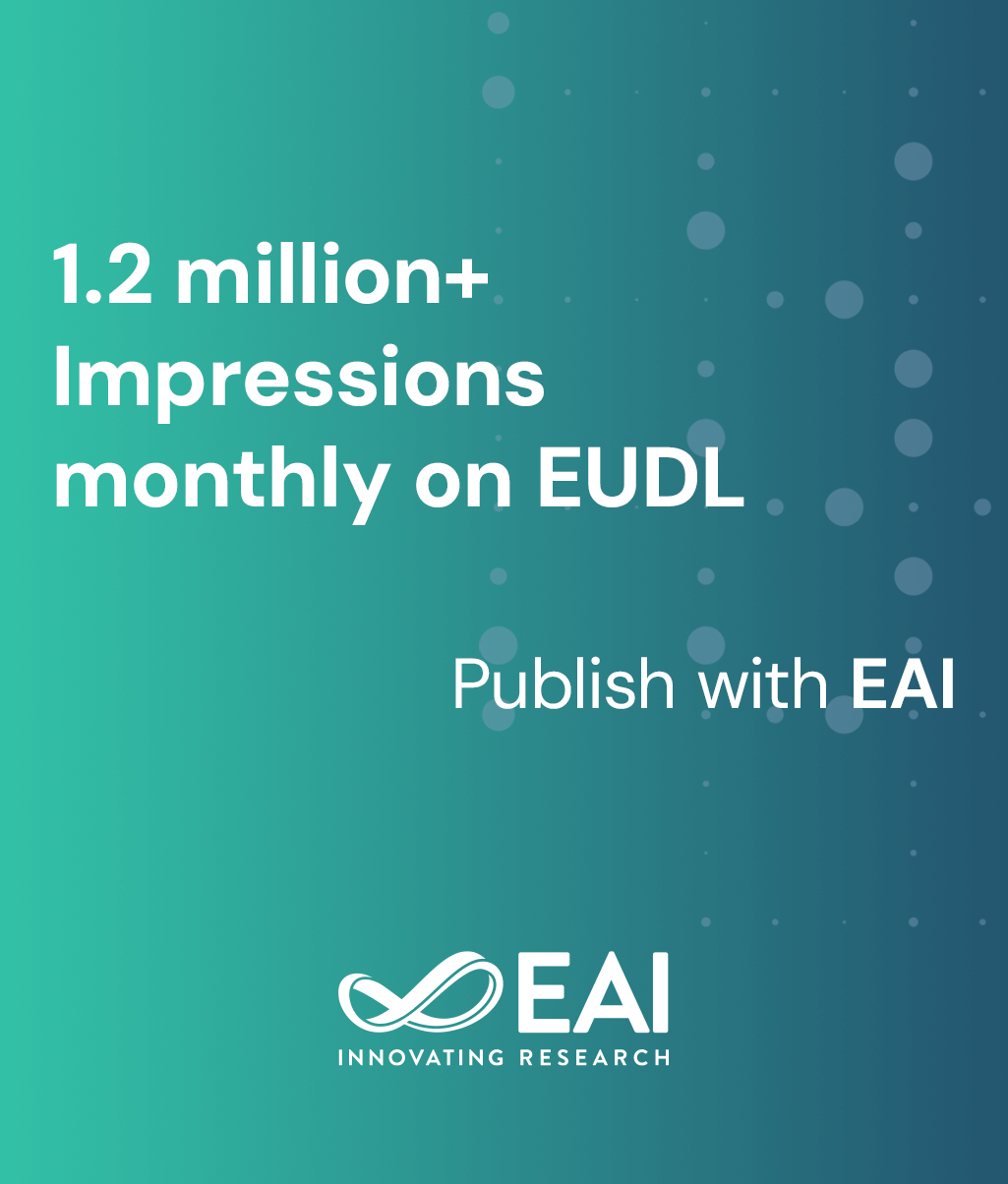
Research Article
Advancing Structured Prediction for 3D Polygonal Model Reconstruction from Single and Multiple Scene Images
@INPROCEEDINGS{10.4108/eai.18-12-2023.2348109, author={Debjyoti Chattopadhyay and Isha Sahni and Arpan Dutta Chowdhury}, title={Advancing Structured Prediction for 3D Polygonal Model Reconstruction from Single and Multiple Scene Images}, proceedings={Proceedings of the 6th Computer Science Research Days, JRI 2023, 18-20 December 2023, Ouagadougou, Burkina Faso}, publisher={EAI}, proceedings_a={JRI}, year={2024}, month={6}, keywords={computer vision 3d-model reconstruction multiple--view reconstruction}, doi={10.4108/eai.18-12-2023.2348109} }
- Debjyoti Chattopadhyay
Isha Sahni
Arpan Dutta Chowdhury
Year: 2024
Advancing Structured Prediction for 3D Polygonal Model Reconstruction from Single and Multiple Scene Images
JRI
EAI
DOI: 10.4108/eai.18-12-2023.2348109
Abstract
We introduce a novel approach for structured prediction aimed at reconstructing 3D polygonal models from both single and multiple images capturing a scene. Building upon recent advancements in single-view reconstruction, we embrace the indoor Manhattan hypothesis category – an intricate set of potential outputs characterised by complex internal constraints – integrated into a structured prediction framework. Our methodology is adaptable for learning in both single-view and multiview scenarios. It is demonstrated that this chosen hypothesis category enables the optimization of diverse high-level loss functions, including metrics such as the relative depth error. Our achieved outcomes surpass the current state-of-the-art, showcasing an enhancement of over 50% in a specific metric.