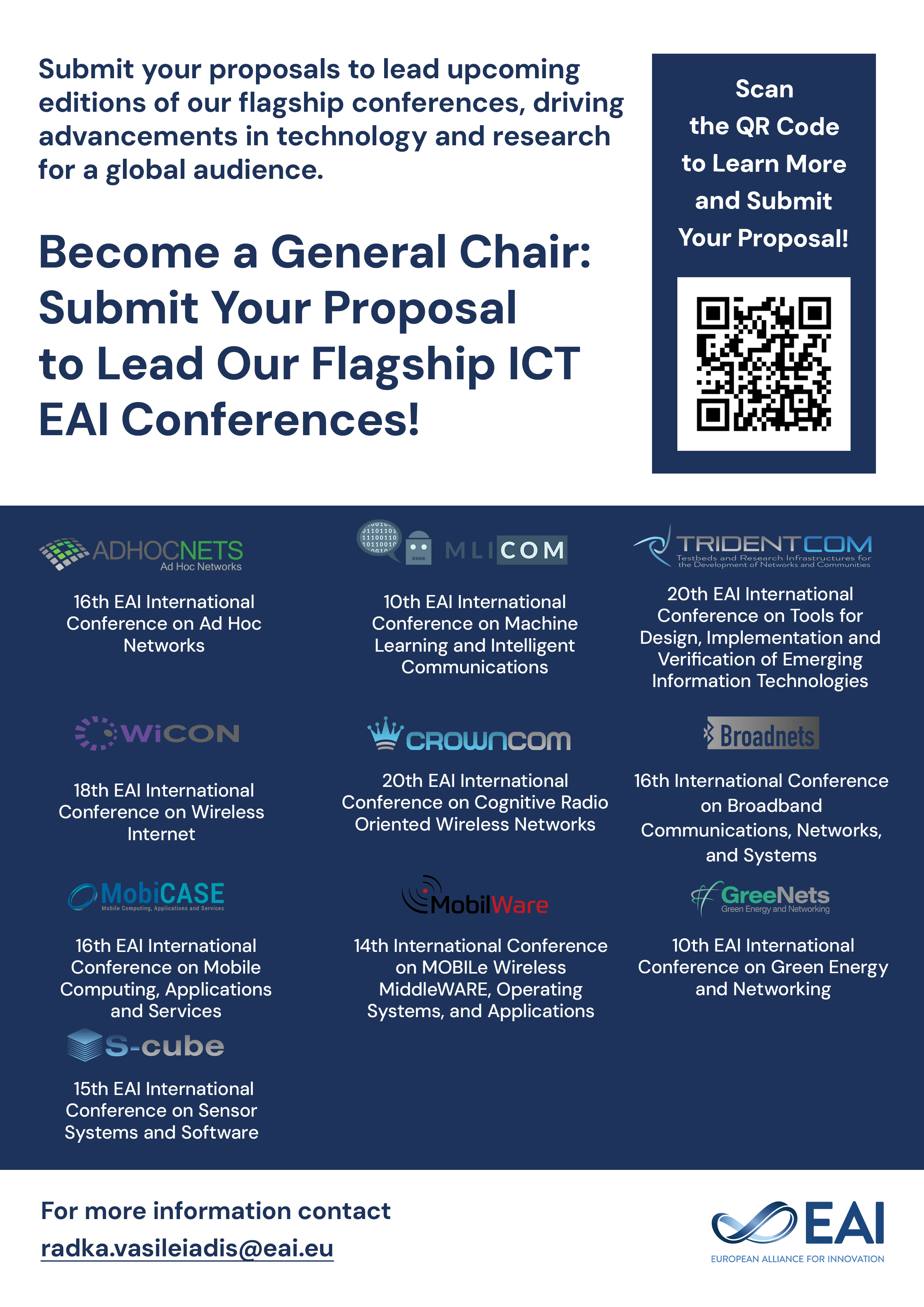
Research Article
Research on Equipment Fault Prediction Method Based on Industrial Big Data
@INPROCEEDINGS{10.4108/eai.17-6-2022.2322795, author={Zihan Qi and Chang Tang and Luli He and Changjian Jiang and Jing Chen and Yulin Huang}, title={Research on Equipment Fault Prediction Method Based on Industrial Big Data}, proceedings={Proceedings of the International Conference on Information Economy, Data Modeling and Cloud Computing, ICIDC 2022, 17-19 June 2022, Qingdao, China}, publisher={EAI}, proceedings_a={ICIDC}, year={2022}, month={10}, keywords={industrial data; data preprocessing; main feature extraction; equipment fault prediction; bpnn}, doi={10.4108/eai.17-6-2022.2322795} }
- Zihan Qi
Chang Tang
Luli He
Changjian Jiang
Jing Chen
Yulin Huang
Year: 2022
Research on Equipment Fault Prediction Method Based on Industrial Big Data
ICIDC
EAI
DOI: 10.4108/eai.17-6-2022.2322795
Abstract
Aiming at the problems of low accuracy and long prediction time of industrial equipment fault prediction, a prediction method based on main feature extraction and back propagation neural network (MFE-BPNN) was proposed. This method firstly preprocesses the missing, abnormal and high-noised industrial equipment data, then uses the method of recursive feature elimination combined with cross validation to extract the main feature variables, then designs the numbers of hidden layers and neurons, and weights of training and learning rates. This method improves the accuracy of industrial equipment fault prediction by preprocessing industrial data and establishing a prediction model based on a neural network. The prediction time is reduced by extracting the main characteristic variables. The experimental results of fan blade icing fault prediction in the field of power generation verify the effectiveness of this method.