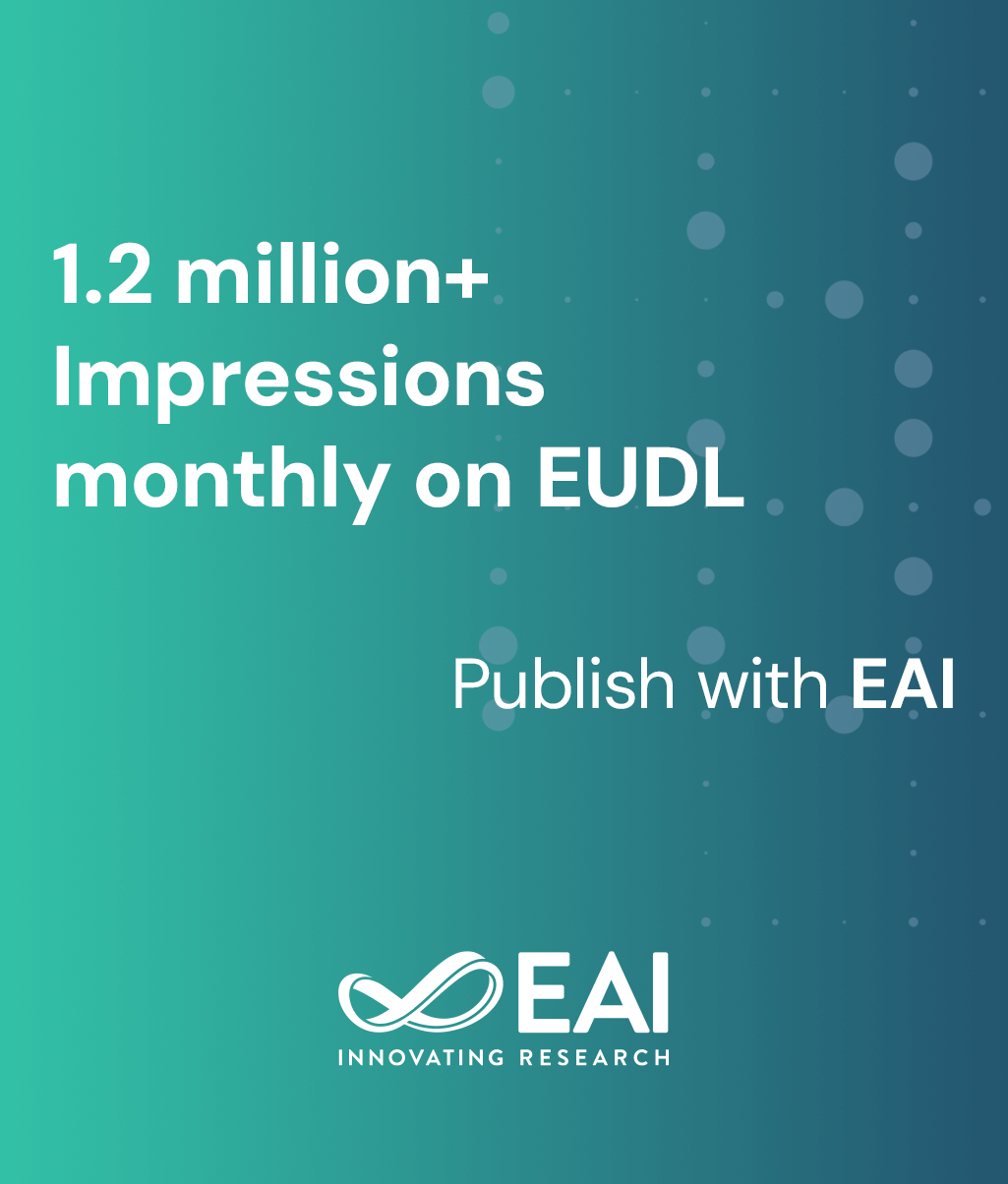
Research Article
Transit Signal Priority Control Method Based on Deep Reinforcement Learning
@INPROCEEDINGS{10.4108/eai.15-3-2024.2346425, author={Junnan Chen and Xufei Zhuang and Heng Li and Chenxi Yang}, title={Transit Signal Priority Control Method Based on Deep Reinforcement Learning}, proceedings={Proceedings of the 4th International Conference on Public Management and Intelligent Society, PMIS 2024, 15--17 March 2024, Changsha, China}, publisher={EAI}, proceedings_a={PMIS}, year={2024}, month={6}, keywords={intelligent transportation; transit signal priority control; deep reinforcement learning; sumo simulation}, doi={10.4108/eai.15-3-2024.2346425} }
- Junnan Chen
Xufei Zhuang
Heng Li
Chenxi Yang
Year: 2024
Transit Signal Priority Control Method Based on Deep Reinforcement Learning
PMIS
EAI
DOI: 10.4108/eai.15-3-2024.2346425
Abstract
Transit signal priority control plays a pivotal role in enhancing the efficiency of public transportation and mitigating traffic congestion. Despite advancements in current research on transit signal priority control using deep reinforcement learning, the field is still nascent, encountering challenges like limited model generalization and slow training convergence, particularly in intricate traffic environments. This paper proposes a transit signal priority control model based on deep reinforcement learning. The model utilizes a deep neural network framework, integrating crucial optimizations like Dueling DQN, Distributional DQN and the PER mechanism, alongside enhancements to the loss function, to accommodate diverse traffic scenarios. The model incorporates essential information at intersections, encompassing vehicle positions, velocities, and lane conditions as input states to comprehensively depict the traffic situation. Concurrently, by delineating a set of signal phase configurations at intersections, a reward function is devised that considers various factors, such as intersection passage efficiency, bus delays, and passenger experience. This guides the agent to optimize the entire system throughout the learning process, duly considering signal control strategies to ensure effective decision-making.