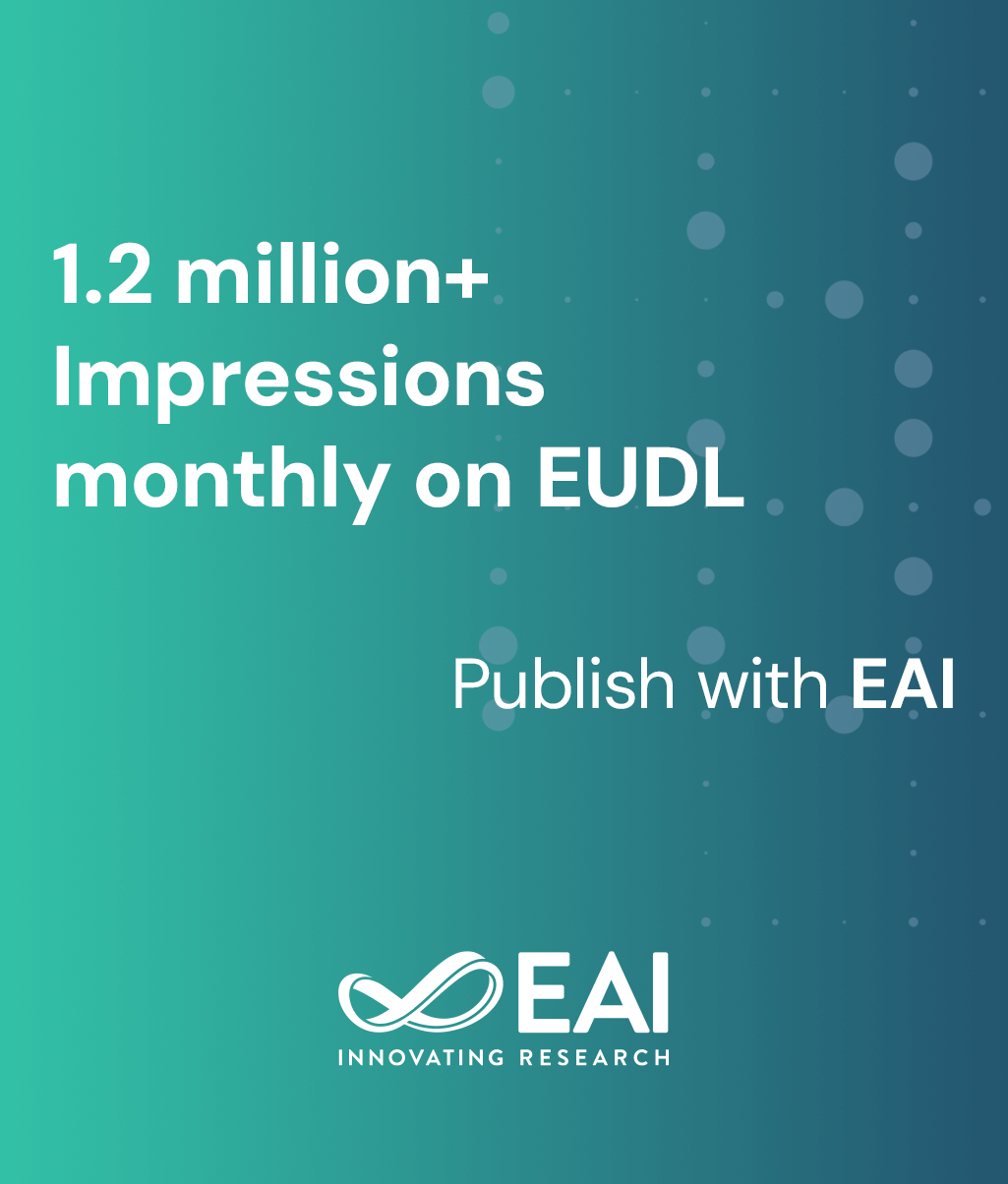
Research Article
Augmenting EEG with Inertial Sensing for Improved 4-Class Subject-Independent Emotion Classification in Virtual Reality
@INPROCEEDINGS{10.4108/eai.18-7-2019.2287946, author={Jason Teo and Nazmi Sofian bin Datuk Suhaimi and James Mountstephens}, title={Augmenting EEG with Inertial Sensing for Improved 4-Class Subject-Independent Emotion Classification in Virtual Reality}, proceedings={Proceedings of the 1st International Conference on Informatics, Engineering, Science and Technology, INCITEST 2019, 18 July 2019, Bandung, Indonesia}, publisher={EAI}, proceedings_a={INCITEST}, year={2019}, month={10}, keywords={inertial sensing emotion classification virtual reality 4-quadrant emotion recognition electroencephalography}, doi={10.4108/eai.18-7-2019.2287946} }
- Jason Teo
Nazmi Sofian bin Datuk Suhaimi
James Mountstephens
Year: 2019
Augmenting EEG with Inertial Sensing for Improved 4-Class Subject-Independent Emotion Classification in Virtual Reality
INCITEST
EAI
DOI: 10.4108/eai.18-7-2019.2287946
Abstract
This investigation reports on the promising results obtained from the novel use of inertial sensing data for augmenting electroencephalography (EEG)-based subject-independent classification of emotions generated by virtual reality stimuli in four classes. 31 users were shown various virtual reality scenes to elicit responses in the four-quadrant emotional space according to Russell’s Circumplex Model of Affect. Prior studies in emotion classification can be broadly grouped according to two main delineations of investigative methods: (1) whether the classification is binary (i.e. two-class classification) or otherwise (e.g. three-class, four-class classification or more) and (2) whether the training and testing occurs within the same participant (also known as subject-dependent classification) or across different participants (also known as subject-independent classification). Due to the significantly higher level of difficulty in conducting ternary/quaternary, subject-independent classification, the large majority of emotion modeling studies that report high accuracy rates adopts the binary, subject-dependent approach to classification. However, this study attempts the more challenging four-class classification, subject-independent classification. The EEG signals, accelerometer, and gyroscopic data were acquired through a wearable brain-computer interface device called Muse.