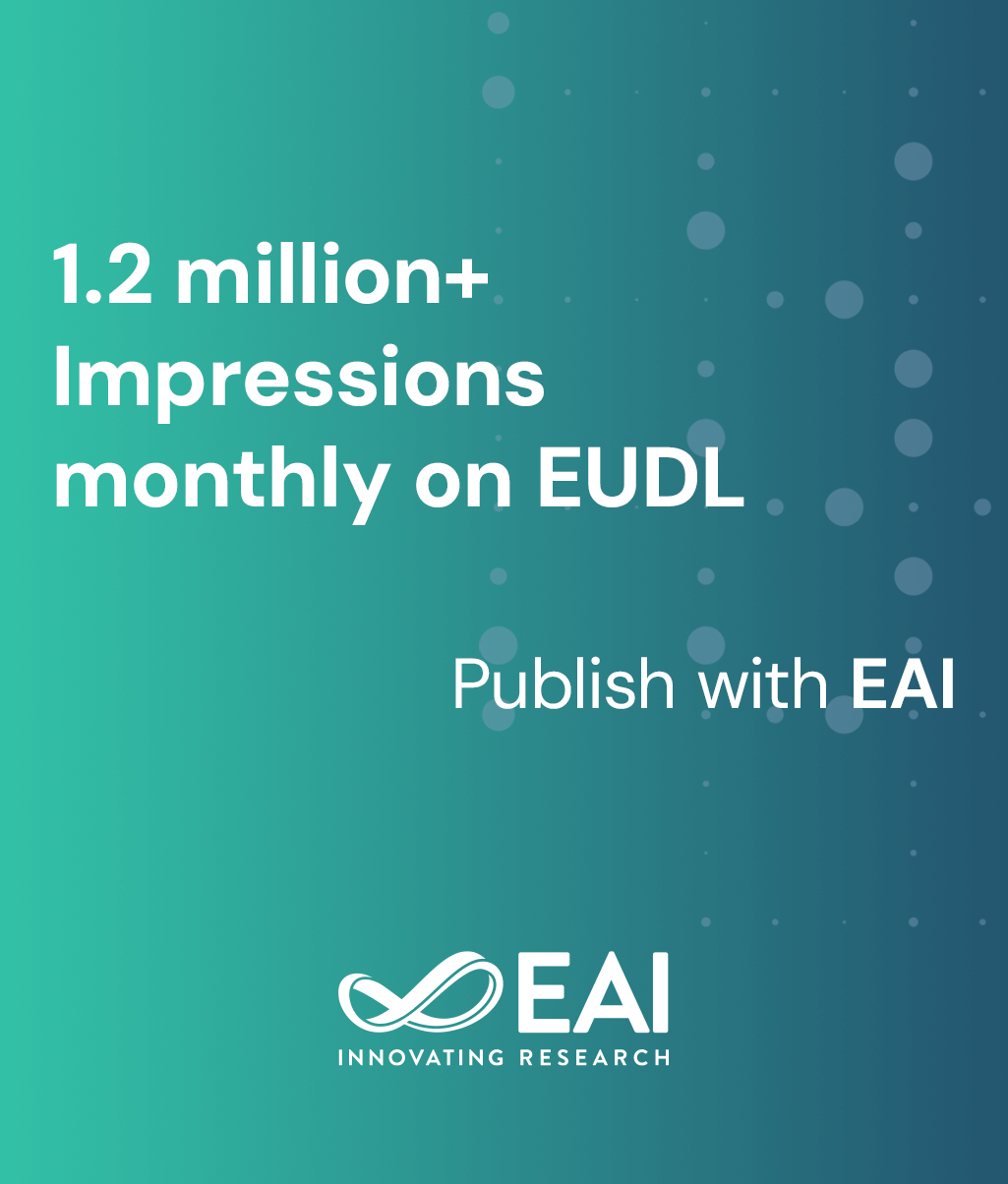
Research Article
Low-Light Image Enhancement Based on Retinex Theory and Attention Mechanism
@INPROCEEDINGS{10.4108/eai.21-11-2024.2354585, author={Linlin Jiao and Fang Zhang}, title={Low-Light Image Enhancement Based on Retinex Theory and Attention Mechanism}, proceedings={Proceedings of the 2nd International Conference on Machine Learning and Automation, CONF-MLA 2024, November 21, 2024, Adana, Turkey}, publisher={EAI}, proceedings_a={CONF-MLA}, year={2025}, month={3}, keywords={low-light retinex attention tvloss}, doi={10.4108/eai.21-11-2024.2354585} }
- Linlin Jiao
Fang Zhang
Year: 2025
Low-Light Image Enhancement Based on Retinex Theory and Attention Mechanism
CONF-MLA
EAI
DOI: 10.4108/eai.21-11-2024.2354585
Abstract
With the popularity of mobile devices and surveillance cameras, image enhancement under low-light conditions has become an crucial research direction in computer vision. Although existing low-light enhancement techniques have made some progress, they still have limitations in processing complex scenes and maintaining image details. To handle these challenges, this paper introduces an approach on account of an improved Retinex-Net and attention mechanism. Our approach combines the retinex theory with Squeeze-and-Excitation Networks (SENet) and the total variance (tvloss) loss function to enhance the quality of low-light images. Comparative experiments were conducted on the LOL dataset, and the results of the experiments confirm that our proposed improved model provides significant improvements in peak signal-to-noise ratio (PSNR), structural similarity index (SSIM), and subjective visual performance compared to the original Retinex-Net model. Ablation experiments were executed to analyze the role of each module in the proposed method individually. The empirical evidence supports the functionality of each module within the algorithm and the advancement of the overall method.