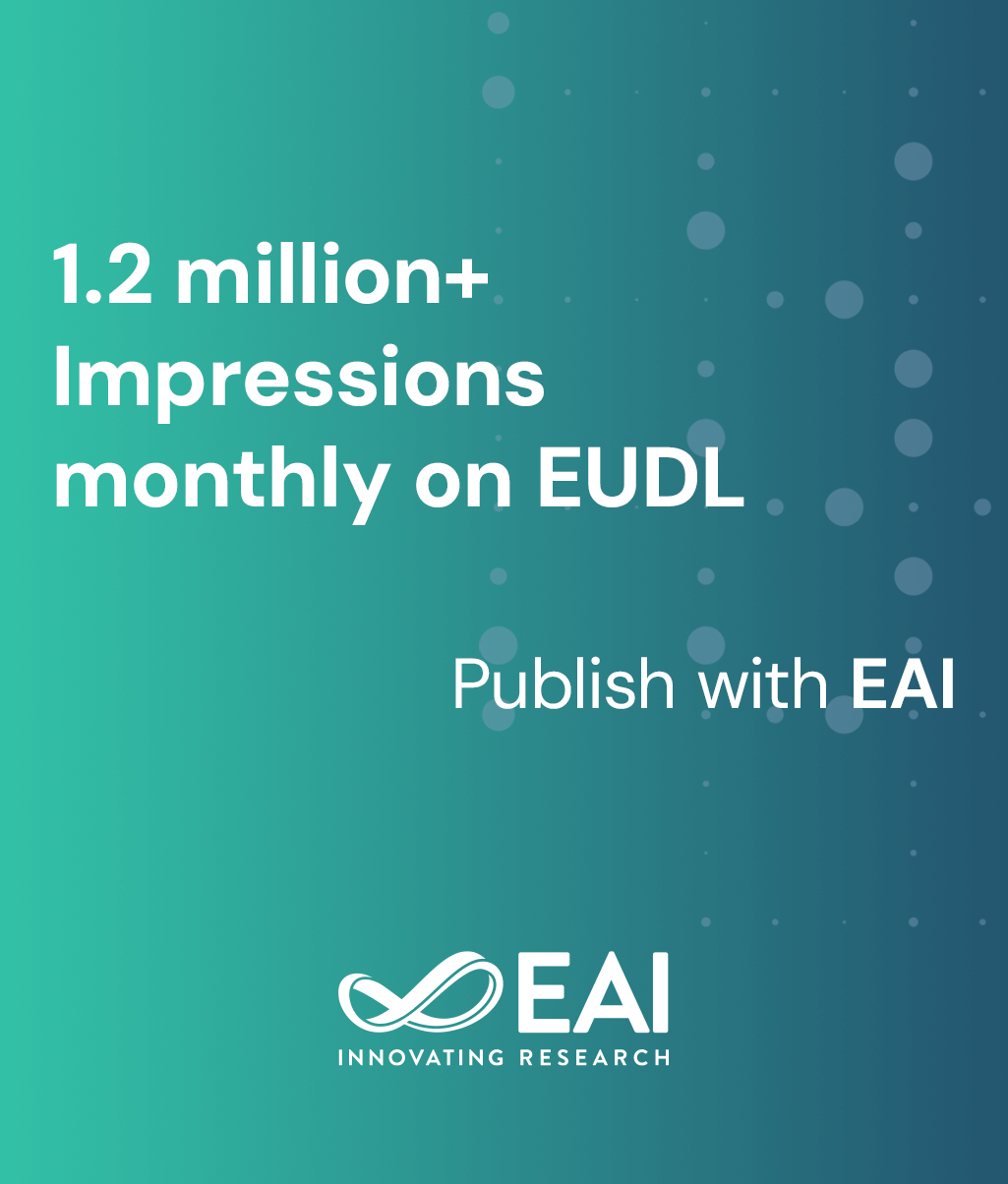
Research Article
Object Detection Using AutoML and YOLO: A Comparative Analysis of EasyDL, YOLOv8, and YOLOv10
@INPROCEEDINGS{10.4108/eai.21-11-2024.2354621, author={Jiaxiang Chen}, title={Object Detection Using AutoML and YOLO: A Comparative Analysis of EasyDL, YOLOv8, and YOLOv10}, proceedings={Proceedings of the 2nd International Conference on Machine Learning and Automation, CONF-MLA 2024, November 21, 2024, Adana, Turkey}, publisher={EAI}, proceedings_a={CONF-MLA}, year={2025}, month={3}, keywords={automl easydl yolov8 yolov10 object detection}, doi={10.4108/eai.21-11-2024.2354621} }
- Jiaxiang Chen
Year: 2025
Object Detection Using AutoML and YOLO: A Comparative Analysis of EasyDL, YOLOv8, and YOLOv10
CONF-MLA
EAI
DOI: 10.4108/eai.21-11-2024.2354621
Abstract
Object detection technology has been applied in multiple fields, and compared to traditional methods, using AutoML to complete object detection tasks may be more convenient and less labor-intensive. This study focuses on the performance of object detection models, comparing the AutoML platform EasyDL with the popular object detection models YOLOv8 and YOLOv10. The experiments were conducted on two datasets: mask detection and slightly larger traffic sign detection. The study compares certain performance metrics of the models and demonstrates the ease or difficulty of completing tasks. The results indicate that EasyDL provides a user-friendly and simplified workflow, allowing users without technical expertise to operate it; however, due to inherent platform limitations, its training time is relatively long. Additionally, on larger datasets, the YOLO models outperform EasyDL in terms of both accuracy and speed. Nevertheless, on smaller datasets, the performance of EasyDL is very close to that of YOLOv8 and YOLOv10. Despite the longer training time, EasyDL delivers competitive results, indicating the feasibility of AutoML in object detection tasks. This study innovatively conducts a comparative analysis of the performance dimensions of AutoML and YOLO models across different task scales, providing new insights for practitioners and non-professionals seeking efficient and accessible solutions for object detection tasks.