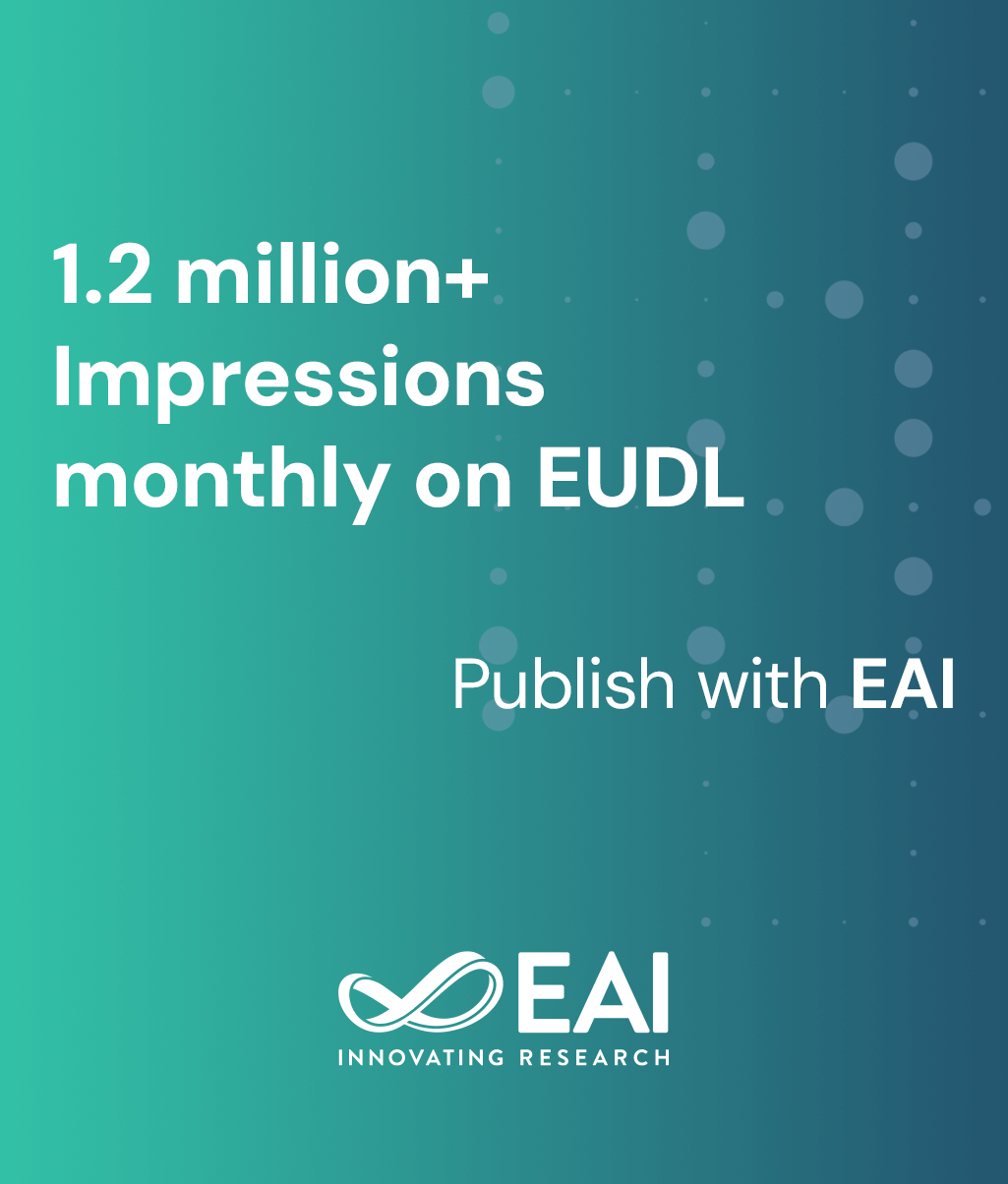
Research Article
Deep Learning based MURA Defect Detection
@ARTICLE{10.4108/eai.16-7-2019.162217, author={Ramya Bagavath Singh and Gaurav Kumar and Gaurav Sultania and Shashank Shrikant Agashe and Priya Ranjan Sinha and Chulmoo Kang}, title={Deep Learning based MURA Defect Detection}, journal={EAI Endorsed Transactions on Cloud Systems}, volume={5}, number={15}, publisher={EAI}, journal_a={CS}, year={2019}, month={7}, keywords={MURA defect, Automatic Defect Classification and Localization, Deep Learning}, doi={10.4108/eai.16-7-2019.162217} }
- Ramya Bagavath Singh
Gaurav Kumar
Gaurav Sultania
Shashank Shrikant Agashe
Priya Ranjan Sinha
Chulmoo Kang
Year: 2019
Deep Learning based MURA Defect Detection
CS
EAI
DOI: 10.4108/eai.16-7-2019.162217
Abstract
INTRODUCTION: MURA defects in LED/LCD panels are one of the most challenging defects for Automatic Defect Classification and Localization (ADC) due to their extremely low contrast when compared with the background. Manual detection is subjective, error prone, very tedious and time consuming. Even when the type of MURA defects can be ascertained manually, the exact bounding box for defect is hard to determine. Various heuristic based image processing techniques have been applied giving sub-optimal accuracy over generic datasets.
OBJECTIVES: The primary objective of this paper is to check whether the state of the art DL (Deep Learning) network for general object classification and localization (MSCOCO PASCAL VOC etc.) can be applied successfully for MURA Defect Classification and Localization.
METHODS: In this paper we present a single DL pipeline for classification and localization which for the first time is applied for MURA defects. Naive DL network - Single Shot multi-box Detector (SSD, pre-trained on ImageNet) was not sufficient to give a good F1 score because of the nature of the defect. Accuracy improved a little after applying various DL specific optimization methods such as loss function optimization, network optimization etc. Utilizing the knowledge from MURA domain for data augmentation, like filtering based on image capture wavelength etc. improved the results significantly.
RESULTS: Using optimization techniques that are from both DL domain as well as specific to MURA domain, we show improvement in the accuracy of the base DL pipeline from ~30% to ~80%. Minimum heuristics were used to define the pipeline so that it can easily adapt to any new MURA dataset. The paper shows the importance of domain specific preprocessing steps for the designed network in case of MURA defects.
CONCLUSION: Using DL, MURA classification and localization had not been tried before. For the first time we demonstrated results for both classification and localization of MURA defects using state-of-the-art DL network with F1~80%. We also conclude that state-of-the-art network for general object detection can be reused with the help of Transfer Learning (TL) concept and fine-tuned with MURA domain specific optimizations mentioned in paper for optimal performances in MURA domain.
Copyright © 2019 Ramya Bagavath Singh et al., licensed to EAI. This is an open access article distributed under the terms of the Creative Commons Attribution licence (http://creativecommons.org/licenses/by/3.0/), which permits unlimited use, distribution and reproduction in any medium so long as the original work is properly cited.