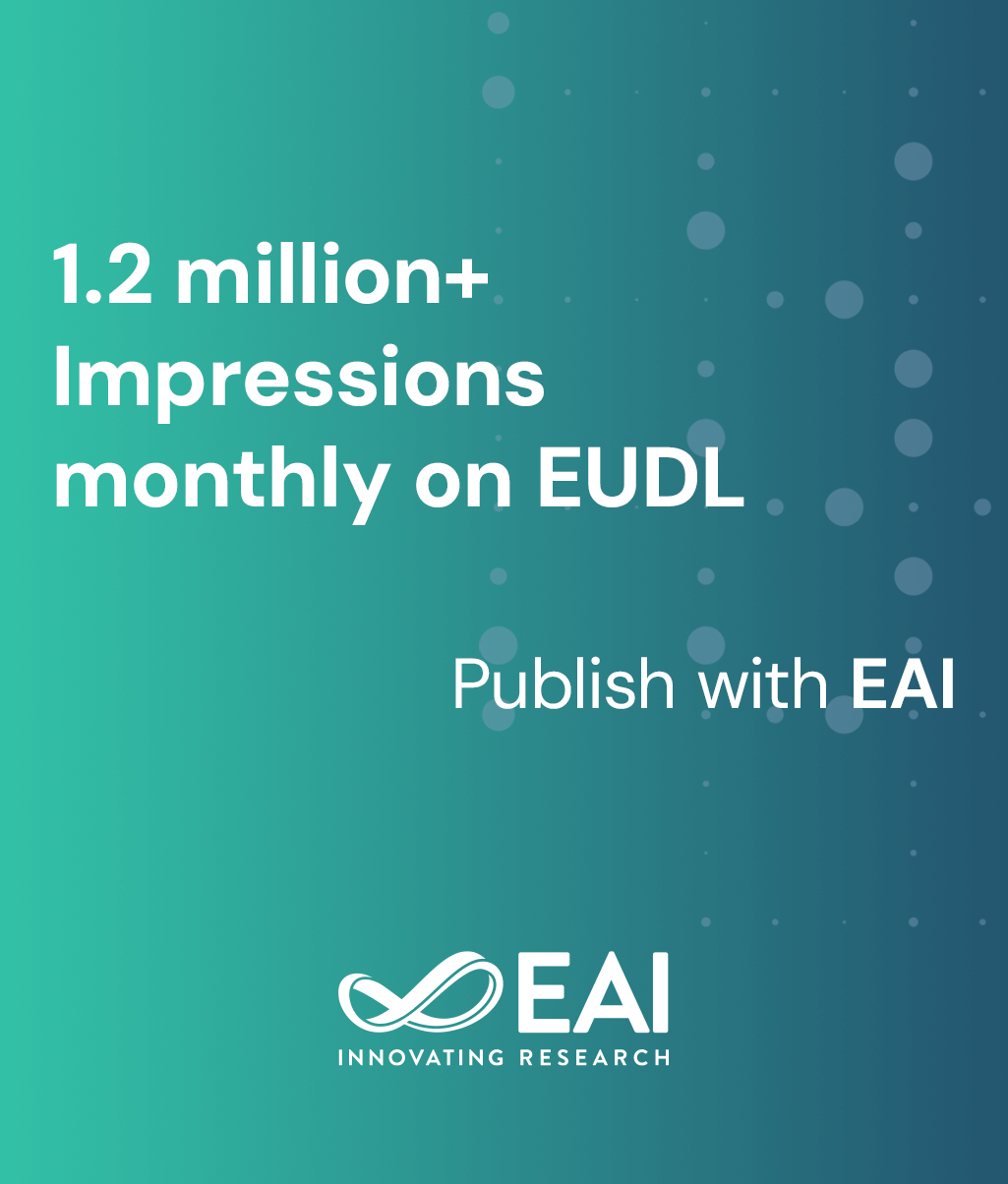
Research Article
Convolutional block attention module based on visual mechanism for robot image edge detection
@ARTICLE{10.4108/eai.19-11-2021.172214, author={Aiyun Ju and Zhongli Wang}, title={Convolutional block attention module based on visual mechanism for robot image edge detection}, journal={EAI Endorsed Transactions on Scalable Information Systems}, volume={9}, number={36}, publisher={EAI}, journal_a={SIS}, year={2021}, month={11}, keywords={deep learning, CBAM, visual mechanism, robot image edge detection}, doi={10.4108/eai.19-11-2021.172214} }
- Aiyun Ju
Zhongli Wang
Year: 2021
Convolutional block attention module based on visual mechanism for robot image edge detection
SIS
EAI
DOI: 10.4108/eai.19-11-2021.172214
Abstract
In recent years, with the continuous development of computer vision, digital image and other information technology, its application in robot image has attracted many domestic and foreign scholars to conduct researches. Edge detection technology based on traditional deep learning produces messy and fuzzy edge lines. Therefore, we present a new convolutional block attention module (CBAM) based on visual mechanism for robot image edge detection. CBAM is added into the trunk network, and a down-sampling technique with translation invariance is adopted. Some down-sampling operations in the trunk network are removed to retain the details of the image. Meanwhile, the extended convolution technique is used to increase the model's receptive field. Training is carried out on BSDS500 and PASCAL VOL Context datasets. We use the image pyramid technique to enhance the edges quality during testing. Experimental results show that the proposed model can extract image contour more clearly than other networks, and can solve the problem of edge blur.
Copyright © 2021 Aiyun Ju et al., licensed to EAI. This is an open access article distributed under the terms of the Creative Commons Attribution license, which permits unlimited use, distribution and reproduction in any medium so long as the original work is properly cited.