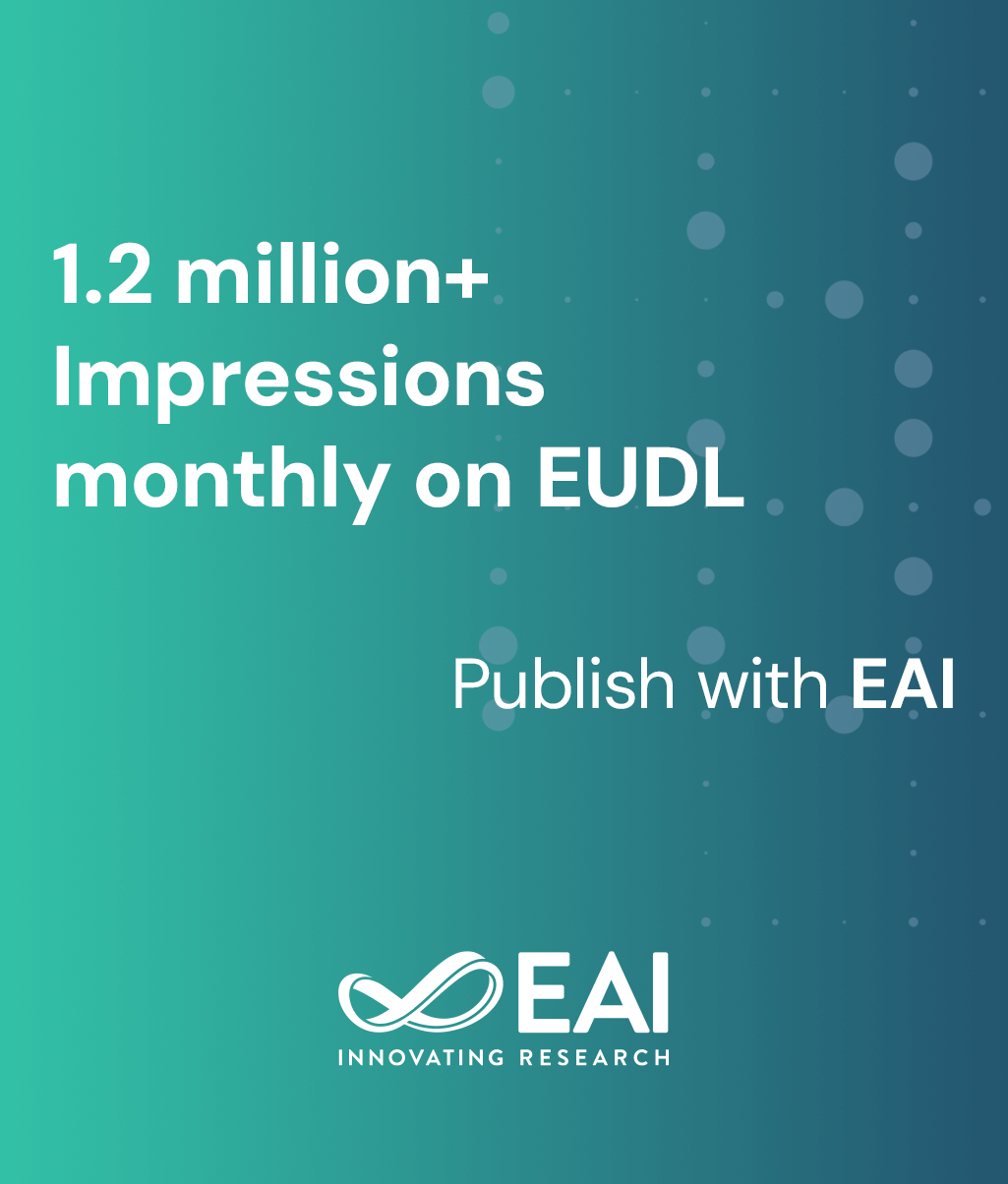
Research Article
Spatio-temporal weight Tai Chi motion feature extraction based on deep network cross-layer feature fusion
@ARTICLE{10.4108/eai.21-9-2021.170964, author={Naiqiu Wu and Yang Shi}, title={Spatio-temporal weight Tai Chi motion feature extraction based on deep network cross-layer feature fusion}, journal={EAI Endorsed Transactions on Scalable Information Systems}, volume={9}, number={34}, publisher={EAI}, journal_a={SIS}, year={2021}, month={9}, keywords={Tai Chi motion feature extraction, spatio-temporal weight, cross-layer feature fusion, deep network}, doi={10.4108/eai.21-9-2021.170964} }
- Naiqiu Wu
Yang Shi
Year: 2021
Spatio-temporal weight Tai Chi motion feature extraction based on deep network cross-layer feature fusion
SIS
EAI
DOI: 10.4108/eai.21-9-2021.170964
Abstract
Tai Chi is a valuable exercise for human health. The research on Tai Chi is helpful to improve people's exercise level. There is a problem with low efficiency in traditional Tai Chi motion feature extraction. Therefore, we propose a spatio-temporal weight Tai Chi motion feature extraction based on deep network cross-layer feature fusion. According to the selected motion spatio-temporal sample, the corresponding spatio-temporal motion key frame is extracted and output in the form of static image. The initial motion image is preprocessed by motion object detection and image enhancement. Traditional convolutional neural network extracts features from the shallow to the deep and builds a classifier for image classification, which is easy to ignore the shallow features. Based on the AlexNet network, a CL-AlexNet network is proposed. Batch normalization (BN) is used for data normalization. The cross-connection structure is introduced and the sensitivity analysis is performed. The Inception module is embedded for multi-scale depth feature extraction. It integrates deep features and shallow features. The spatio-temporal weight adaptive interpolation method is used to reduce the error of edge detection. From the edge features and the motion spatio-temporal features, it realizes motion features extraction, and outputs the extraction results. Compared with the state-of-the-art feature extraction algorithms, the experiment results show that the proposed algorithm can extract more effective features. The recognition rate exceeds 90%. It can be used as guidance and evidence for Tai Chi training.
Copyright © 2021 Naiqiu Wu et al., licensed to EAI. This is an open access article distributed under the terms of the Creative Commons Attribution license, which permits unlimited use, distribution and reproduction in any medium so long as the original work is properly cited.