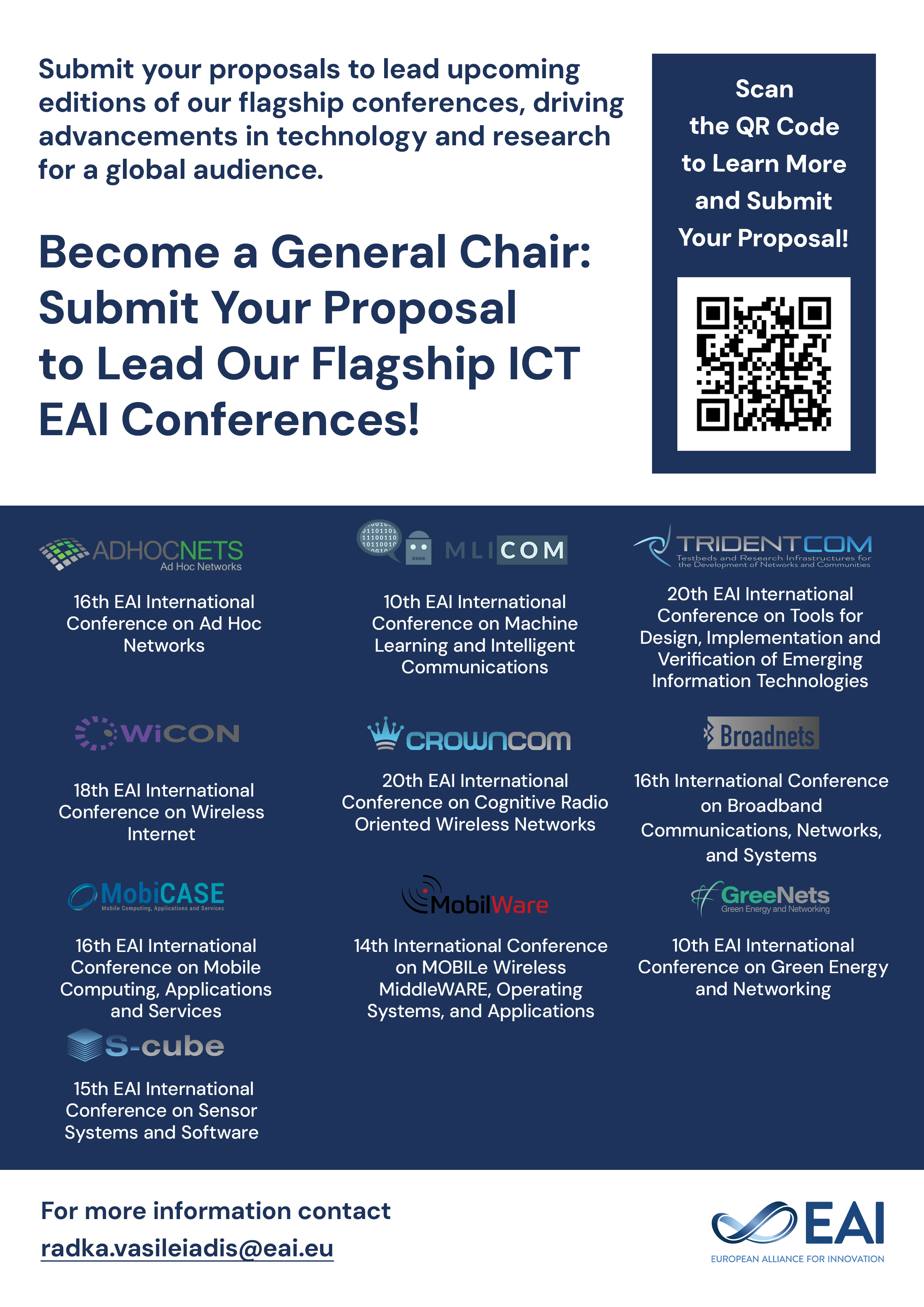
Research Article
BAYESIAN QUANTILE REGRESSION MODELING TO ESTIMATE EXTREME RAINFALL IN INDRAMAYU
@INPROCEEDINGS{10.4108/eai.2-8-2019.2290491, author={Eko Primadi Hendri and Aji Hamim Wigena and Anik Djuraidah}, title={BAYESIAN QUANTILE REGRESSION MODELING TO ESTIMATE EXTREME RAINFALL IN INDRAMAYU}, proceedings={Proceedings of the 1st International Conference on Statistics and Analytics, ICSA 2019, 2-3 August 2019, Bogor, Indonesia}, publisher={EAI}, proceedings_a={ICSA}, year={2020}, month={1}, keywords={bayesian quantile regression lasso mcmc statistical downscaling}, doi={10.4108/eai.2-8-2019.2290491} }
- Eko Primadi Hendri
Aji Hamim Wigena
Anik Djuraidah
Year: 2020
BAYESIAN QUANTILE REGRESSION MODELING TO ESTIMATE EXTREME RAINFALL IN INDRAMAYU
ICSA
EAI
DOI: 10.4108/eai.2-8-2019.2290491
Abstract
Quantile regression can be used to analyze symmetric or asymmetric data. Estimates of quantile regression parameters are obtained by the simplex method. Another approach is the Bayesian method based on Laplace's asymmetric distribution using MCMC. MCMC is used numerically to estimate parameters from each posterior distribution. The Bayesian quantile regression and the quantile regression can be used for statistical downscaling in extreme rainfall cases. This study used statistical downscaling to obtain relationship between global-scale data and local-scale data. The data used were monthly rainfall data in Indramayu and GCM output data. LASSO regularization was used to overcome multicollinearity problems in GCM output data. The purpose of this study was to compare Bayesian quantile regression models with quantile regression. The Bayesian quantile regression and the quantile regression couldpredict extreme rainfallmore accurate and consistent in one year ahead. The Bayesian quantile regression model is relatively better than the quantile regression.