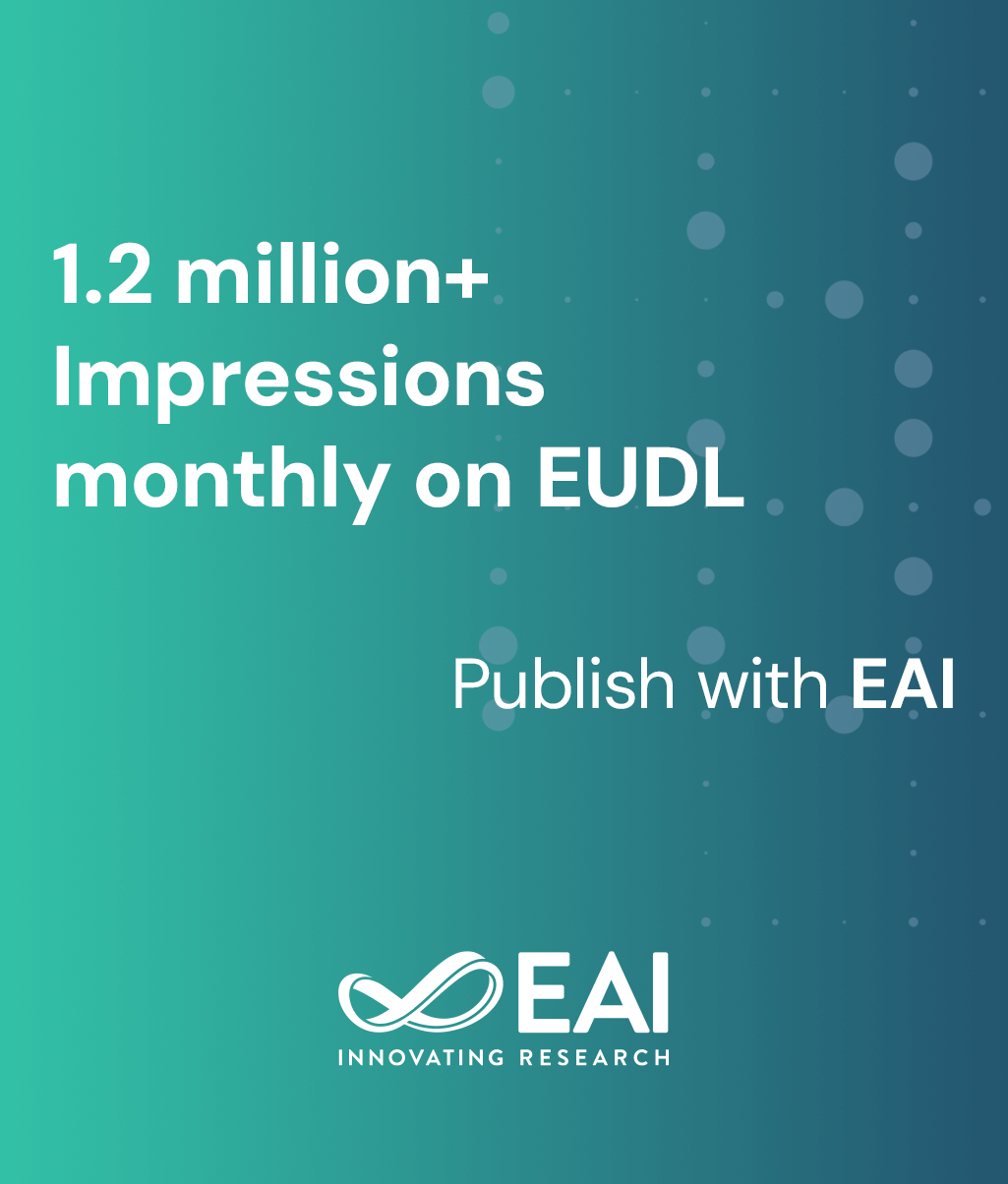
Research Article
Rating Prediction Method for Item-based Collaborative Filtering Recommender Systems Using Formal Concept Analysis
@ARTICLE{10.4108/eai.2-12-2020.167291, author={Chemmalar Selvi G. and Lakshmi Priya G.G.}, title={Rating Prediction Method for Item-based Collaborative Filtering Recommender Systems Using Formal Concept Analysis}, journal={EAI Endorsed Transactions on Energy Web}, volume={8}, number={33}, publisher={EAI}, journal_a={EW}, year={2020}, month={12}, keywords={Recommender system, Collaborative Filtering, Item-Based Collaborative Filtering, Top-n recommendation, Formal concept analysis}, doi={10.4108/eai.2-12-2020.167291} }
- Chemmalar Selvi G.
Lakshmi Priya G.G.
Year: 2020
Rating Prediction Method for Item-based Collaborative Filtering Recommender Systems Using Formal Concept Analysis
EW
EAI
DOI: 10.4108/eai.2-12-2020.167291
Abstract
The recommender systems are used to mainly suggest recommendations to the online users by utilizing the user preferences recorded during the item purchase. No matter how, the performance of the recommendation quality seems to be inevitable and far satisfactory. In this paper, a new approach based on the mathematical model, Formal Concept Analysis (FCA) is used to improve the rating prediction of the unknown users which can certainly overcome the issues of the existing approaches like data sparsity, high dimensionality of data, performance of the recommendation generated for top n recommendation. The FCA method is applied using Boolean Matrix Factorization (ie. optimal formal concepts) in predicting the rating of the unknown users in the available user-item interaction matrix which proves to be more efficientin tackling the problem of computational complexity managing the high dimensionality of data. The proposed method is applied using item-based collaborative filtering technique and the experiment is conducted on the Movielens dataset which shows the satisfactory results. The experiments results are evaluated using the related error metrics and performance metrics. The experimental results are also compared with existing item-based Collaborative Filtering techniques which demonstrate that the performance of recommendation quality gradually improved with state-of-the-art existing techniques.
Copyright © 2020 Chemmalar Selvi G. et al., licensed to EAI. This is an open access article distributed under the terms of the Creative Commons Attribution license, which permits unlimited use, distribution and reproduction in any medium so long as the original work is properly cited.