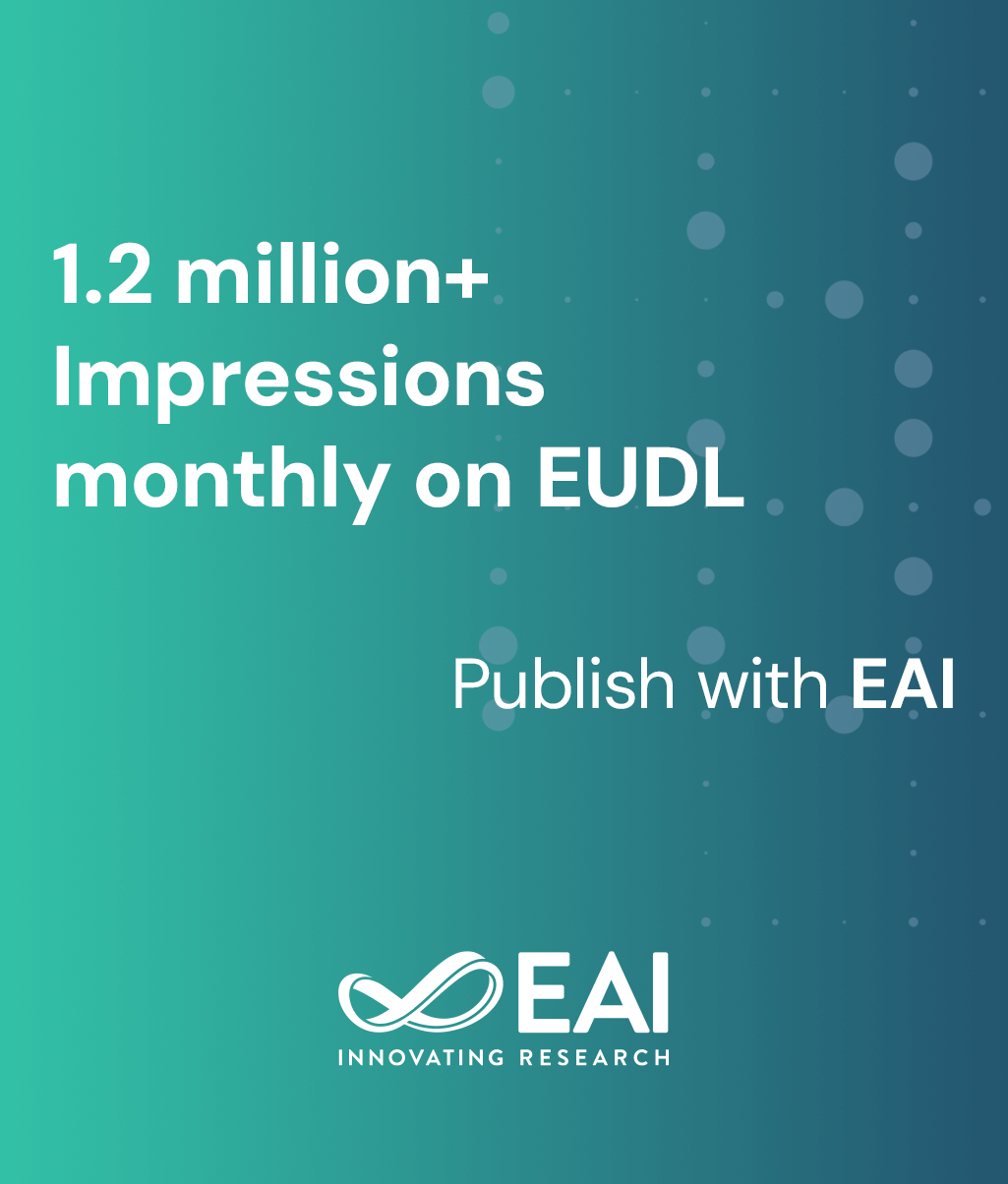
Research Article
Machine Learning Approaches for Fish Pond Water Quality Classification: Random Forest, Gaussian Naive Bayes, and Decision Tree Comparison
@INPROCEEDINGS{10.4108/eai.21-9-2023.2342964, author={Danuri Danuri and Muhammad Syafiq Mohd Pozi}, title={Machine Learning Approaches for Fish Pond Water Quality Classification: Random Forest, Gaussian Naive Bayes, and Decision Tree Comparison}, proceedings={Proceedings of the 11th International Applied Business and Engineering Conference, ABEC 2023, September 21st, 2023, Bengkalis, Riau, Indonesia}, publisher={EAI}, proceedings_a={ABEC}, year={2024}, month={2}, keywords={fish pond water quality classification machine learning}, doi={10.4108/eai.21-9-2023.2342964} }
- Danuri Danuri
Muhammad Syafiq Mohd Pozi
Year: 2024
Machine Learning Approaches for Fish Pond Water Quality Classification: Random Forest, Gaussian Naive Bayes, and Decision Tree Comparison
ABEC
EAI
DOI: 10.4108/eai.21-9-2023.2342964
Abstract
The health and production of fish in fish farms are greatly influenced by the water quality. This study examines three Machine Learning(ML) methods for categorizing fish pond water quality: Random Forest(RF), Gaussian Naive Bayes(GNB), and Decision Tree(DT). Accuracy, precision, recall, and the F1-Score as a performance indicator are taken into account while evaluating the model. The evaluation findings reveal that RF and GNB outperform DT in every evaluation criteria. GNB, with a rating of 0.958932, had the highest accuracy, followed by RF, with a value of 0.955822, and DT, with a value of 0.932269. The consistent performance of GNB and RF in precision, recall, and F1-Score underscores their superiority.
Copyright © 2023–2025 EAI