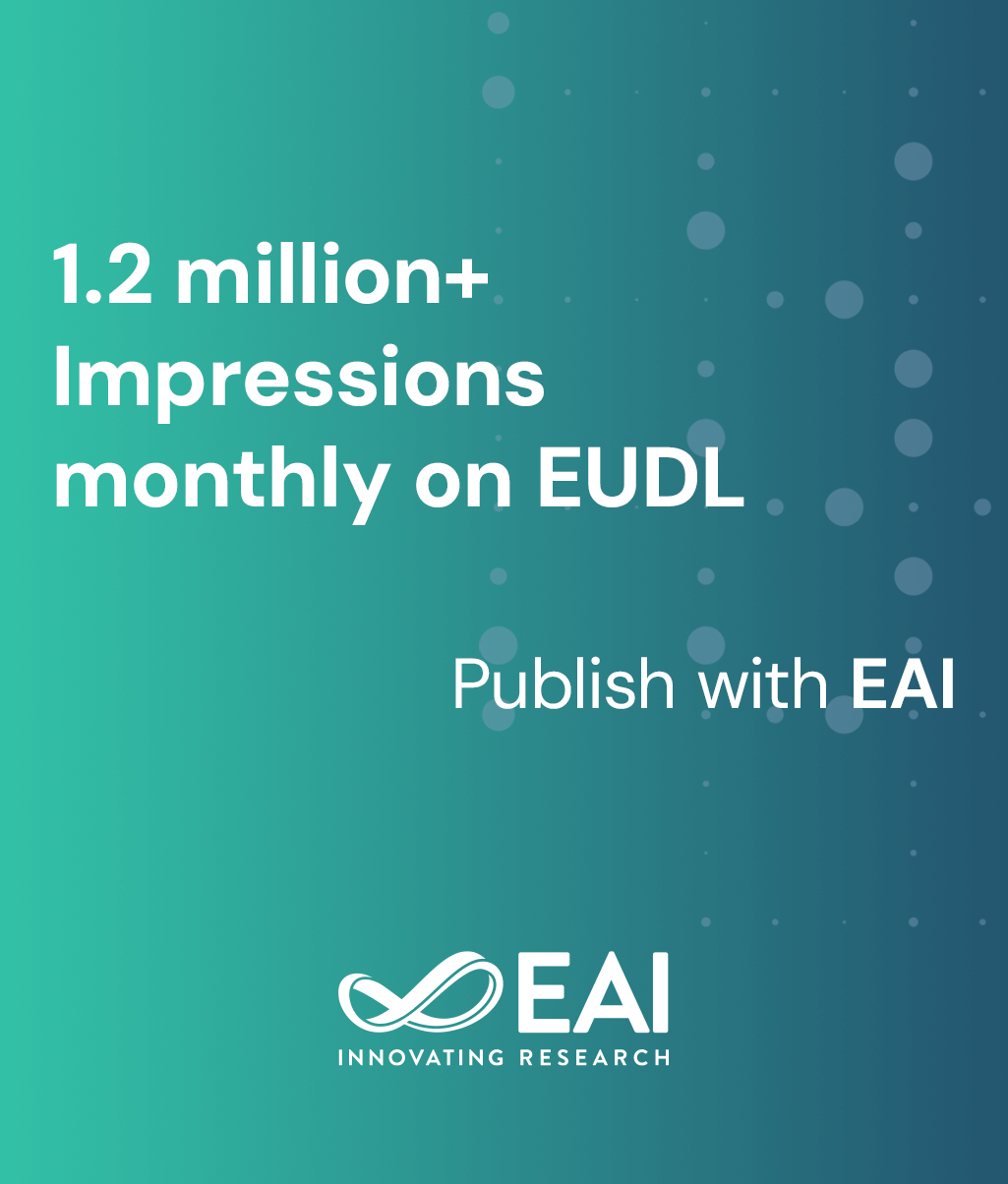
Research Article
A Study on Image Recognition of Chinese Herbal Medicine Based on Probabilistic Convolution Voting Model
@INPROCEEDINGS{10.4108/eai.21-11-2024.2354590, author={Yiheng Yan and Ziting Gao}, title={A Study on Image Recognition of Chinese Herbal Medicine Based on Probabilistic Convolution Voting Model}, proceedings={Proceedings of the 2nd International Conference on Machine Learning and Automation, CONF-MLA 2024, November 21, 2024, Adana, Turkey}, publisher={EAI}, proceedings_a={CONF-MLA}, year={2025}, month={3}, keywords={ensemble learning chinese herbal medicine deep learning image recognition probabilistic convolution voting model convolutional neural networks vision transformers}, doi={10.4108/eai.21-11-2024.2354590} }
- Yiheng Yan
Ziting Gao
Year: 2025
A Study on Image Recognition of Chinese Herbal Medicine Based on Probabilistic Convolution Voting Model
CONF-MLA
EAI
DOI: 10.4108/eai.21-11-2024.2354590
Abstract
This study investigates the application of deep learning in the field of Chinese herbal medicine (CHM) image recognition, proposing a novel multi-model ensemble approach called the Probabilistic Convolution Voting Model (PCVM) to address the limitations of current single-model systems. Traditional Chinese Medicine (TCM), with its roots in ancient Daoist practices, has gained global relevance, particularly during the COVID-19 pandemic, for its efficacy and minimal side effects. However, CHM identification faces challenges such as inefficiency and error-proneness in traditional identification methods. We leverage advances in convolutional neural networks (CNNs) and Vision Transformers (ViTs) to enhance image recognition tasks essential for CHM identification. The proposed PCVM integrates multiple pre-trained models to improve accuracy and robustness, focusing particularly on enhancing recognition capabilities in visually complex categories. The ensemble method uses a novel convolutional and voting layer system to reduce misclassification and optimize decision-making. Comprehensive experiments demonstrate that PCVM significantly outperforms traditional methods, particularly in challenging categories, making it a promising solution not only for CHM but potentially for other high-precision medical image recognition tasks such as cancer detection. Future work will focus on further optimizing and expanding the PCVM framework to enhance computational efficiency and applicability.