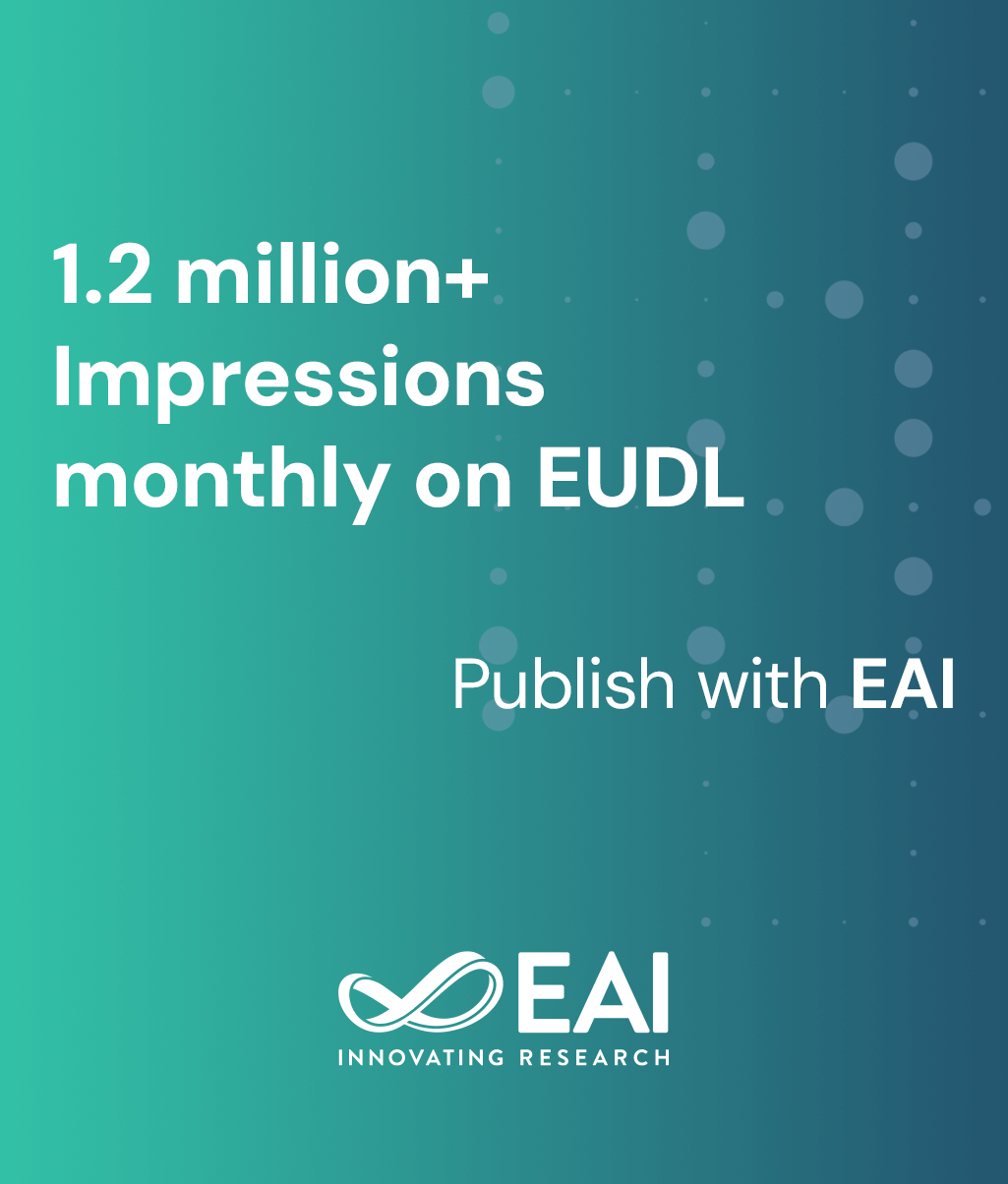
Research Article
The Investment Strategy Optimization based on BL Stock Price Selection based on Arima and Time Series fitting based on Monte Carlo and Optimization Strategy
@INPROCEEDINGS{10.4108/eai.18-11-2022.2326922, author={Li Yuan and Xuan Zhou}, title={The Investment Strategy Optimization based on BL Stock Price Selection based on Arima and Time Series fitting based on Monte Carlo and Optimization Strategy}, proceedings={Proceedings of the 4th International Conference on Economic Management and Model Engineering, ICEMME 2022, November 18-20, 2022, Nanjing, China}, publisher={EAI}, proceedings_a={ICEMME}, year={2023}, month={2}, keywords={investment strategy optimization; financial modeling; arima model; monte carlo simulation; black-litterman model; stock allocation}, doi={10.4108/eai.18-11-2022.2326922} }
- Li Yuan
Xuan Zhou
Year: 2023
The Investment Strategy Optimization based on BL Stock Price Selection based on Arima and Time Series fitting based on Monte Carlo and Optimization Strategy
ICEMME
EAI
DOI: 10.4108/eai.18-11-2022.2326922
Abstract
Investment strategy optimization is largely discussed in the financial industry and academia as investors seek to maximize the Return with limited input. This paper discusses how potentially profitable stocks can be selected using statistical models to form a portfolio and then use the predicted prices to find the optimal allocation strategy. We first use the ARIMA Model to predict the stock price on the following 21st day. We further verify the stock selection with Monte Carlo Simulation predicting the price ranges which the stocks will fall into in the next year. Then Black-Litterman Model is used to optimize the asset allocation. We select five stocks with non-negative returns based on the results to form the target portfolio: TSLA, KO, AMD, NKE, and ORCL. According to our simulation, the stocks have an annual profit of 3%, 2%, 7,8%, 3.4%, and 5.1%, respectively. The 95% confidence interval of those five stocks is small which demonstrates that the stock we choose has low risk. For instance, the confidence interval for the TSLA is (-0.05,0.06). Next, we get the weight of each stock to be 27.92%, 0%, 39.61%, 10.10%, and 22.38%, respectively. This asset allocation is the optimal choice. Comparing to the equal weight stock selection, although the equal weight stock selection shows lower variance, our asset allocation has higher profit. In conclusion, we show that the above five stocks will have positive profits in both the short and long run.