Year: 2017
This Malware Looks Familiar: Laymen Identify Malware Run-time Similarity with Chernoff faces and Stick Figures
BICT
EAI
DOI: 10.4108/eai.22-3-2017.152417
Abstract
Classifying unknown malicious binaries into malware families provides valuable information to security professionals. The reverse engineering necessary to classify a given binary into a known family, however, is expensive because the time of the human expert is expensive. In this work, we give a proof-of-concept approach to visualizing malware so that non-experts are able to distinguish between three heterogenous families of malware with minimal training. We present this work as a first step towards a human in the loop active learning system for malware analysis. To do so we curated a dataset of malware variants and labeled them using expert malware reverse engineering, instrumented runtime behavior of these malware variants, constructed a simple, graph based feature set from the runtime behavior, and visualized low-dimensional representations of these system call graphs with stick figures and Chernoff faces. We then selected the three families with the largest within family variation and asked non-experts on Amazon Mechanical Turk to classify binaries between these three families using the generated visual representations. We found that non-experts completed the task with between 63% and 86% accuracy, and when aggregated, these non-expert labels successfully trained a classifier to a similar level of performance as the ground truth labels. Moreover, the information from the experiments yielded new insights into the variation within one of the malware families.