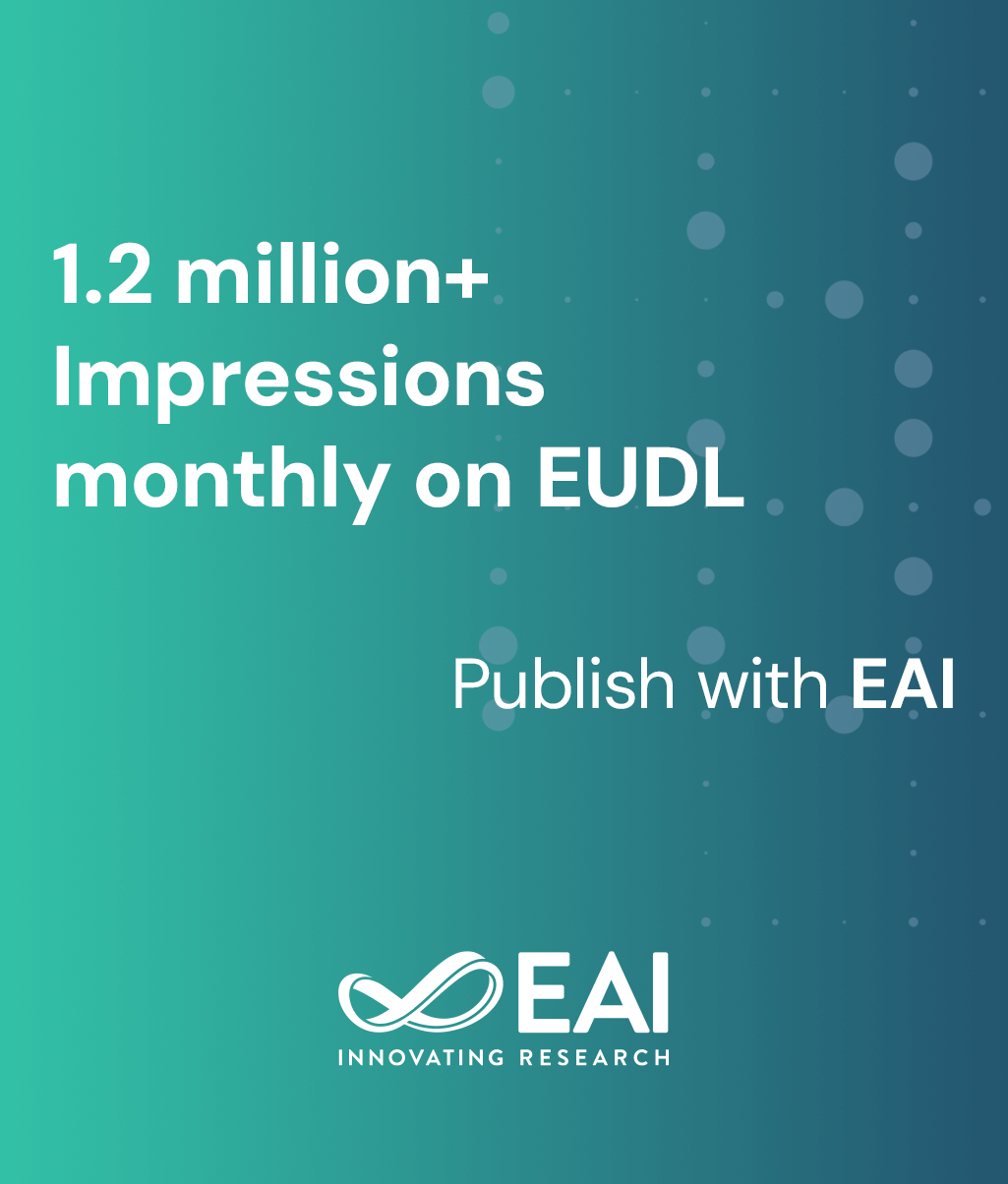
Research Article
Scheduling Hybrid Spark Jobs Based on Deep Reinforcement Learning
@INPROCEEDINGS{10.4108/eai.19-5-2023.2334333, author={Kun Chen and Jia Wang}, title={Scheduling Hybrid Spark Jobs Based on Deep Reinforcement Learning}, proceedings={Proceedings of the 2nd International Conference on Bigdata Blockchain and Economy Management, ICBBEM 2023, May 19--21, 2023, Hangzhou, China}, publisher={EAI}, proceedings_a={ICBBEM}, year={2023}, month={7}, keywords={spark drl hybrid job utilization cost}, doi={10.4108/eai.19-5-2023.2334333} }
- Kun Chen
Jia Wang
Year: 2023
Scheduling Hybrid Spark Jobs Based on Deep Reinforcement Learning
ICBBEM
EAI
DOI: 10.4108/eai.19-5-2023.2334333
Abstract
As a popular big data computing framework, Spark requires effective job scheduling to optimize resource utilization and execute applications efficiently. However, the hy-brid jobs (jobs with and without deadlines) and the heterogeneous clusters bring great challenges for job scheduling. In this paper, a job scheduling based on deep rein-forcement learning is proposed. A weight-based job sorting strategy is designed to ob-tain better job scheduling. The proposed method is evaluated with large-scale real-world data. Experimental results show that more jobs can satisfy deadline constraints and the cost of cluster utilization is reduced.
Copyright © 2023–2025 EAI