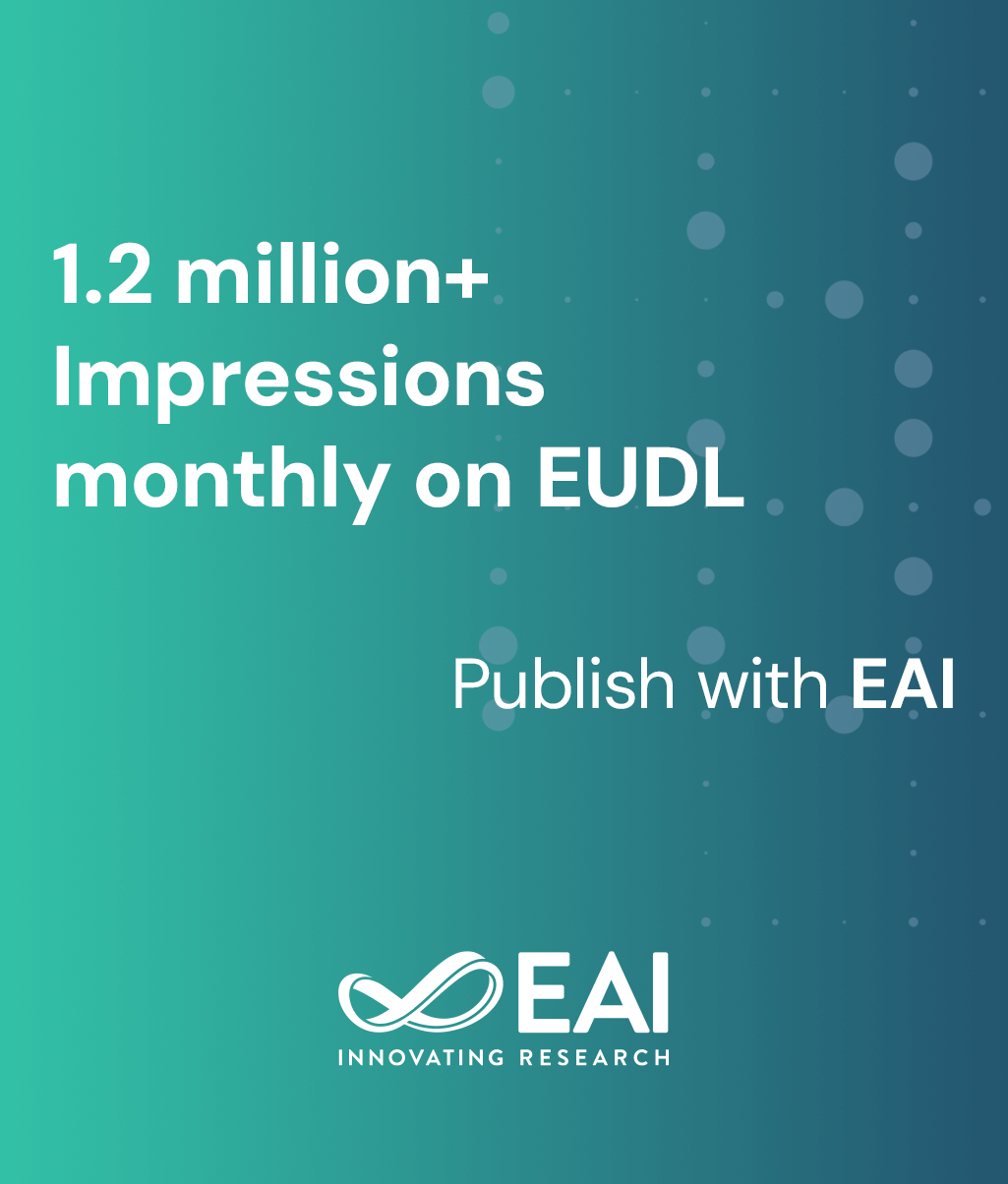
Research Article
Comparative Studies on Methods of Selecting Features in Credit Scoring
@INPROCEEDINGS{10.4108/eai.17-11-2023.2342728, author={Jingyao Li}, title={Comparative Studies on Methods of Selecting Features in Credit Scoring}, proceedings={Proceedings of the 5th International Conference on Economic Management and Model Engineering, ICEMME 2023, November 17--19, 2023, Beijing, China}, publisher={EAI}, proceedings_a={ICEMME}, year={2024}, month={2}, keywords={feature selection operations research machine learning}, doi={10.4108/eai.17-11-2023.2342728} }
- Jingyao Li
Year: 2024
Comparative Studies on Methods of Selecting Features in Credit Scoring
ICEMME
EAI
DOI: 10.4108/eai.17-11-2023.2342728
Abstract
Feature selection (FS) is a critical process in credit scoring, which can improve models, address overfitting, decrease computational cost, and increase model interpretability. This paper presents a classification of research problems and methods related to the above issues. The research problems can be divided into two types based on focus: model development or evaluation, and two types based on methodology: machine learning or operations research. The machine learning research objects can be divided into four types based on the feature of training and type of optimization. The paper also provides system factors and evaluation metrics of the experiments for FS in credit scoring. The system factors include personal information, credit history, type of loan, loan amount, and loan purpose. The evaluation metrics include accuracy, precision, recall, F1 score, and AUC.