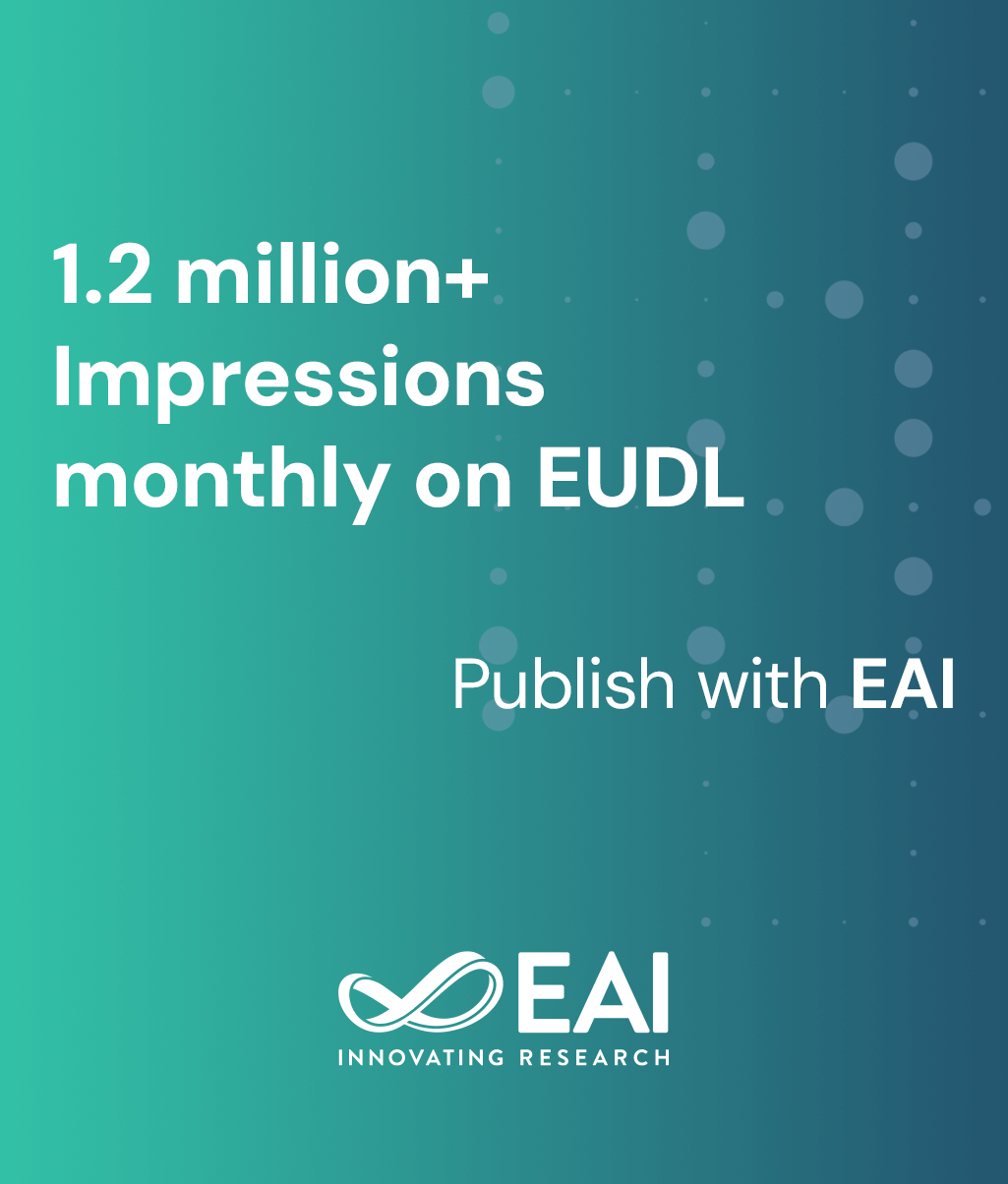
Research Article
Performance Evaluation of Word Embeddings for Sarcasm Detection- A Deep Learning Approach
@INPROCEEDINGS{10.4108/eai.16-5-2020.2303935, author={Annie Annie Johnson and R. Karthik}, title={Performance Evaluation of Word Embeddings for Sarcasm Detection- A Deep Learning Approach}, proceedings={Proceedings of the First International Conference on Advanced Scientific Innovation in Science, Engineering and Technology, ICASISET 2020, 16-17 May 2020, Chennai, India}, publisher={EAI}, proceedings_a={ICASISET}, year={2021}, month={1}, keywords={sarcasm deep learning word embedding sentiment analysis}, doi={10.4108/eai.16-5-2020.2303935} }
- Annie Annie Johnson
R. Karthik
Year: 2021
Performance Evaluation of Word Embeddings for Sarcasm Detection- A Deep Learning Approach
ICASISET
EAI
DOI: 10.4108/eai.16-5-2020.2303935
Abstract
Sarcasm detection is a critical step to sentiment analysis, with the aim of understanding, and exploiting the available information from platforms such as social media. The accuracy of sarcasm detection depends on the nature of word embeddings. This research performs a comprehensive analysis of different word embedding techniques for effective sarcasm detection. A hybrid combination of optimizers including; the Adaptive Moment Estimation (Adam), the Adaptive Gradient Algorithm (AdaGrad) and Adadelta functions and activation functions like Rectified Linear Unit (ReLU) and Leaky ReLU have been experimented with.Different word embedding techniques including; Bag of Words (BoW), BoW with Term Frequency–Inverse Document Frequency (TF-IDF), Word2vec and Global Vectors for word representation (GloVe) are evaluated. The highest accuracy of 61.68% was achieved with theGloVeembeddings and an AdaGrad optimizer and a ReLU activation function, used in the deep learning model.