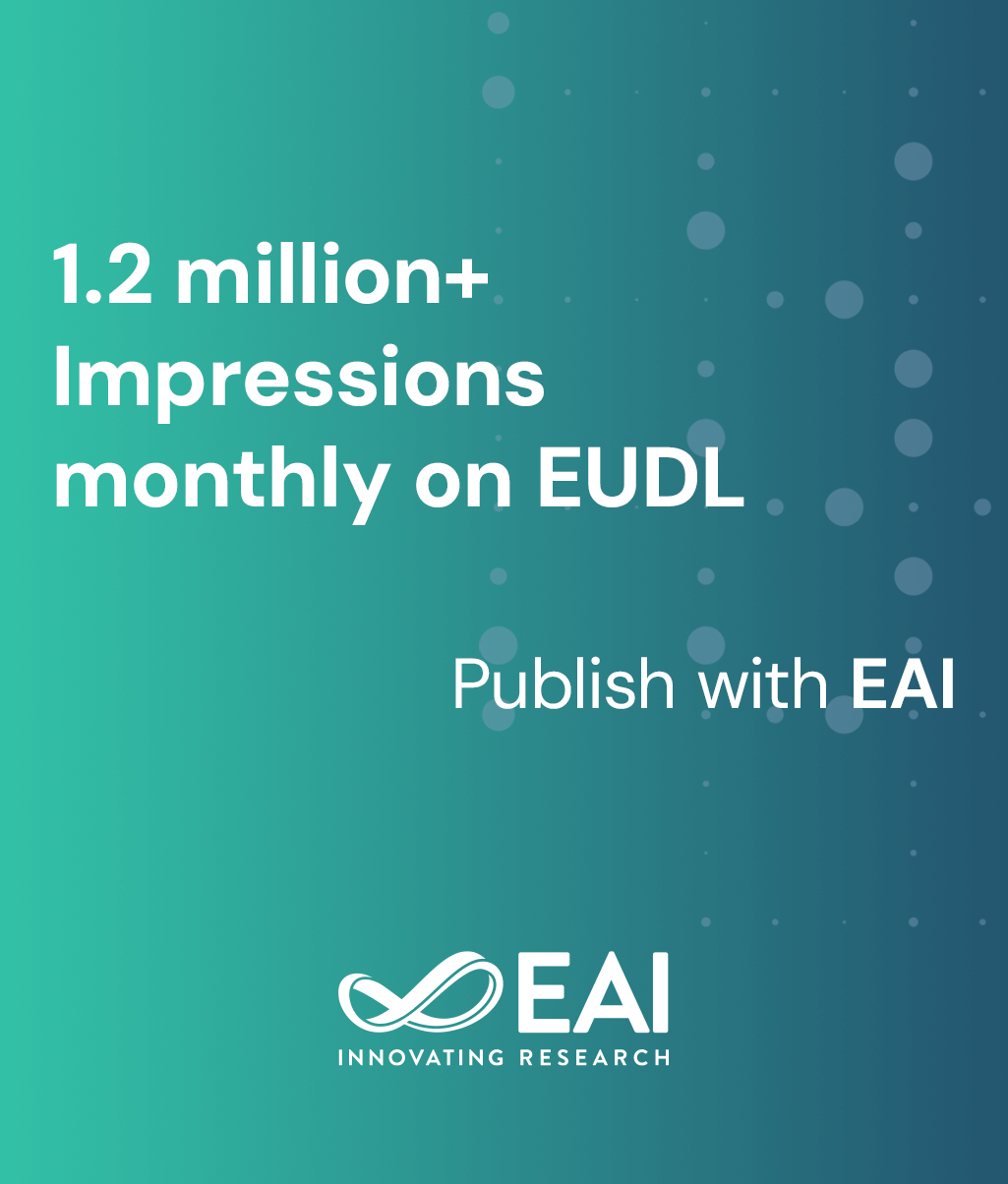
Research Article
Comparison of Maximum Likelihood and Generalized Method of Moments in Spatial Autoregressive Model with Heteroskedasticity
@INPROCEEDINGS{10.4108/eai.2-8-2019.2290489, author={Rohimatul Anwar and Anik Djuraidah and Aji Hamim Wigena}, title={Comparison of Maximum Likelihood and Generalized Method of Moments in Spatial Autoregressive Model with Heteroskedasticity}, proceedings={Proceedings of the 1st International Conference on Statistics and Analytics, ICSA 2019, 2-3 August 2019, Bogor, Indonesia}, publisher={EAI}, proceedings_a={ICSA}, year={2020}, month={1}, keywords={heteroskedasticity spatial autoregressive maximum likelihood generalized moment method}, doi={10.4108/eai.2-8-2019.2290489} }
- Rohimatul Anwar
Anik Djuraidah
Aji Hamim Wigena
Year: 2020
Comparison of Maximum Likelihood and Generalized Method of Moments in Spatial Autoregressive Model with Heteroskedasticity
ICSA
EAI
DOI: 10.4108/eai.2-8-2019.2290489
Abstract
Spatial dependence and spatial heteroskedasticity are problems in spatial regression. Spatial autoregressive regression (SAR) concerns only to the dependence on lag. The estimation of SAR parameters containingheteroskedasticityusing the maximum likelihood estimation (MLE) method provides biased and inconsistent. The alternative method is the generalized method of moments (GMM). GMM uses a combination of linear and quadratic moment functions simultaneously so that the computation is easier than MLE. The bias is used to evaluate the GMM in estimating parameters of SAR model with heteroskedasticity disturbances in simulation data. The results show that GMM provides the bias of parameter estimates relatively consistent and smaller compared to the MLE method.