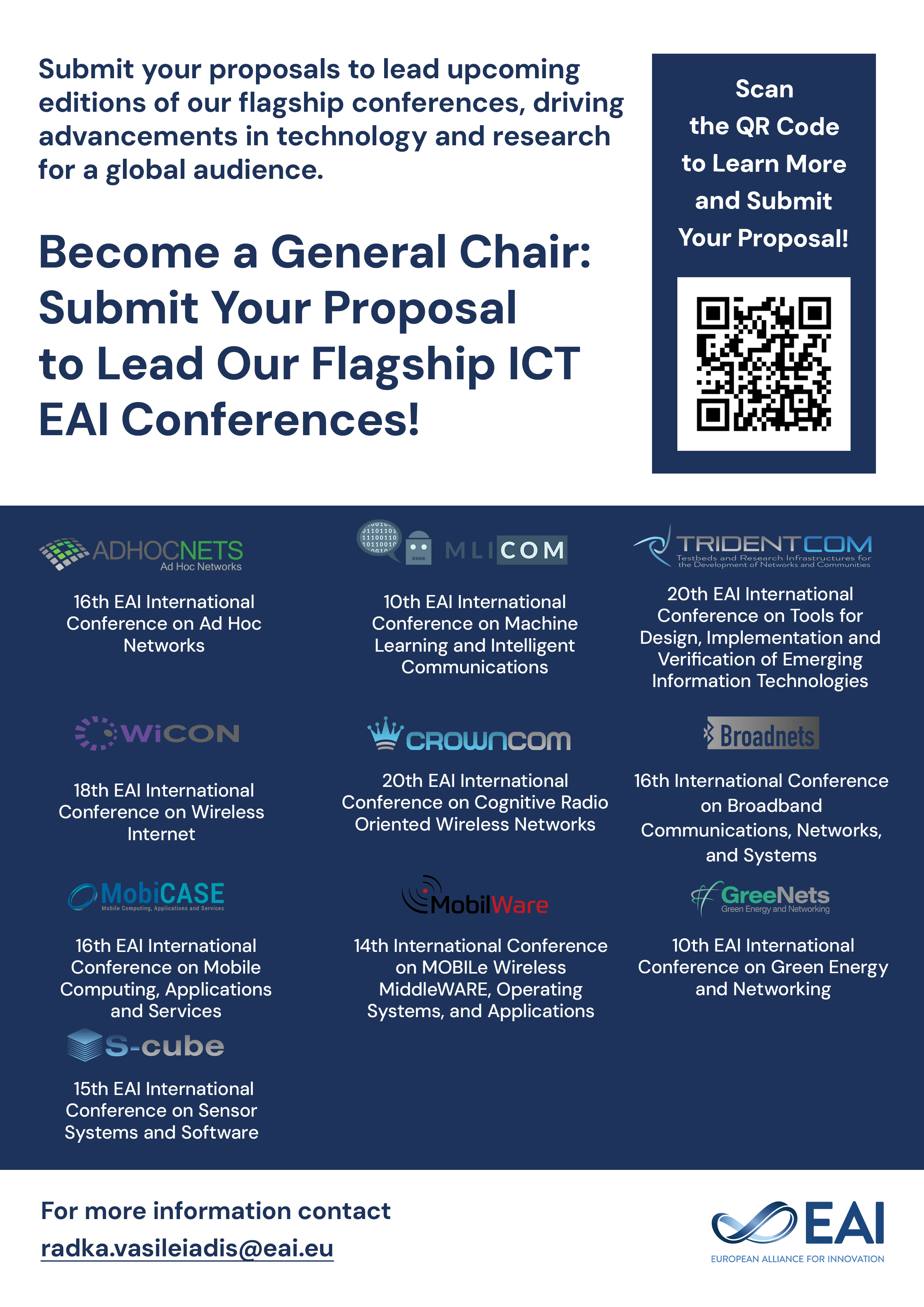
Research Article
GAN Data Augmentation for Improved Automated Atherosclerosis Screening from Coronary CT Angiography
@ARTICLE{10.4108/eai.17-5-2022.173981, author={Amel Laidi and Mohammed Ammar and Mostafa El Habib Daho and Said Mahmoudi}, title={GAN Data Augmentation for Improved Automated Atherosclerosis Screening from Coronary CT Angiography}, journal={EAI Endorsed Transactions on Scalable Information Systems}, volume={10}, number={1}, publisher={EAI}, journal_a={SIS}, year={2022}, month={5}, keywords={Atherosclerosis, CCTA, Transfer learning, Generative Adversarial Networks, GAN, Data augmentation}, doi={10.4108/eai.17-5-2022.173981} }
- Amel Laidi
Mohammed Ammar
Mostafa El Habib Daho
Said Mahmoudi
Year: 2022
GAN Data Augmentation for Improved Automated Atherosclerosis Screening from Coronary CT Angiography
SIS
EAI
DOI: 10.4108/eai.17-5-2022.173981
Abstract
INTRODUCTION: Atherosclerosis is a chronic medical condition that can result in coronary artery disease, strokes, or even heart attacks. early detection can result in timely interventions and save lives. OBJECTIVES: In this work, a fully automatic transfer learning-based model was proposed for Atherosclerosis detection in coronary CT angiography (CCTA). The model’s performance was improved by generating training data using a Generative Adversarial Network. METHODS: A first experiment was established on the original dataset with a Resnet network, reaching 95.2% accuracy, 60.8% sensitivity, 99.25% specificity and 90.48% PPV. A Generative Adversarial Network (GAN) was then used to generate a new set of images to balance the dataset, creating more positive images. Experiments were made adding from 100 to 1000 images to the dataset. RESULTS: adding 1000 images resulted in a small drop in accuracy to 93.2%, but an improvement in overall performance with 89.0% sensitivity, 97.37% specificity and 97.13% PPV. CONCLUSION: This paper was one of the early research projects investigating the efficiency of data augmentation using GANs for atherosclerosis, with results comparable to the state of the art.
Copyright © 2022 A. Laidi et al., licensed to EAI. This is an open access article distributed under the terms of the Creative Commons Attribution license, which permits unlimited use, distribution and reproduction in any medium so long as the original work is properly cited.