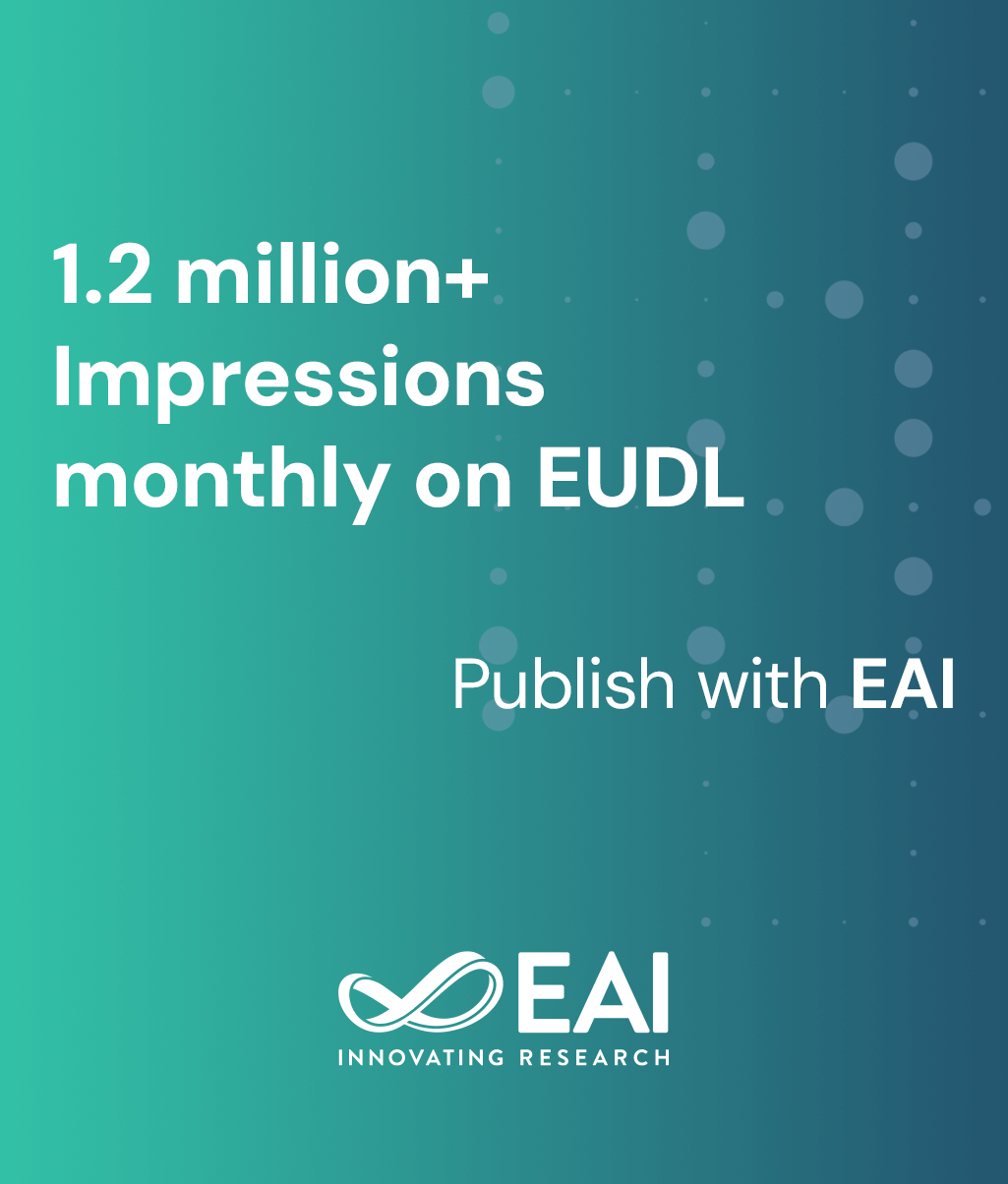
Research Article
Face recognition based on LDA in manifold subspace
@ARTICLE{10.4108/eai.2-5-2016.151209, author={Hung Phuoc Truong and Tue-Minh Dinh Vo and Thai Hoang Le}, title={Face recognition based on LDA in manifold subspace}, journal={EAI Endorsed Transactions on Context-aware Systems and Applications}, volume={3}, number={9}, publisher={EAI}, journal_a={CASA}, year={2016}, month={5}, keywords={face recognition, manifold learning, semi-supervised, discriminative}, doi={10.4108/eai.2-5-2016.151209} }
- Hung Phuoc Truong
Tue-Minh Dinh Vo
Thai Hoang Le
Year: 2016
Face recognition based on LDA in manifold subspace
CASA
EAI
DOI: 10.4108/eai.2-5-2016.151209
Abstract
Although LDA has many successes in dimensionality reduction and data separation, it also has disadvantages, especially the small sample size problem in training data because the "within-class scatter" matrix may not be accurately estimated. Moreover, this algorithm can only operate correctly with labeled data in supervised learning. In practice, data collection is very huge and labeling data requires high-cost, thus the combination of a part of labeled data and unlabeled data for this algorithm in Manifold subspace is a novelty research. This paper reports a study that propose a semi-supervised method called DSLM, which aims at overcoming all these limitations. The proposed method ensures that the discriminative information of labeled data and the intrinsic geometric structure of data are mapped to new optimal subspace. Results are obtained from the experiments and compared to several related methods showing the effectiveness of our proposed method.
Copyright © 2016 H. P. Truong et al., licensed to EAI. This is an open access article distributed under the terms of the Creative Commons Attribution licence (http://creativecommons.org/licenses/by/3.0/), which permits unlimited use, distribution and reproduction in any medium so long as the original work is properly cited.