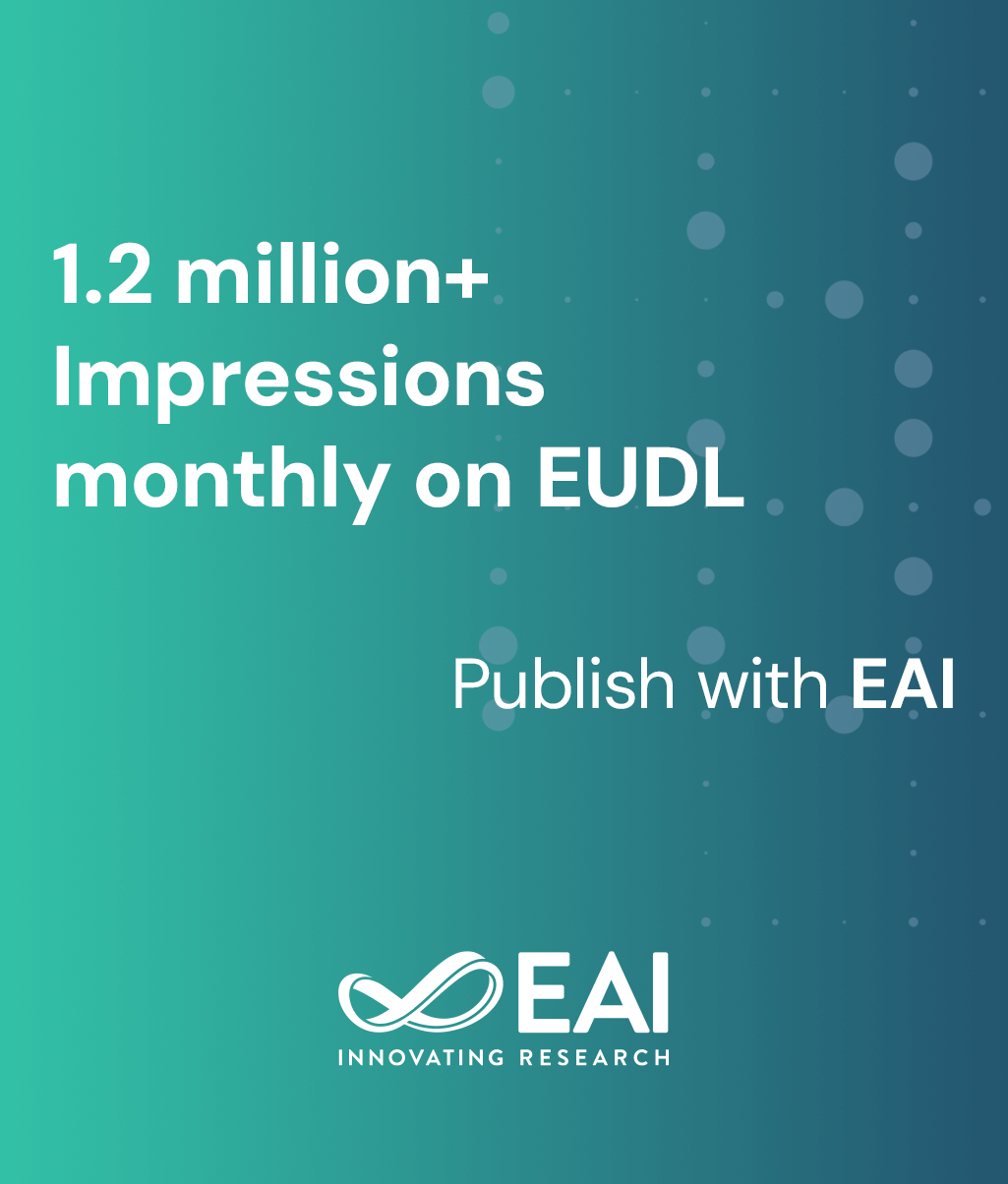
Research Article
Enhancing Movie Recommendation Systems with Hybrid Collaborative Filtering, Content-based Filtering and SVD
@INPROCEEDINGS{10.4108/eai.21-11-2024.2354625, author={Liheng Xu and Zhile Guan and Yu Wu}, title={Enhancing Movie Recommendation Systems with Hybrid Collaborative Filtering, Content-based Filtering and SVD}, proceedings={Proceedings of the 2nd International Conference on Machine Learning and Automation, CONF-MLA 2024, November 21, 2024, Adana, Turkey}, publisher={EAI}, proceedings_a={CONF-MLA}, year={2025}, month={3}, keywords={movie recommendation hybrid filtering algorithm collaborative filtering content-based svd}, doi={10.4108/eai.21-11-2024.2354625} }
- Liheng Xu
Zhile Guan
Yu Wu
Year: 2025
Enhancing Movie Recommendation Systems with Hybrid Collaborative Filtering, Content-based Filtering and SVD
CONF-MLA
EAI
DOI: 10.4108/eai.21-11-2024.2354625
Abstract
The swift advancement of Internet technology has intensified the issue of information overload. Consequently, users face difficulties in efficiently navigating extensive data and locating content tailored to their interests. To handle this issue, this paper proposes an enhanced movie recommendation system that leverages a hybrid approach combining collaborative filtering, content-based filtering, and Singular Value Decomposition (SVD). By analyzing the MovieLens dataset, we identify critical features and develop hybrid models that aim to improve upon existing methods by harnessing the strengths of each filtering technique. Our hybrid methods seek to bypass the constraints of individual filters, enhancing prediction accuracy and recommendation relevance. The experiments demonstrated that combining these techniques significantly reduces Root Mean Square Error (RMSE) and Mean Absolute Error (MAE), indicating improved performance. Our findings show that hybrid approaches improve movie recommendation systems. They offer more personalized and accurate recommendations. However, there may be limitations to these methods. Therefore, future research should investigate these limitations and work on refining the techniques. The goal is to achieve even better performance.