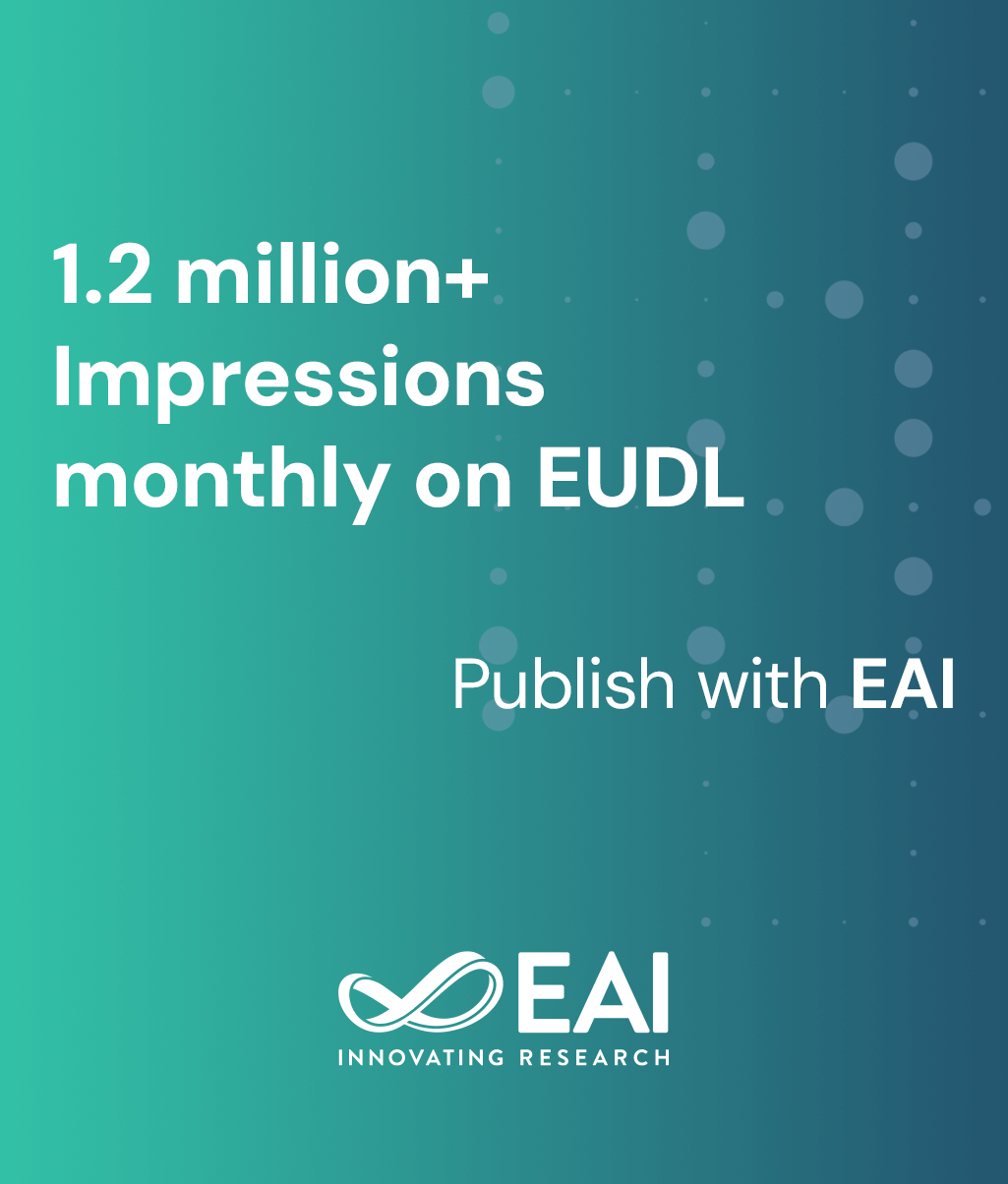
Research Article
Small Area Estimation with Penalty for Specific Area Effects Selection
@INPROCEEDINGS{10.4108/eai.2-8-2019.2290492, author={Novi Hidayat Pusponegoro and Anang Kurnia and Khairil Anwar Notodiputro and Agus Mohamad Sholeh and Erni Tri Astuti}, title={Small Area Estimation with Penalty for Specific Area Effects Selection}, proceedings={Proceedings of the 1st International Conference on Statistics and Analytics, ICSA 2019, 2-3 August 2019, Bogor, Indonesia}, publisher={EAI}, proceedings_a={ICSA}, year={2020}, month={1}, keywords={per capita income sparsity of random effects iterative algorithm}, doi={10.4108/eai.2-8-2019.2290492} }
- Novi Hidayat Pusponegoro
Anang Kurnia
Khairil Anwar Notodiputro
Agus Mohamad Sholeh
Erni Tri Astuti
Year: 2020
Small Area Estimation with Penalty for Specific Area Effects Selection
ICSA
EAI
DOI: 10.4108/eai.2-8-2019.2290492
Abstract
Small area estimation (SAE) techniques are now widely employed to produce parameter estimates for smaller domains where sample sizes cannot be used to deliver direct estimation. SAE as an indirect estimation method utilizes strength from other related small areas to improve the precision. In the 'Big data' era, database size and technology has developed rapidly. This leads to computational and statistical challenges since the availability of high data volume. Thus, the existing SAE methods can not longer handle the complexity of fixed effects or random effects in this data. The big data also provide large number areas as observation where not all them are small areas. This sparsity of random effects also brings out violation to its normal assumptions. Therefore, identifying the effective random effects is very important to ease the computational burden and to construct more interpretable models. This study presents a small area estimation method that is able to overcome the complexity of random effects with hard-ridge penalty. In this paper, simulations are delivered to demonstrate the performance of the methods and applied to estimate sub-district level mean of per capita income using the poverty survey data in Bangka Belitung Province at 2017.