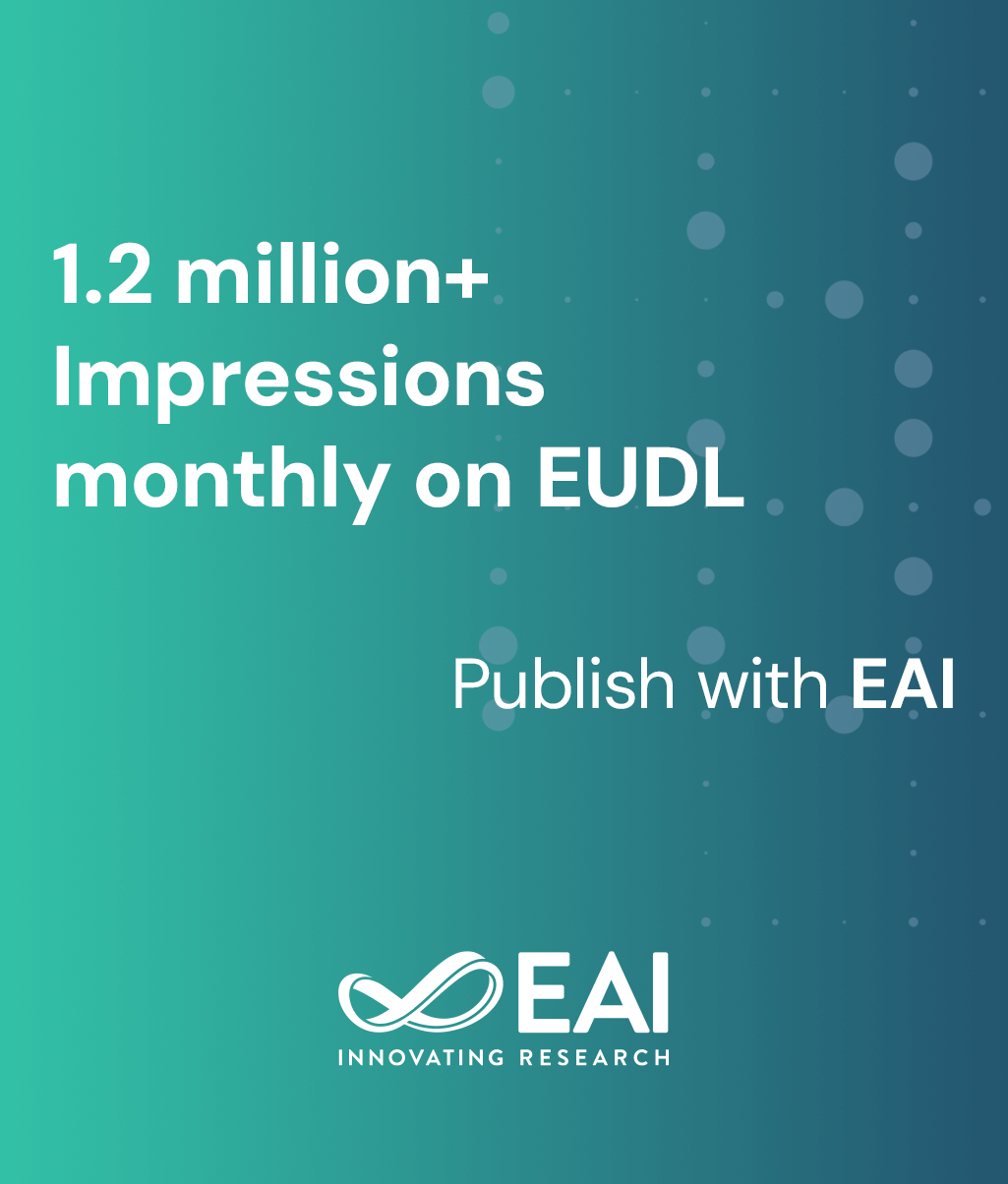
Research Article
Stock Price Prediction using Artificial Neural Model: An Application of Big Data
@ARTICLE{10.4108/eai.19-12-2018.156085, author={Malav Shastri and Sudipta Roy and Mamta Mittal}, title={Stock Price Prediction using Artificial Neural Model: An Application of Big Data}, journal={EAI Endorsed Transactions on Scalable Information Systems}, volume={6}, number={20}, publisher={EAI}, journal_a={SIS}, year={2019}, month={1}, keywords={News Headlines, Stock Market, Big Data, Artificial Intelligence, Artificial Neural Networks, Sentimental Analysis}, doi={10.4108/eai.19-12-2018.156085} }
- Malav Shastri
Sudipta Roy
Mamta Mittal
Year: 2019
Stock Price Prediction using Artificial Neural Model: An Application of Big Data
SIS
EAI
DOI: 10.4108/eai.19-12-2018.156085
Abstract
In recent time, stock price prediction is an area of profound interest in the realm of fiscal market. To predict the stock prices, authors have proposed a technique by first calculating the sentiment scores through Naïve Bayes classifier and after that neural network is applied on both sentiment scores and historical stock dataset. They have also addressed the issue of data cleaning using a Hive ecosystem. This ecosystem is being used for pre-processing part and a neural network model with inputs from sentiment analysis and historic data is used to predict the prices. It has been observed from the experiments that the accuracy level reaches above 90% in maximum cases, as well as it also provides the solid base that model will be more accurate if it trained with recent data. The intended combination of sentiment analysis and Neural networks is used to establish a statistical relationship between historic numerical data records of a particular stock and other sentimental factors which can affects the stock prices.
Copyright © 2019 Malav Shastri et al., licensed to EAI. This is an open access article distributed under the terms of the Creative Commons Attribution licence (http://creativecommons.org/licenses/by/3.0/), which permits unlimited use, distribution and reproduction in any medium so long as the original work is properly cited.