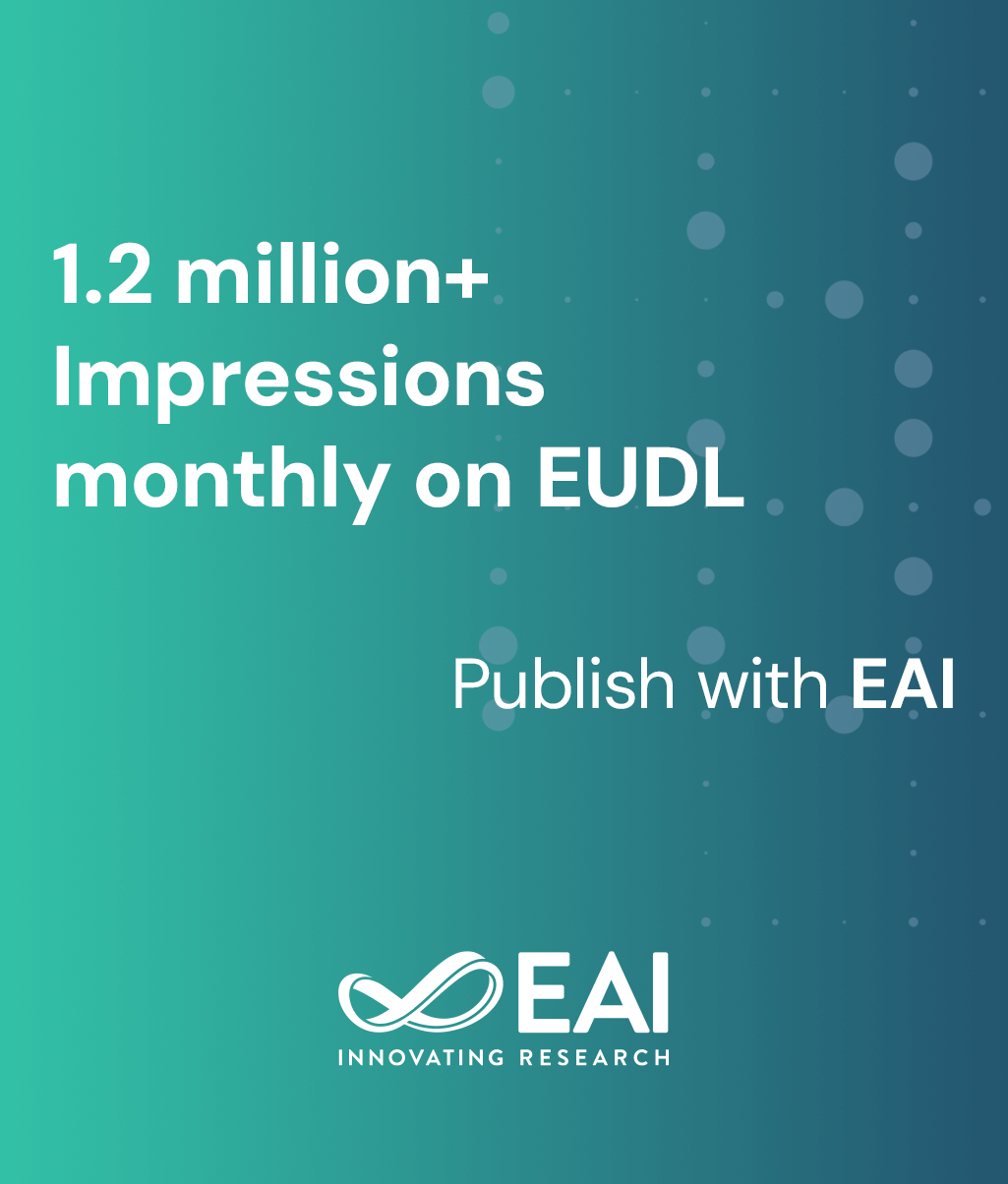
Research Article
Identifying Irregularities Usage of Smart Electric Voucher using Support Vector Machines
@INPROCEEDINGS{10.4108/eai.20-1-2018.2281928, author={Supriyadi La Wungo and Armin Lawi and Hartono Hartono}, title={Identifying Irregularities Usage of Smart Electric Voucher using Support Vector Machines}, proceedings={Proceedings of the 1st Workshop on Multidisciplinary and Its Applications Part 1, WMA-01 2018, 19-20 January 2018, Aceh, Indonesia}, publisher={EAI}, proceedings_a={WMA-1}, year={2019}, month={9}, keywords={the irregularities usage smart electric voucher vector machine}, doi={10.4108/eai.20-1-2018.2281928} }
- Supriyadi La Wungo
Armin Lawi
Hartono Hartono
Year: 2019
Identifying Irregularities Usage of Smart Electric Voucher using Support Vector Machines
WMA-1
EAI
DOI: 10.4108/eai.20-1-2018.2281928
Abstract
This paper applies machine-learning approach in identifying the irregularities of customer behaviour to the purchase transaction of smart electric vouchers. The Support Vector Machine (SVM) is used as the classification machine learning to identify the irregularities usage into two classes. The performance of the classification system is evaluated using the 10-fold cross-validation technique. Validation results are measured using accuracy, precision, and recall values. Our implementation results showed the use of SVM method gives very good performances in classifying the electrical consumption behaviour. Experimental results with different amounts of data testing indicated that the SVM method has high degree of accuracy, precision, and recall of 99 to 100%.