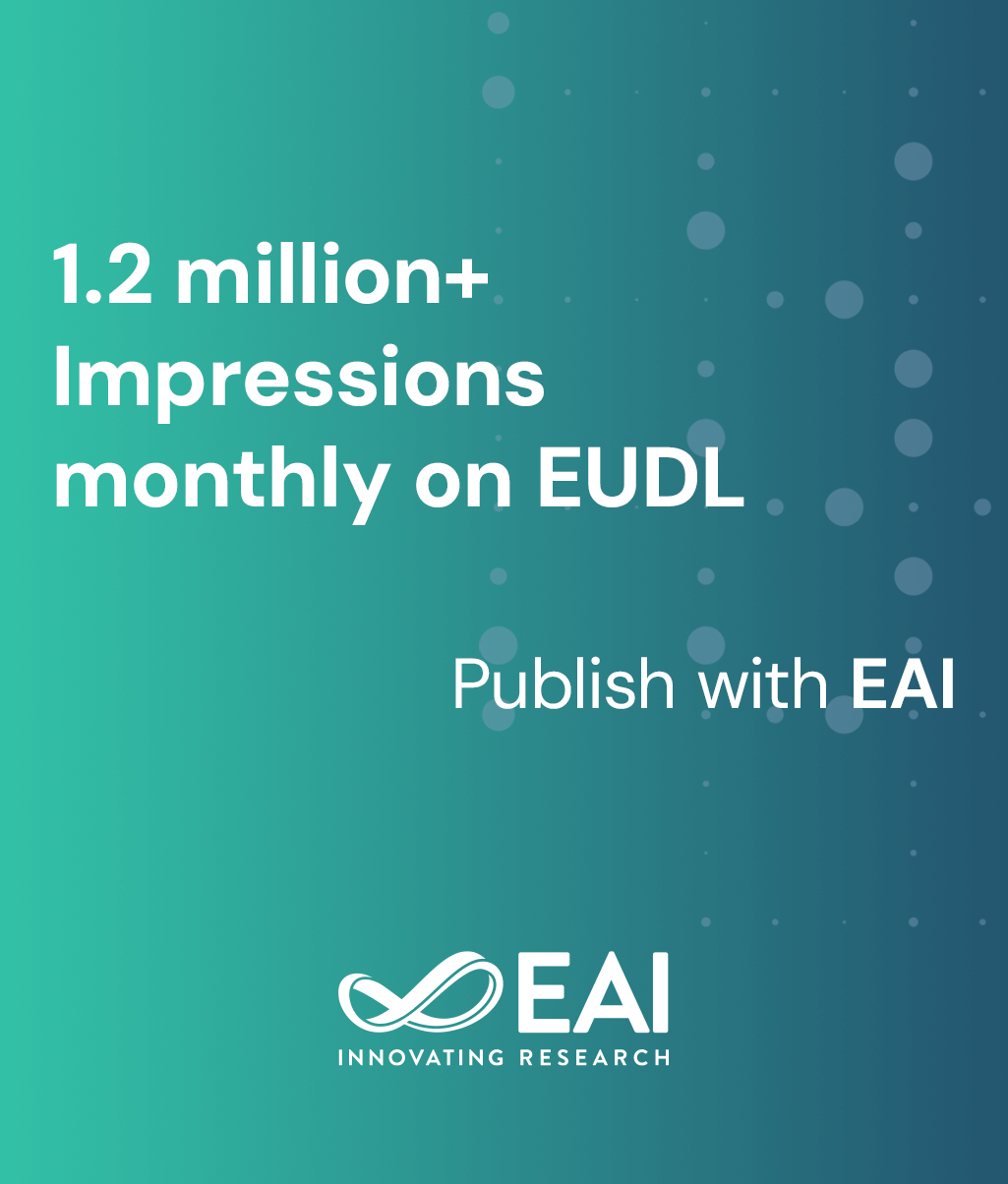
Research Article
Cocoa Beans Digital Image Classification Based On Color Features using Multiclass Ensemble Least-Squares Support Vector Machine
@INPROCEEDINGS{10.4108/eai.20-1-2018.2281929, author={Yudhi Adhitya and Armin Lawi and Hartono Hartono and Mursalin Mursalin}, title={Cocoa Beans Digital Image Classification Based On Color Features using Multiclass Ensemble Least-Squares Support Vector Machine}, proceedings={Proceedings of the 1st Workshop on Multidisciplinary and Its Applications Part 1, WMA-01 2018, 19-20 January 2018, Aceh, Indonesia}, publisher={EAI}, proceedings_a={WMA-1}, year={2019}, month={9}, keywords={the cocoa beans digital image multiclass ensemble least-squares vector machine}, doi={10.4108/eai.20-1-2018.2281929} }
- Yudhi Adhitya
Armin Lawi
Hartono Hartono
Mursalin Mursalin
Year: 2019
Cocoa Beans Digital Image Classification Based On Color Features using Multiclass Ensemble Least-Squares Support Vector Machine
WMA-1
EAI
DOI: 10.4108/eai.20-1-2018.2281929
Abstract
This research aims to determine the quality of cocoa beans through their fermentation status of their digital images. Samples of cocoa beans were scattered on a bright white paper under a controlled lighting condition. A compact digital camera was used to capture the images. The images were then processed to extract their color parameters. Classification process begins with an analysis of cocoa beans image based on color feature extraction. Parameters of visual classification of cocoa beans were obtained by extraction of color feature parameters, i.e.: Red (R), Green (G), Blue (B), Hue (H), Saturation (S) and Value (V). Then the beans are classified into 3 classes, i.e., Fermented Beans, Un-Fermented Beans and Moldy Beans. The classification process using the Multiclass Ensemble Least-Squares Support Vector Machine (MELS-SVM) method starts with the training process to get the model, and then the model is used in the testing process to get accuracy. The classification model of input parameters from our 1,604 cocoa beans images based on the color features obtained accuracy of 99.281%.