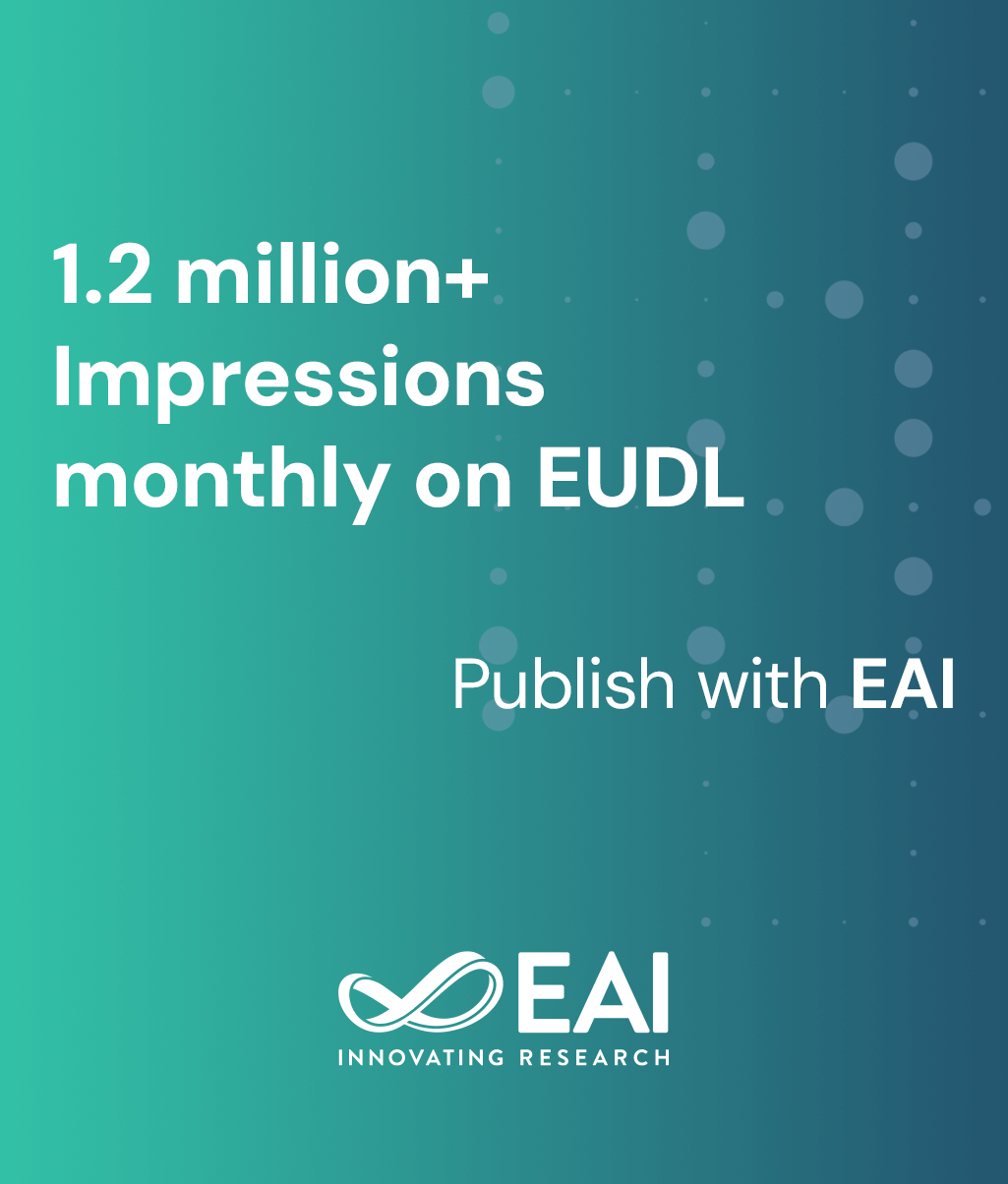
Research Article
Vulnerability and Defense: Mitigating Backdoor Attacks in Deep Learning-Based Crowd Counting Models
@INPROCEEDINGS{10.4108/eai.17-1-2025.2355235, author={Jinzi Luo}, title={Vulnerability and Defense: Mitigating Backdoor Attacks in Deep Learning-Based Crowd Counting Models}, proceedings={Proceedings of the 4th International Conference on Computing Innovation and Applied Physics, CONF-CIAP 2025, 17-23 January 2025, Eskişehir, Turkey}, publisher={EAI}, proceedings_a={CONF-CIAP}, year={2025}, month={4}, keywords={crowd counting deep learning backdoor attack defense}, doi={10.4108/eai.17-1-2025.2355235} }
- Jinzi Luo
Year: 2025
Vulnerability and Defense: Mitigating Backdoor Attacks in Deep Learning-Based Crowd Counting Models
CONF-CIAP
EAI
DOI: 10.4108/eai.17-1-2025.2355235
Abstract
Crowd counting aims to infer the number of people or objects in an image through different methods. It is widely used in surveillance, sensitive events, etc., and plays a vital role in a series of security- critical applications. Most of the state-of-the-art crowd counting models are based on deep learning, which are very efficient and accurate in handling dense scenes. Although such models are effective, they are still vulnerable to backdoor attacks. Attackers can compromise model accuracy by poisoning surveillance data or using global triggers, leading to inaccurate crowd counts. In this paper, we verify the vulnerability of deep learning-based crowd counting models to backdoor attacks and prove the effectiveness of density manipulation attacks on two different types of crowd counting models. At the same time, a defense method similar to fine-tuning is proposed based on this backdoor attack. Through in-depth analysis, we observe that our defense method not only reduces the effectiveness of backdoor attacks – the attack success rate ρAsr by 72.5%, but also improves the accuracy of the original model’s prediction – the accuracy ρAcc by 66.5%. Our work can help eliminate potential backdoor attacks on crowd counting models.