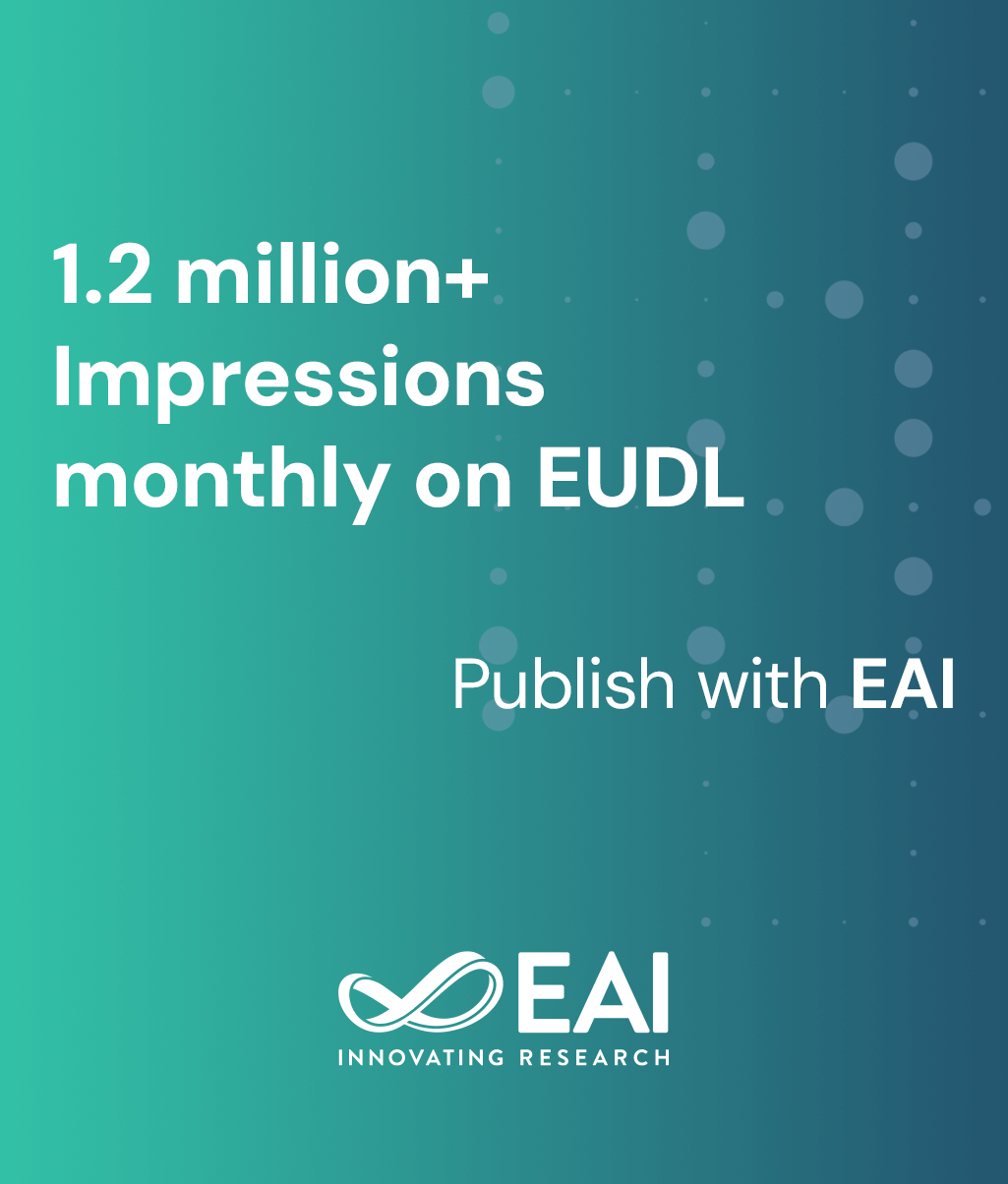
Research Article
A Combining Model for Crowd Flow Prediction in Partition Coverage Wireless Network Scenarios
@INPROCEEDINGS{10.4108/eai.21-11-2024.2354639, author={Zheng Ma and Rui Zhang and Lang Gao}, title={A Combining Model for Crowd Flow Prediction in Partition Coverage Wireless Network Scenarios}, proceedings={Proceedings of the 2nd International Conference on Machine Learning and Automation, CONF-MLA 2024, November 21, 2024, Adana, Turkey}, publisher={EAI}, proceedings_a={CONF-MLA}, year={2025}, month={3}, keywords={wireless network crowd prediction arima cnn-lstm}, doi={10.4108/eai.21-11-2024.2354639} }
- Zheng Ma
Rui Zhang
Lang Gao
Year: 2025
A Combining Model for Crowd Flow Prediction in Partition Coverage Wireless Network Scenarios
CONF-MLA
EAI
DOI: 10.4108/eai.21-11-2024.2354639
Abstract
With the development of modern higher education, university campuses serve multiple functions for both faculty and students, including teaching, research, and daily life. These campuses commonly face issues such as high population density, frequent mobility, and complex composition. Accurate prediction of campus traffic environments is fundamental for scientific and efficient management. Predictive data can support various aspects of campus operations, including energy conservation, resource allocation, event scheduling, hazard prevention, and emergency response, making it of significant importance. This paper leverages the advantages of wireless network seamless roaming technology to obtain the number of people in different areas from wireless controllers. Based on this, we propose a crowd flow prediction model using ARIMA-CNN-LSTM. The model has been tested, and its accuracy meets expectations and surpasses traditional algorithms.