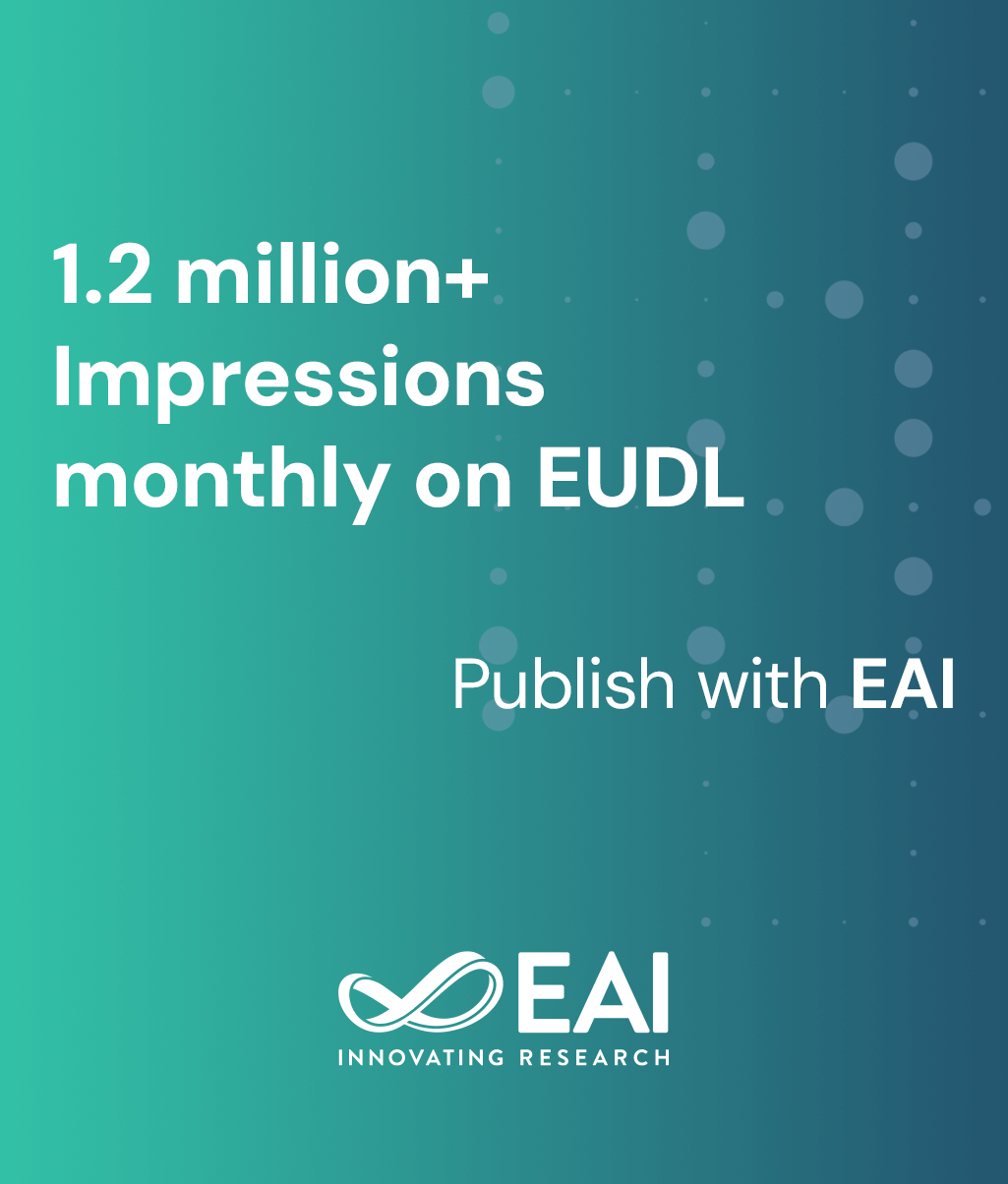
Research Article
Improved Deep Reinforcement Learning Algorithms and Applications in Quantitative Trading in Extreme Stock Markets
@INPROCEEDINGS{10.4108/eai.2-6-2023.2334605, author={Deguan Cui and Ailin Deng and Zhengxun Xia and Liqi Zeng}, title={Improved Deep Reinforcement Learning Algorithms and Applications in Quantitative Trading in Extreme Stock Markets}, proceedings={Proceedings of the 2nd International Conference on Information Economy, Data Modeling and Cloud Computing, ICIDC 2023, June 2--4, 2023, Nanchang, China}, publisher={EAI}, proceedings_a={ICIDC}, year={2023}, month={8}, keywords={deep reinforcement learning td algorithm quantitative trading extreme markets}, doi={10.4108/eai.2-6-2023.2334605} }
- Deguan Cui
Ailin Deng
Zhengxun Xia
Liqi Zeng
Year: 2023
Improved Deep Reinforcement Learning Algorithms and Applications in Quantitative Trading in Extreme Stock Markets
ICIDC
EAI
DOI: 10.4108/eai.2-6-2023.2334605
Abstract
Aiming at the issue that existing deep reinforcement learning algorithms cannot achieve satisfactory returns in the quantitative trading process, particularly during extreme stock market conditions such as sharp declines, we propose an improved TD algorithm based on immediate reward R_t, which we name as RTD. We further extend RTD to multi-step conditions (MRTD) and apply it to enhance algorithms such as DQN and DDPG. We then utilize these two improved algorithms in the stock quantitative trading process. Experimental results demonstrate that our proposed algorithms can respond to market changes more efficiently, resulting in improved accuracy of investment strategies and higher investment return rates, even during extreme market conditions. For instance, when the market declines by more than 10%, and the two specified stocks decline by nearly 5.09% and 13.30%, we can still obtain return rates of 2.78% and 4.19% respectively, which confirms the effectiveness of our proposed algorithms.