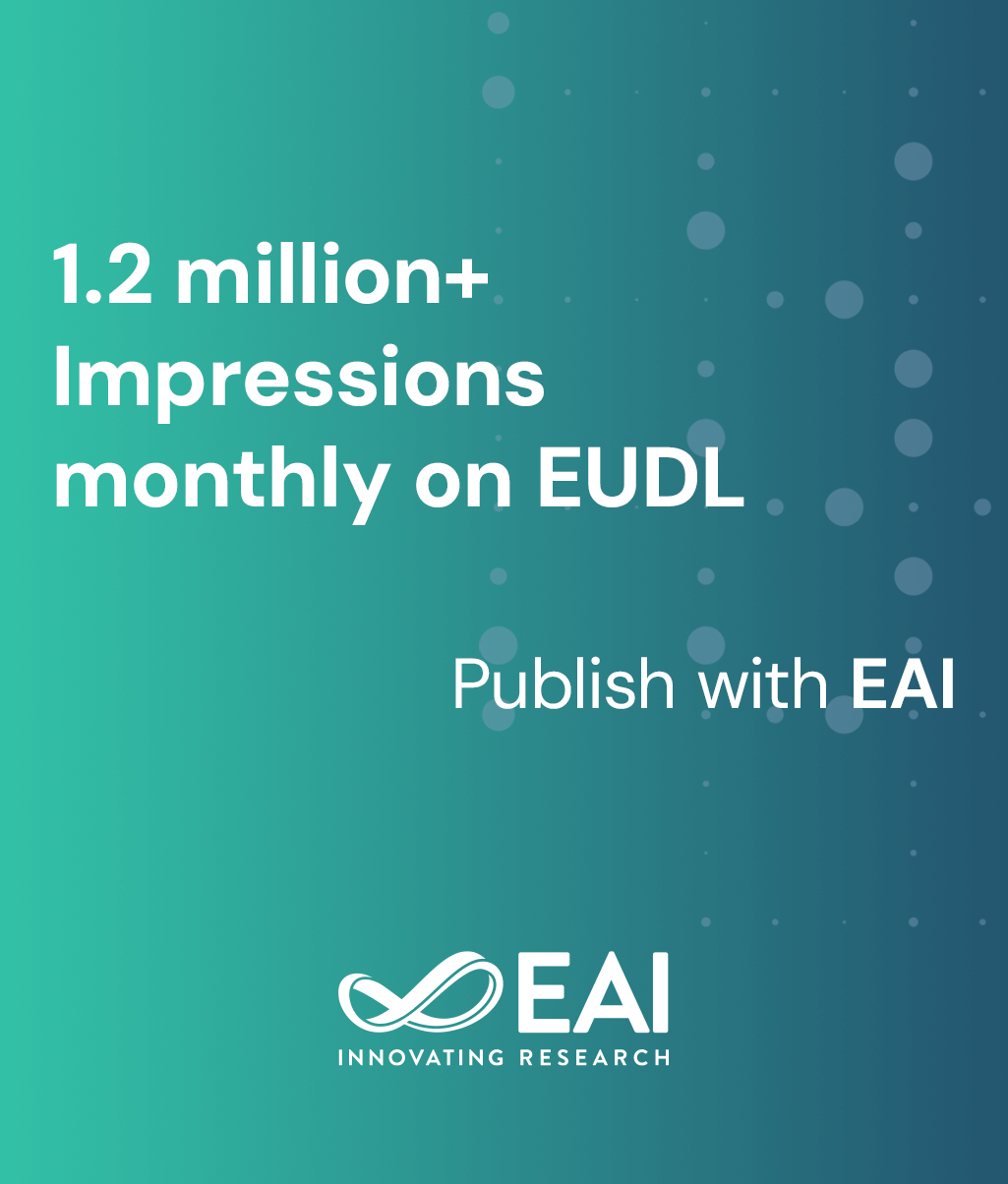
Research Article
Diffusion Based Data Augmentation for Face Anti-spoofing
@INPROCEEDINGS{10.4108/eai.21-11-2024.2354616, author={Tianheng Zhang}, title={Diffusion Based Data Augmentation for Face Anti-spoofing}, proceedings={Proceedings of the 2nd International Conference on Machine Learning and Automation, CONF-MLA 2024, November 21, 2024, Adana, Turkey}, publisher={EAI}, proceedings_a={CONF-MLA}, year={2025}, month={3}, keywords={diffusion model computer vision face anti-spoofing unet self-attention}, doi={10.4108/eai.21-11-2024.2354616} }
- Tianheng Zhang
Year: 2025
Diffusion Based Data Augmentation for Face Anti-spoofing
CONF-MLA
EAI
DOI: 10.4108/eai.21-11-2024.2354616
Abstract
As the internet continues to evolve, the application of facial recognition technology across different sectors ranging from unlocking smartphones to enhancing airport security and facilitating financial transactions is on the rise. Consequently, the necessity for robust face anti-spoofing (FAS) technology is becoming ever more critical. This article presents a new FAS method that enhances the security of face recognition systems through the optimization of the diffusion model and neural network structure. The diffusion model is used to generate spoof face data similar to the real face, which enriches the original dataset and enhances the robustness of the model. Furthermore, the UNet network structure is enhanced by incorporating the Attention mechanism and optimizing the loss function through a comprehensive consideration of SSIM, Cross-Entropy, and MSE. These modifications aim to improve the ability to distinguish between authentic and fraudulent faces. Experimental results demonstrate that our proposed approach surpasses existing technologies in testing scenarios, showcasing its potential in the field of face anti-spoofing.