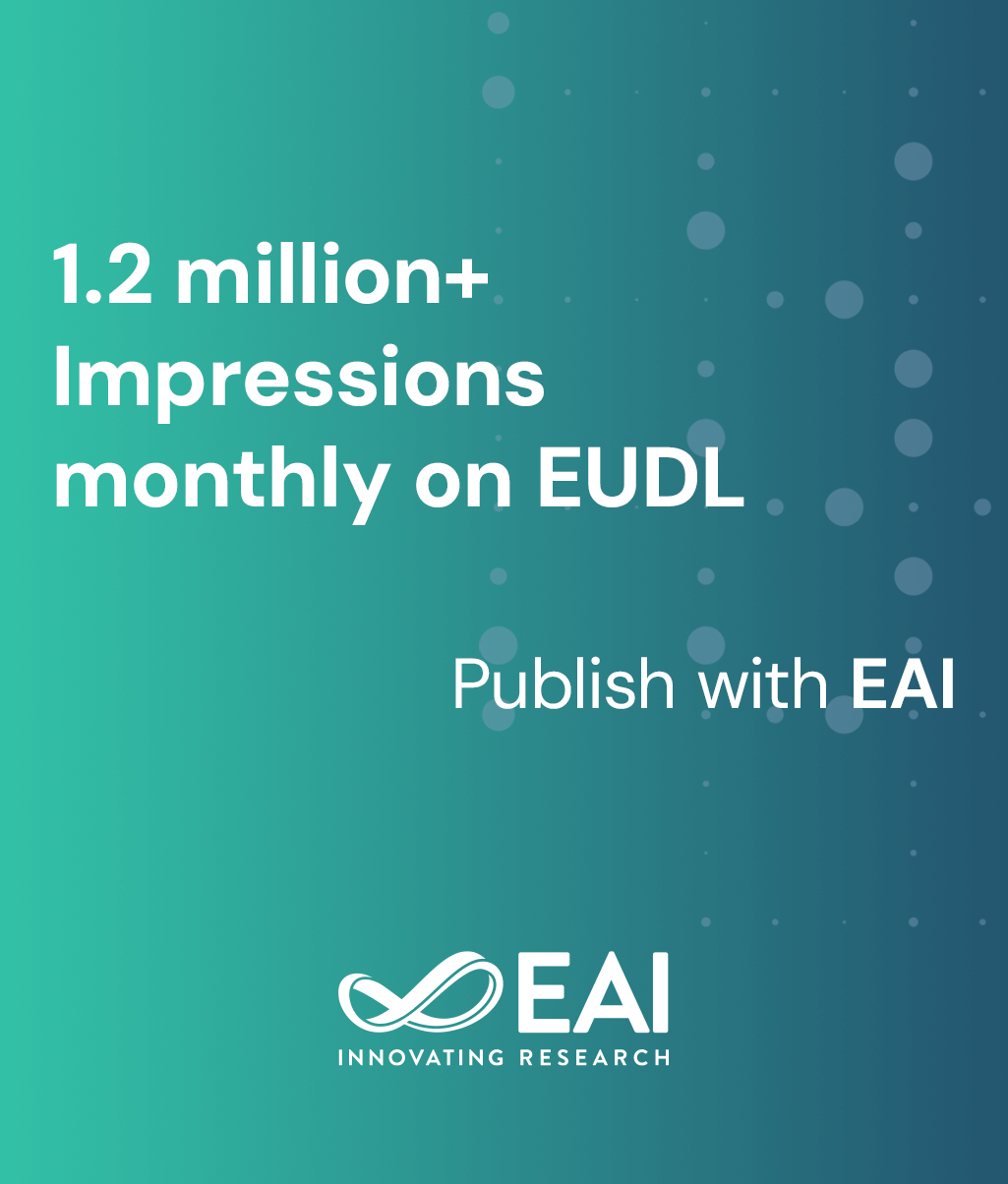
Research Article
On Identifying Facial Expression using Multiclass Ensemble Least-Squares Support Vector Machine
@INPROCEEDINGS{10.4108/eai.20-1-2018.2281939, author={M. Sya'rani and Armin Lawi and Rayuwati Rayuwati and Mursalin Mursalin}, title={On Identifying Facial Expression using Multiclass Ensemble Least-Squares Support Vector Machine}, proceedings={Proceedings of the 1st Workshop on Multidisciplinary and Its Applications Part 1, WMA-01 2018, 19-20 January 2018, Aceh, Indonesia}, publisher={EAI}, proceedings_a={WMA-1}, year={2019}, month={9}, keywords={the facial expression multiclass ensemble least-squares vector machine}, doi={10.4108/eai.20-1-2018.2281939} }
- M. Sya'rani
Armin Lawi
Rayuwati Rayuwati
Mursalin Mursalin
Year: 2019
On Identifying Facial Expression using Multiclass Ensemble Least-Squares Support Vector Machine
WMA-1
EAI
DOI: 10.4108/eai.20-1-2018.2281939
Abstract
Facial expression is one of behavior characteristics of human-being. The use of biometrics technology system with facial expression characteristics makes it possible to recognize a person's mood or emotion. The basic components of facial expression analysis system are face detection, face image extraction, facial classification and facial expressions recognition. This paper uses Linear Discriminant Analysis (LDA) algorithm to extract facial features with expression parameters, i.e., happy, sad, neutral, angry, fear, and disgusted. Then Multiclass Ensemble Least-Squares Support Vector Machine (MELS-SVM) is used for the classification process of facial expression. The result of MELS-SVM model obtained from our 185 different expression images of 10 persons showed high accuracy level of 99.998% using RBF kernel.