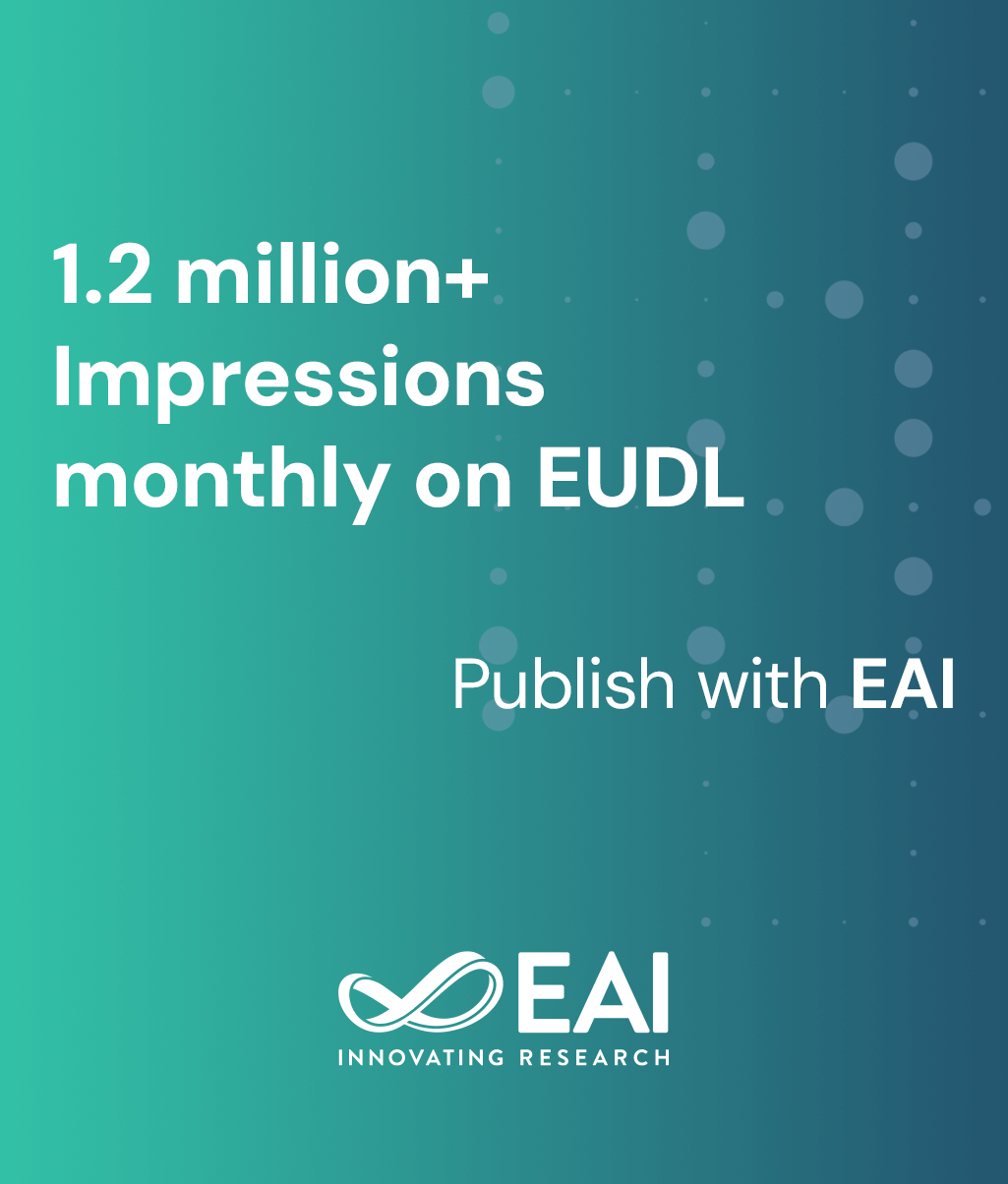
Research Article
Predicting Patient Waiting Time and Detecting Overload in Emergency Department through Machine Learning
@INPROCEEDINGS{10.4108/eai.17-1-2025.2355323, author={Zihan Qian and Xuanyi Shen and Rongshuo Shang}, title={Predicting Patient Waiting Time and Detecting Overload in Emergency Department through Machine Learning}, proceedings={Proceedings of the 4th International Conference on Computing Innovation and Applied Physics, CONF-CIAP 2025, 17-23 January 2025, Eskişehir, Turkey}, publisher={EAI}, proceedings_a={CONF-CIAP}, year={2025}, month={4}, keywords={patient waiting time emergency department regression model machine learn ing deep learning overload detection}, doi={10.4108/eai.17-1-2025.2355323} }
- Zihan Qian
Xuanyi Shen
Rongshuo Shang
Year: 2025
Predicting Patient Waiting Time and Detecting Overload in Emergency Department through Machine Learning
CONF-CIAP
EAI
DOI: 10.4108/eai.17-1-2025.2355323
Abstract
Accurately predicting patient waiting times and detecting workflow overload in emergency departments are critical challenges that significantly impact patient care and resource management. Despite advancements in patient waiting time prediction, current methodologies often struggle with universal applicability in practical settings and fail to accurately capture extreme values. This study proposes a robust and generalized predictive model tailored to the specific challenges of ED workflows to address these gaps. A dataset containing multiple variables that record the workflow within an emergency department is utilized, and a systematic exploration and comparison of specific machine learning and deep learning models are conducted. Different machine learning models are compared, and a model is developed to enhance the accuracy of prediction. The effectiveness of the model in detecting ED overload is evaluated, and its generalization capability is improved through feature selection and feature classification. The proposed model demonstrates superior accuracy in predicting patient waiting times and exhibits high sensitivity in detecting workflow bottlenecks. The model’s ability to operate effectively with fewer variables enhances its generalization ability across different ED facilities. It is found that machine learning models can effectively capture patient waiting peaks, which are critical indicators of ED overload.