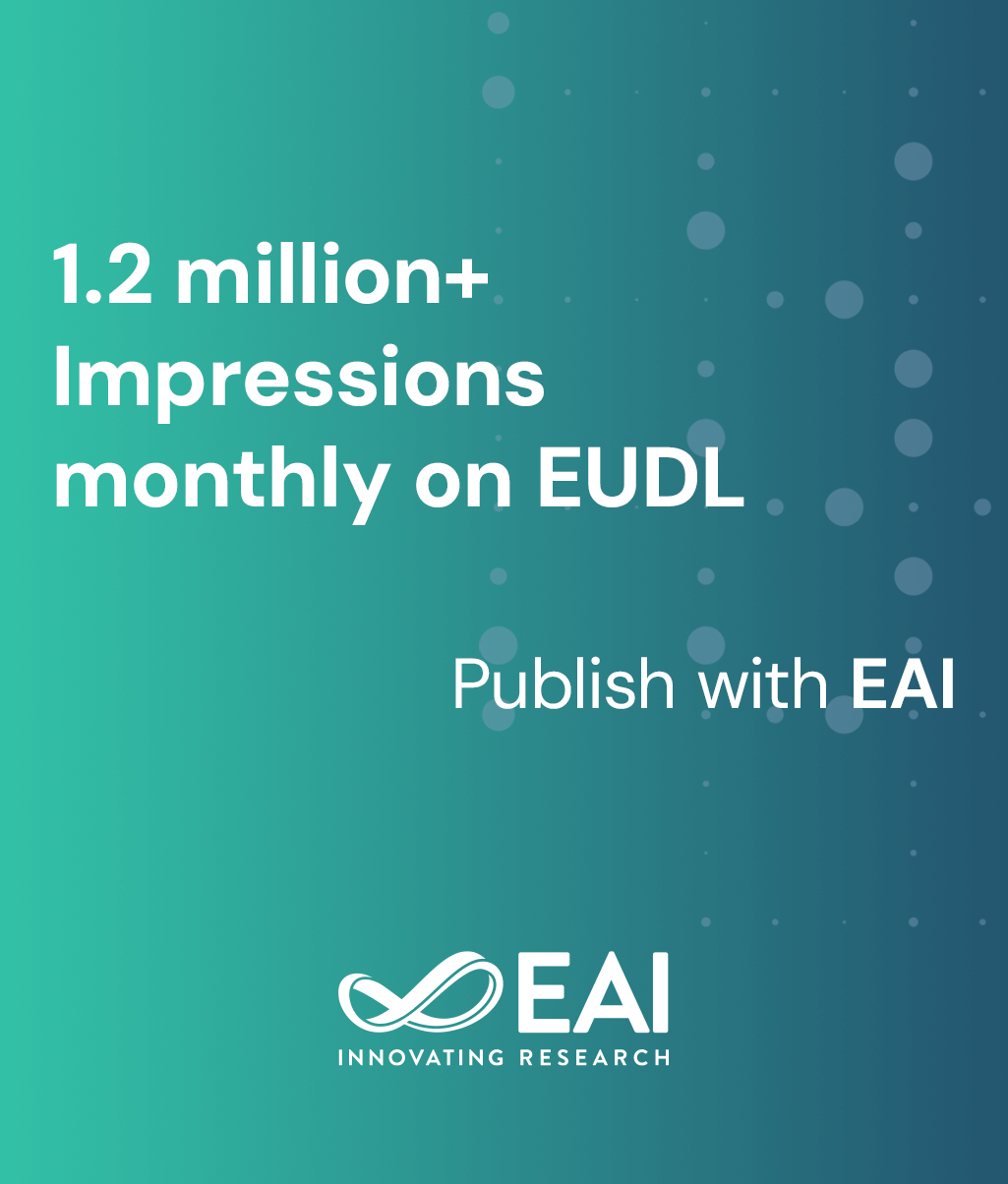
Research Article
Clustering Time Series Data Considering Both Trend Filtering and Subject Level Closeness
@INPROCEEDINGS{10.4108/eai.21-11-2024.2354637, author={Zhiren Wang}, title={Clustering Time Series Data Considering Both Trend Filtering and Subject Level Closeness}, proceedings={Proceedings of the 2nd International Conference on Machine Learning and Automation, CONF-MLA 2024, November 21, 2024, Adana, Turkey}, publisher={EAI}, proceedings_a={CONF-MLA}, year={2025}, month={3}, keywords={clustering methodology time series analysis medoid-based optimization lung cancer}, doi={10.4108/eai.21-11-2024.2354637} }
- Zhiren Wang
Year: 2025
Clustering Time Series Data Considering Both Trend Filtering and Subject Level Closeness
CONF-MLA
EAI
DOI: 10.4108/eai.21-11-2024.2354637
Abstract
This paper presents a novel clustering methodology that integrates both longitudinal trends and multi-dimensional proximity of lung cancer rates across 50 U.S. states and Washington D.C. from 1969 to 2019. By synthesizing temporal dynamics and local similarities, the proposed hybrid algorithm refines traditional clustering techniques to offer a comprehensive understanding of state-level lung cancer trends. The methodology combines K-means clustering with a medoid-based optimization approach, capturing the evolving patterns in lung cancer rates while mitigating the impact of outliers. Results reveal distinct clusters that reflect shared historical trajectories in health outcomes, outperforming traditional methods such as K-means, Radial Basis Function (RBF) networks, and Support Vector Machines (SVM) in sensitivity to temporal variations. The findings provide insights into regional health behaviors and interventions, highlighting the significance of integrating time-series analysis with clustering frameworks in public health research.