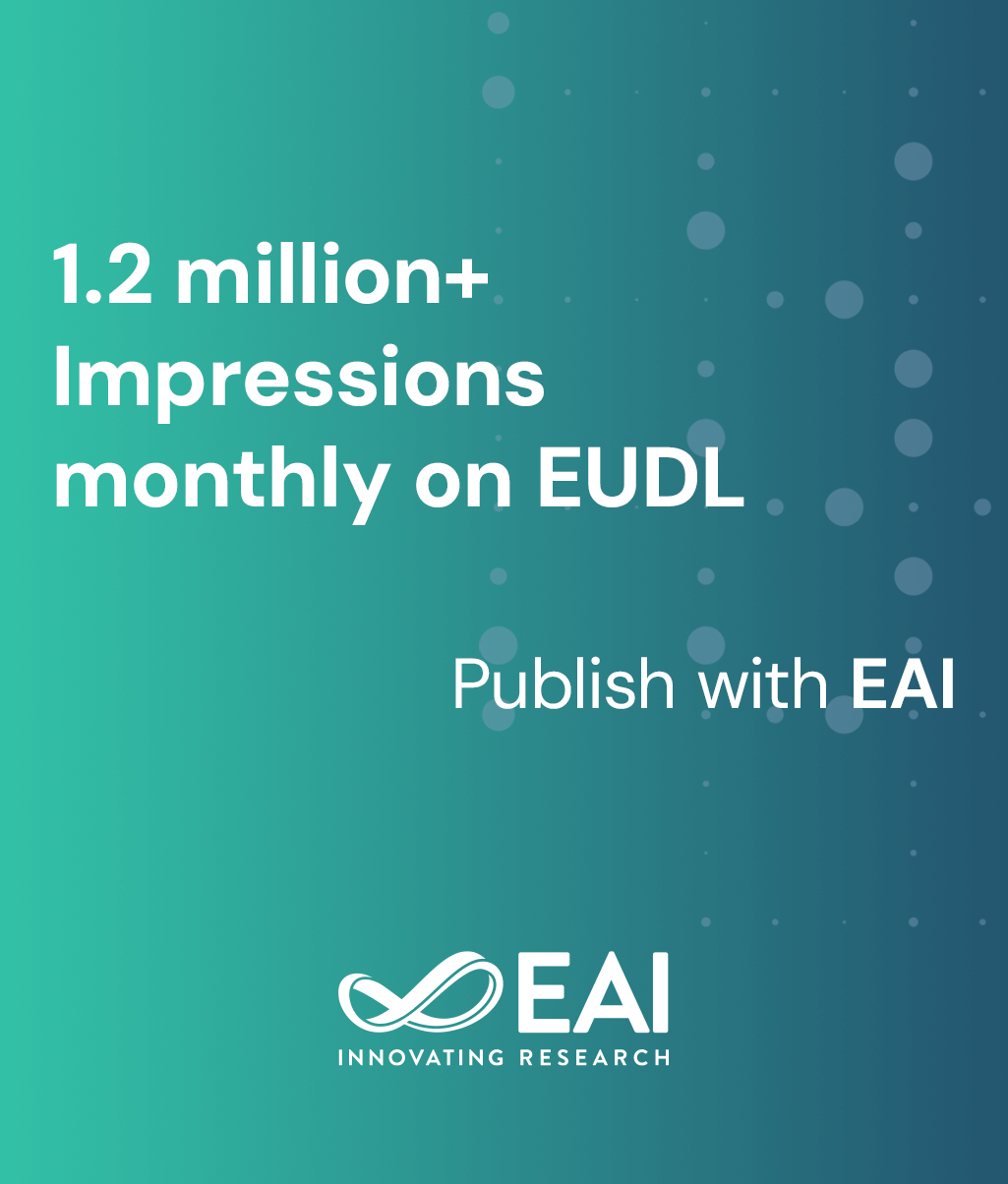
Research Article
Research on vehicle multi-classification object detection algorithm based on Ultralytics/YOLOv5 improvement
@INPROCEEDINGS{10.4108/eai.17-1-2025.2355328, author={Yu Deng}, title={Research on vehicle multi-classification object detection algorithm based on Ultralytics/YOLOv5 improvement}, proceedings={Proceedings of the 4th International Conference on Computing Innovation and Applied Physics, CONF-CIAP 2025, 17-23 January 2025, Eskişehir, Turkey}, publisher={EAI}, proceedings_a={CONF-CIAP}, year={2025}, month={4}, keywords={vehicle multi-classification object detection ultralytics/yolov5 deep learning real-time detection}, doi={10.4108/eai.17-1-2025.2355328} }
- Yu Deng
Year: 2025
Research on vehicle multi-classification object detection algorithm based on Ultralytics/YOLOv5 improvement
CONF-CIAP
EAI
DOI: 10.4108/eai.17-1-2025.2355328
Abstract
This paper investigates an improved vehicle multi-classification object detection algorithm based on Ultralytics/YOLOv5. By combining the YOLOv5 model with a custom dataset, training and testing were conducted on Bangladeshi road vehicle images. The main contributions of this paper include optimizing the hyperparameter settings of the model to adapt to the vehicle multi-classification problem and improving detection performance by adjusting input sizes and the number of categories. The training set contains 2704 images, the validation set contains 300 images, covering 21 classes of vehicles. Experimental results show that the improved algorithm performs well in terms of mAP, Precision, and Recall values, with an average mAP of 0.41 and Precision of 0.669 on the test set. The research results of this paper demonstrate that the improved YOLOv5 model exhibits high accuracy and robustness in vehicle detection tasks in complex traffic environments, providing strong support for intelligent traffic monitoring and autonomous driving.