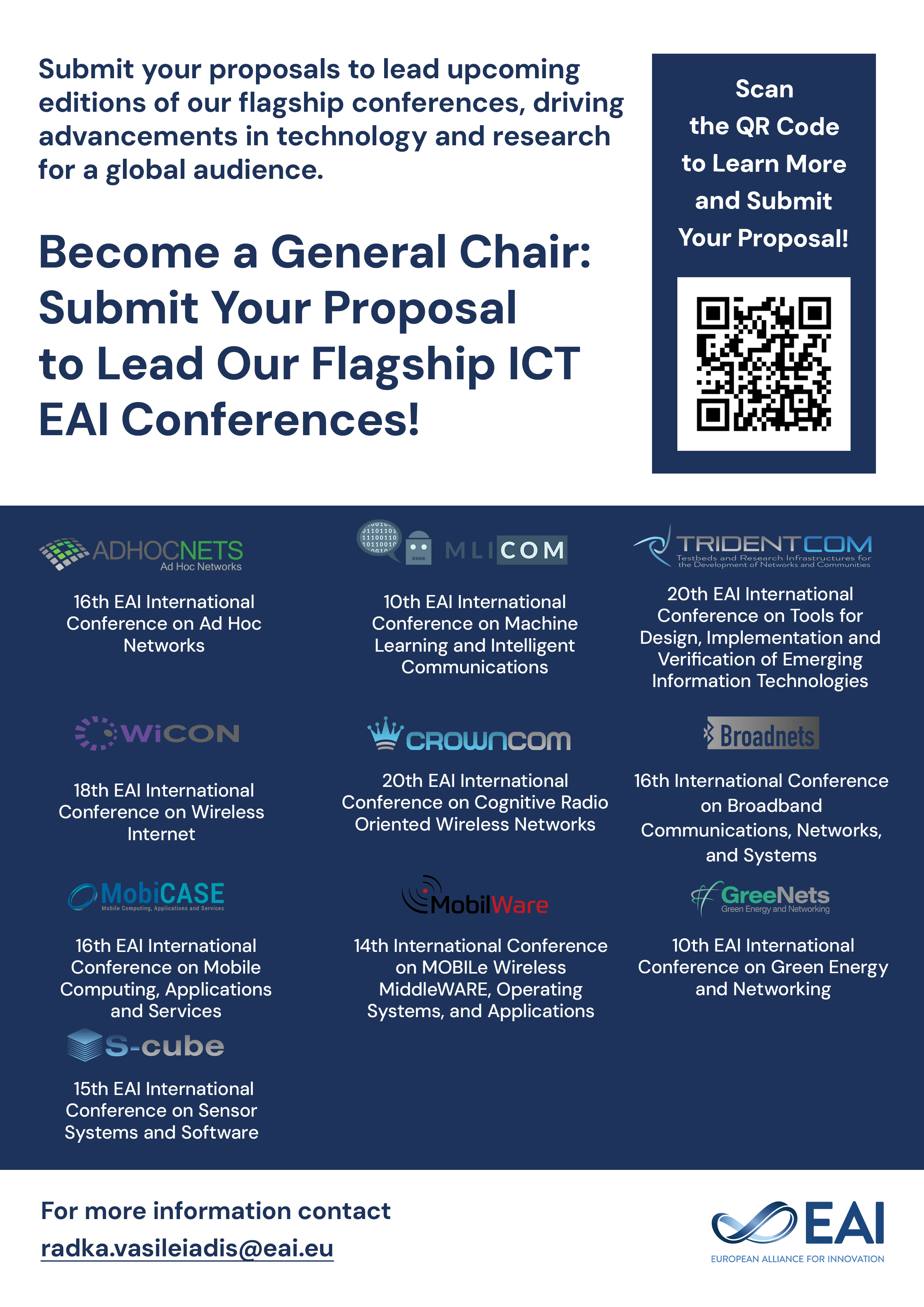
Research Article
Convolutional Neural Networks Deep Learning Based for Malaria Detection and Diagnosis
@INPROCEEDINGS{10.4108/eai.18-12-2023.2348130, author={Josue Ouedraogo and Ferdinand T. Guinko and Kiswendsida Kisito Kabore}, title={Convolutional Neural Networks Deep Learning Based for Malaria Detection and Diagnosis}, proceedings={Proceedings of the 6th Computer Science Research Days, JRI 2023, 18-20 December 2023, Ouagadougou, Burkina Faso}, publisher={EAI}, proceedings_a={JRI}, year={2024}, month={6}, keywords={malaria deep learning object detection yolov5 plasmodium falciparum}, doi={10.4108/eai.18-12-2023.2348130} }
- Josue Ouedraogo
Ferdinand T. Guinko
Kiswendsida Kisito Kabore
Year: 2024
Convolutional Neural Networks Deep Learning Based for Malaria Detection and Diagnosis
JRI
EAI
DOI: 10.4108/eai.18-12-2023.2348130
Abstract
Malaria is a disease that occurs worldwide, especially in tropical regions where a high prevalence is observed. Difficulties are encountered especially in developing countries where resources in terms of equipment and trained personnel are limited. Until today, microscopic analysis is the standard method for diagnosing Plasmodium falciparum, which is the causative agent of malaria. In this paper, we proposed a malaria detection and diagnosis system using a deep learning technique which is a convolutional neural network called YOLOv5. Model learning was performed using a combination of two given image sources, Delgado Dataset B and Dijkstra Dataset, as a dataset containing thin smear images. We then evaluated the performance of the model by comparing it with other state-of-the-art results on deep learning. We obtained for the detection, a mean Average Precision of 96.71%.