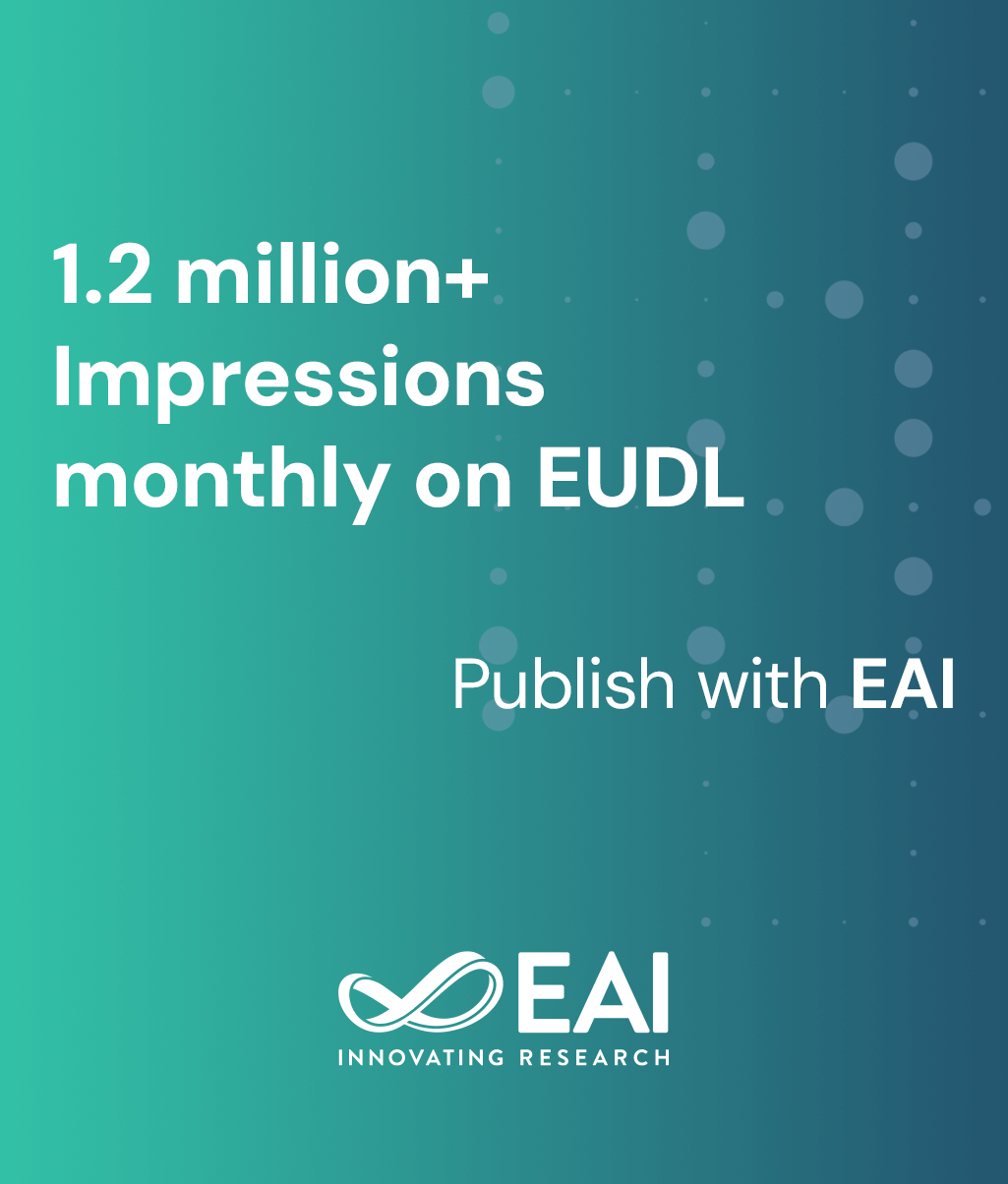
Research Article
Signature Identification with Gray Level Co-ccurrence Matrix and Extreme Learning Machine
@INPROCEEDINGS{10.4108/eai.20-1-2018.2281923, author={Roseri Sinaga and Tulus Tulus and Erna Budhiarti and A M H Pardede}, title={Signature Identification with Gray Level Co-ccurrence Matrix and Extreme Learning Machine}, proceedings={Proceedings of the 1st Workshop on Multidisciplinary and Its Applications Part 1, WMA-01 2018, 19-20 January 2018, Aceh, Indonesia}, publisher={EAI}, proceedings_a={WMA-1}, year={2019}, month={9}, keywords={signature identification extreme learning machine gray level co-occurrence matrix}, doi={10.4108/eai.20-1-2018.2281923} }
- Roseri Sinaga
Tulus Tulus
Erna Budhiarti
A M H Pardede
Year: 2019
Signature Identification with Gray Level Co-ccurrence Matrix and Extreme Learning Machine
WMA-1
EAI
DOI: 10.4108/eai.20-1-2018.2281923
Abstract
Identification of the signature is a process used to identify the signature of a person. The Identification of the signature can be divided into two parts. Identification signature off-line and the Identification of signatures on-line. Forgery of signatures is still common in data security systems. So, we need an approach to improve the accuracy of signature recognition on Extreme Learning Machine algorithms. This study use a gray level co - occurrence matrix (GLCM) for feature extraction and modification of Extreme Learning Machine (ELM) for recognition. ELM is a new learning method of a neural network or commonly called the Single Hidden Layer Feed forward Neural Networks (SLFNs). From the experiments the identification of the signature using the gray level co-occurrence matrix (GLCM) and signature classification using extreme learning machine with the addition of elementary transformations showed that the accuracy on identification of the signature is 43% using of features contrast, correlation, energy and homogeneity, while using just ELM accuracy is 36%.