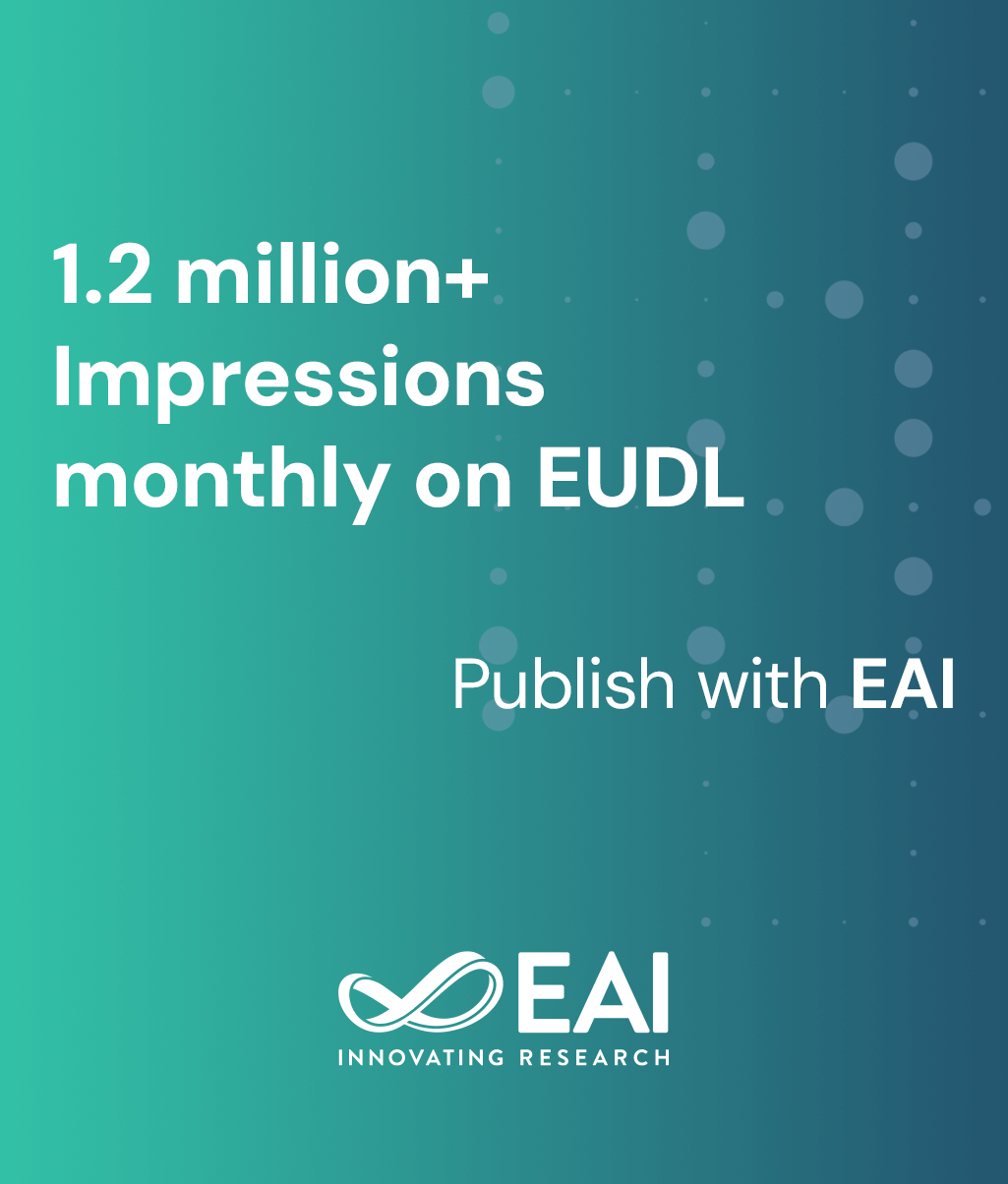
Research Article
Model Design of Intrusion Detection System on Web Server Using Machine Learning Based
@INPROCEEDINGS{10.4108/eai.21-9-2023.2342879, author={Agus Tedyyana and Osman Ghazali and Onno W. Purbo}, title={Model Design of Intrusion Detection System on Web Server Using Machine Learning Based}, proceedings={Proceedings of the 11th International Applied Business and Engineering Conference, ABEC 2023, September 21st, 2023, Bengkalis, Riau, Indonesia}, publisher={EAI}, proceedings_a={ABEC}, year={2024}, month={2}, keywords={intrusion detection systems web server machine learning feature selection anomaly detection}, doi={10.4108/eai.21-9-2023.2342879} }
- Agus Tedyyana
Osman Ghazali
Onno W. Purbo
Year: 2024
Model Design of Intrusion Detection System on Web Server Using Machine Learning Based
ABEC
EAI
DOI: 10.4108/eai.21-9-2023.2342879
Abstract
In the current era of information technology development, web server security has become a primary concern in maintaining data integrity, confidentiality, and availability. With the emergence of increasingly complex and evolving cyber threats, Intrusion Detection Systems (IDS) play a crucial role in addressing these challenges. In this research, we propose an innovative approach to enhance web server security by implementing an Intrusion Detection System empowered with Machine Learning Algorithms. In the pursuit of enhancing web server security, this research delves into designing an Intrusion Detection System (IDS) empowered by Machine Learning (ML). The foundation of this approach hinges on transparent public datasets, subjected to intensive pre-processing steps such as data cleaning, normalization, and feature selection. After refining, the data steers ML algorithms to discern potential cyber threats from standard patterns. The novel ML-based IDS showcases an ability superior to traditional systems, with the prowess to differentiate between benign and malicious activities. Comparative results accentuate the model's enhanced accuracy and a significant reduction in false alarms. This study substantiates the utility of ML in elevating cybersecurity measures and paves the way for deeper investigations into advanced web server protective mechanisms.