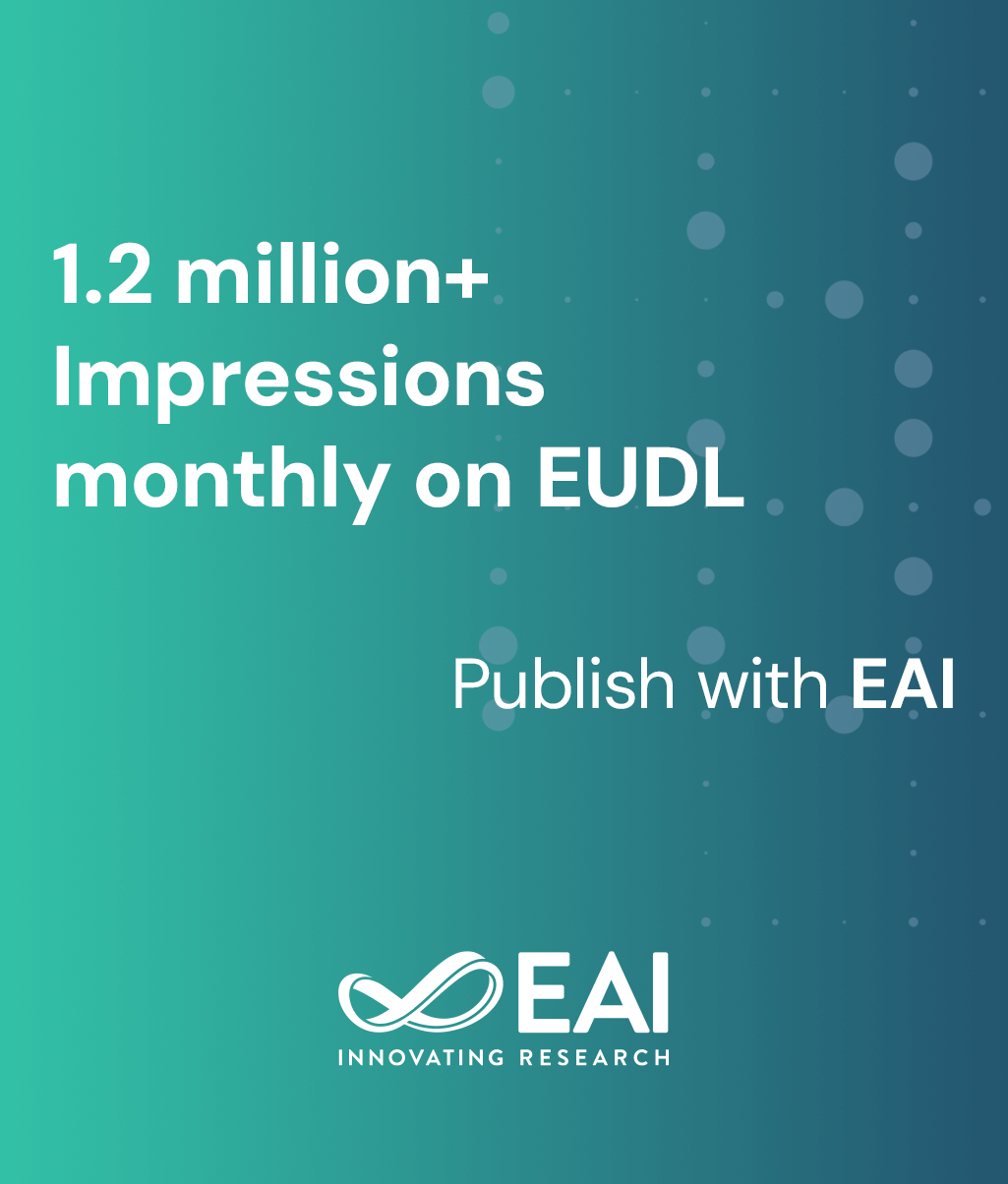
Research Article
Study of Robust Regression Modeling Using MM-Estimator and Least Median Squares
@INPROCEEDINGS{10.4108/eai.2-8-2019.2290533, author={Khusnul Khotimah and Kusman Sadik and Akbar Rizki}, title={Study of Robust Regression Modeling Using MM-Estimator and Least Median Squares}, proceedings={Proceedings of the 1st International Conference on Statistics and Analytics, ICSA 2019, 2-3 August 2019, Bogor, Indonesia}, publisher={EAI}, proceedings_a={ICSA}, year={2020}, month={1}, keywords={least median squares multi-stage method outliers robust regression root mean squares error}, doi={10.4108/eai.2-8-2019.2290533} }
- Khusnul Khotimah
Kusman Sadik
Akbar Rizki
Year: 2020
Study of Robust Regression Modeling Using MM-Estimator and Least Median Squares
ICSA
EAI
DOI: 10.4108/eai.2-8-2019.2290533
Abstract
Ordinary least squares (OLS) is a method commonly used to estimate regression equations. One solution handle OLS limitation to outlier problem is to use the robust regression method. This study used least-median squares (LMS) and multi-stage method (MM) robust regression. Simulation results of regression analysis in various scenarios are concluded that LMS and MM methods have better performance compared to OLS on data containing vertical and bad leverage point outliers. MM method has lowest average parameter estimation bias, followed by LMS, then OLS. LMS has smallest average root mean squares error (RMSE) and highest average R^2 is followed by MM then OLS. The results of the regression analysis comparison of the three methods on Indonesian rice production data in 2017 which contains 10% outliers were concluded that the LMS is the best method. The LMS produces the smallest RMSE of 4.44 and the highest R^2 that is 98%.