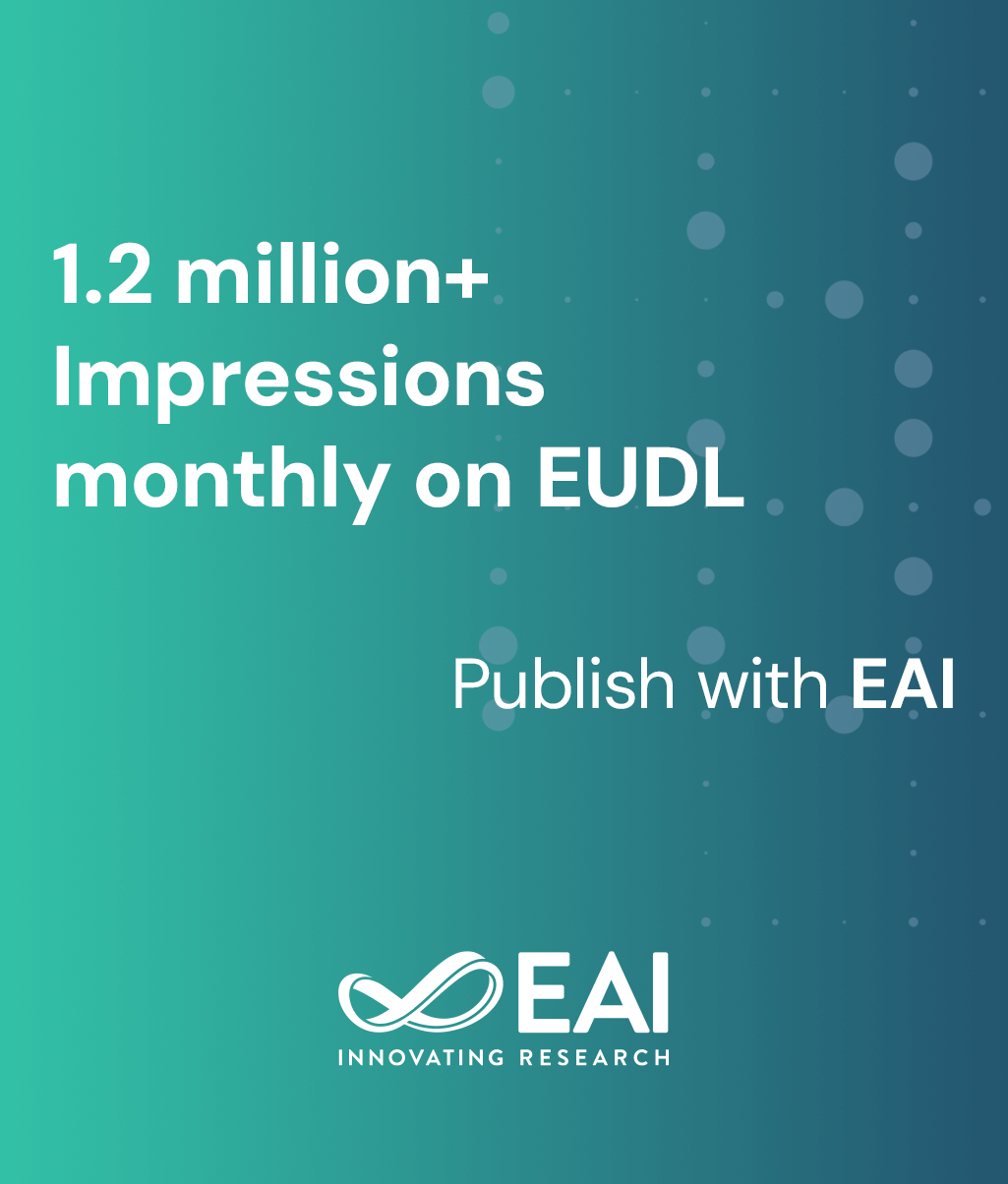
Research Article
A Non-Vector Retrieval-Augmented Generation Model for External Time-Relevant Corpus Extraction
@INPROCEEDINGS{10.4108/eai.21-11-2024.2354622, author={Jinghao You}, title={A Non-Vector Retrieval-Augmented Generation Model for External Time-Relevant Corpus Extraction}, proceedings={Proceedings of the 2nd International Conference on Machine Learning and Automation, CONF-MLA 2024, November 21, 2024, Adana, Turkey}, publisher={EAI}, proceedings_a={CONF-MLA}, year={2025}, month={3}, keywords={llm rag test-to-sql time-relevant information extraction}, doi={10.4108/eai.21-11-2024.2354622} }
- Jinghao You
Year: 2025
A Non-Vector Retrieval-Augmented Generation Model for External Time-Relevant Corpus Extraction
CONF-MLA
EAI
DOI: 10.4108/eai.21-11-2024.2354622
Abstract
Large language models (LLMs) have emerged as a powerful tool in the natural language process, allowing information extraction and answer generation from pre-trained databases. Regarding untrained external corpus, the retrieval-augmented generation (RAG) techniques enable quick establishing of databases. However, this process is currently of low accuracy when handling problems with strong indexing correlations, such as time-relevant input. This paper proposes a non-vector retrieval-augmented generation (NVRAG) model to enhance the RAG model for processing multi-index related complex queries based on a non-vector database and text-to-SQL technology. NVRAG stores relevant parameters in a non-vector database to narrow the embedding range and improve indexing accuracy. Experiments on a weather briefings database are conducted to validate the effectiveness of the NVRAG model. The results show that compared with the original RAG, the faithfulness and accuracy rate of NVRAG are higher, thereby sacrificing the response time. By implementing this approach, the system's ability to handle complex query requests is enhanced.