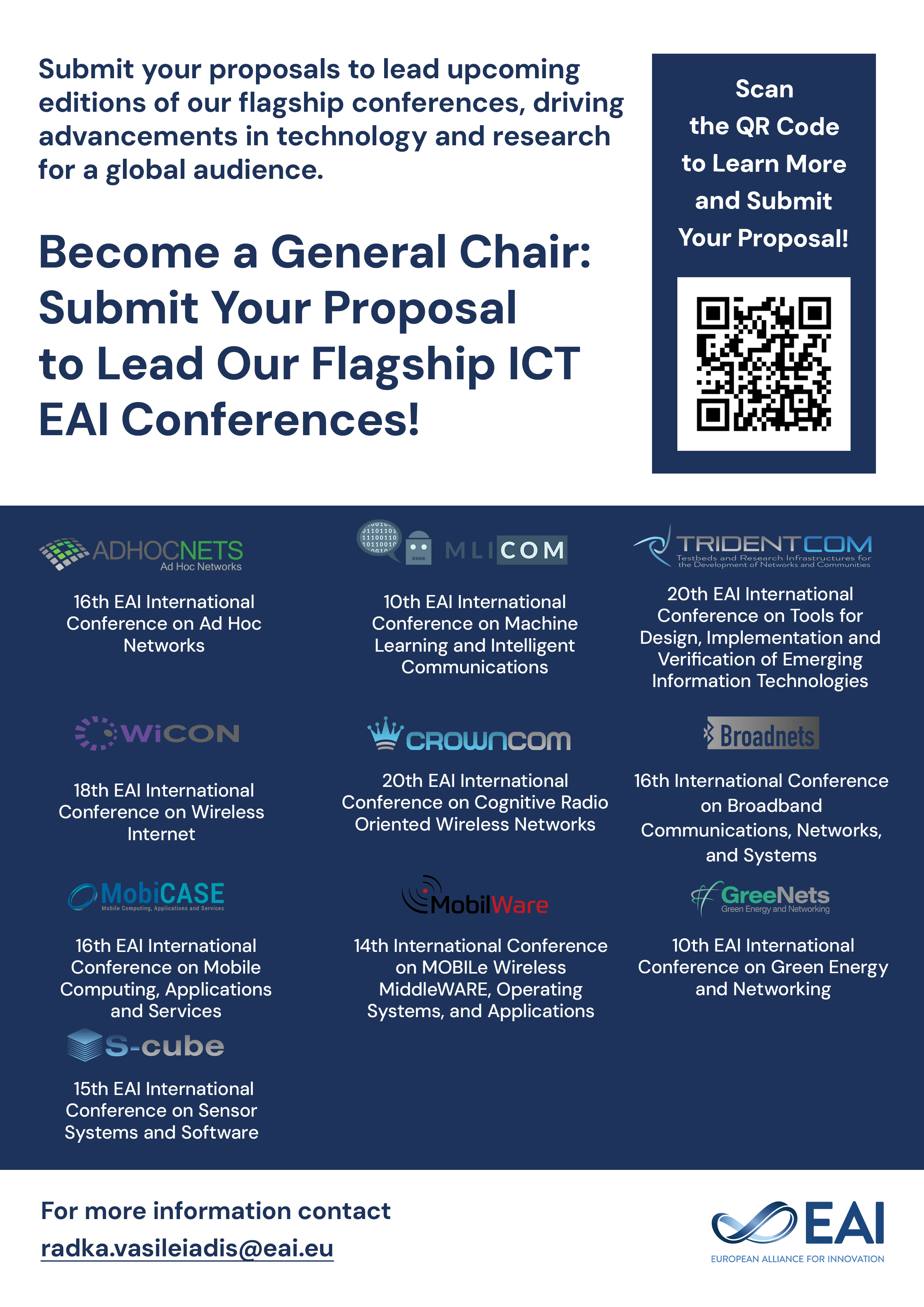
Research Article
Efficient Feature Vector Clustering for Automatic Speech Recognition Systems
@INPROCEEDINGS{10.4108/eai.22-3-2017.152399, author={Lilia Lazli and Mounir Boukadoum and Otmane Ait Mohamed and Mohamed-Tayeb Laskri}, title={Efficient Feature Vector Clustering for Automatic Speech Recognition Systems}, proceedings={10th EAI International Conference on Bio-inspired Information and Communications Technologies (formerly BIONETICS)}, publisher={EAI}, proceedings_a={BICT}, year={2017}, month={3}, keywords={unsupervised speech clustering genetic algorithm fuzzy c-means algorithm speech recognition system hmm/mlp}, doi={10.4108/eai.22-3-2017.152399} }
- Lilia Lazli
Mounir Boukadoum
Otmane Ait Mohamed
Mohamed-Tayeb Laskri
Year: 2017
Efficient Feature Vector Clustering for Automatic Speech Recognition Systems
BICT
EAI
DOI: 10.4108/eai.22-3-2017.152399
Abstract
In this paper, we present an efficient algorithm for the clustering of speech data. The algorithm based on regulating a similarity measure to set the number of clusters and the cluster boundaries, thus overcoming the shortcomings of conventional clustering algorithms such as k-Means and Fuzzy C-Means, which require a priori knowledge of the number of clusters, the use of similarity measure that follows the data distribution, and are sensitive to the choice of initial configuration, The algorithm performance was tested in an HMM/MLP automatic speech recognition system, with the results were compared with those obtained when using a combination of Fuzzy C-Means and genetic algorithms to do the clustering, showing better performance.