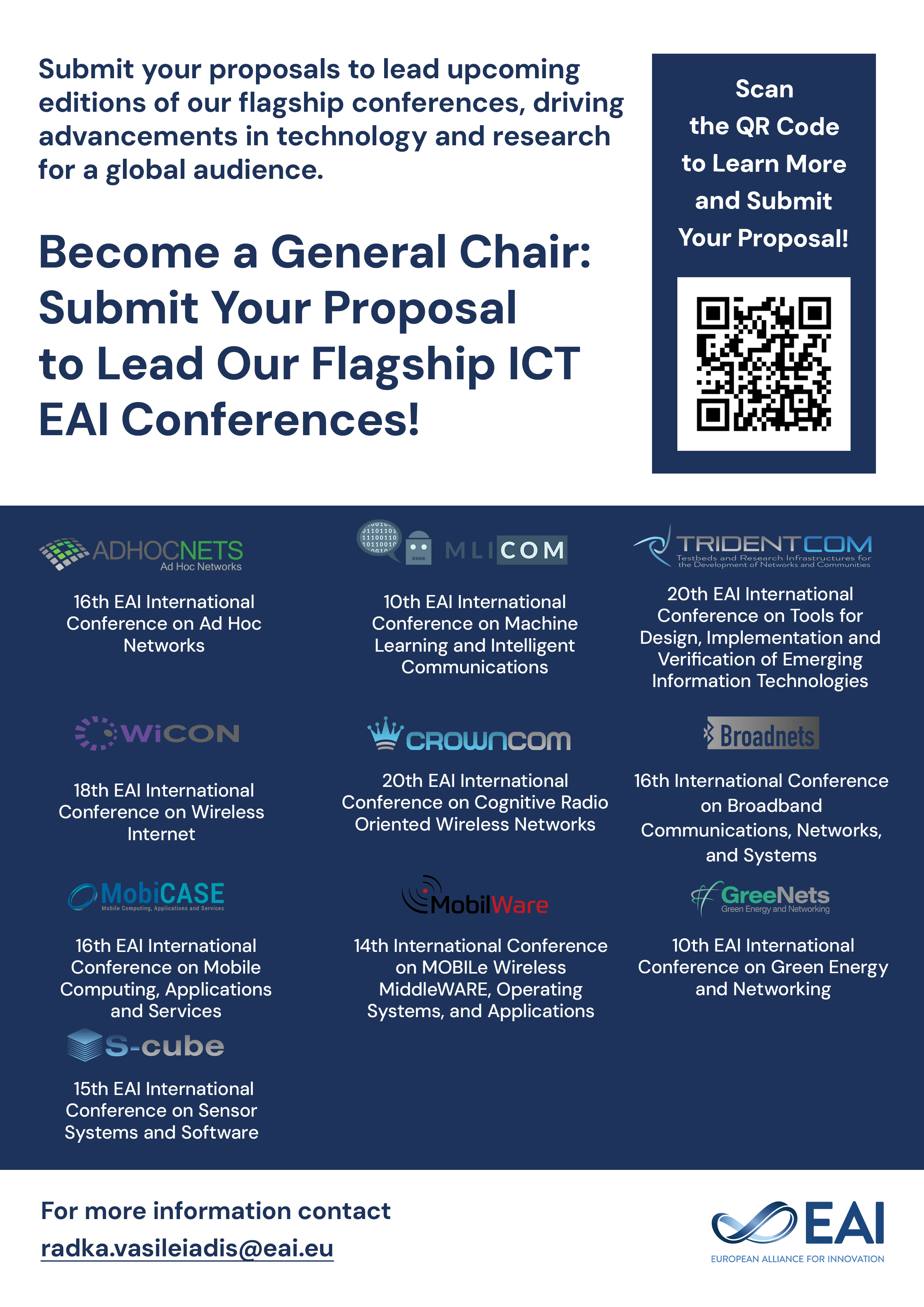
Research Article
A Multi-Layer Feature Low-Rank Fusion Algorithm Using Social Media for Disaster Information Detection
@INPROCEEDINGS{10.4108/eai.15-12-2023.2345422, author={Bonan Li and Honglu Cheng and Jinyan Zhou and Rui Cao and Hong Zhang and Xingang Wang}, title={A Multi-Layer Feature Low-Rank Fusion Algorithm Using Social Media for Disaster Information Detection}, proceedings={Proceedings of the 3rd International Conference on Public Management and Big Data Analysis, PMBDA 2023, December 15--17, 2023, Nanjing, China}, publisher={EAI}, proceedings_a={PMBDA}, year={2024}, month={5}, keywords={multi-modal; social media; low-rank fusion; multi-layer feature extraction}, doi={10.4108/eai.15-12-2023.2345422} }
- Bonan Li
Honglu Cheng
Jinyan Zhou
Rui Cao
Hong Zhang
Xingang Wang
Year: 2024
A Multi-Layer Feature Low-Rank Fusion Algorithm Using Social Media for Disaster Information Detection
PMBDA
EAI
DOI: 10.4108/eai.15-12-2023.2345422
Abstract
When disasters occur, people use social media to post in real time, which includes rich text and visual images. Relevant authorities can use this information to make emergency decisions and public opinion analysis quickly. However, the high complexity of multimodal deep learning models cannot meet the high timeliness requirements of disaster analysis. In addition, social media multimodal datasets for disaster detection are often scarce, and simple features are not sufficient to adequately provide usable information for analysis models. To mitigate these concerns, this paper introduces a model for low-level fusion called the Multilayer Feature Low-Level Fusion Model (MLLMF). The model uses transfer learning pre-trained models to extract text features from different hidden layers instead of traditional single-layer text features, and introduces gate attention units (GAUs) to enhance each modal feature to fully extract the intrinsic information of each single modality so that the information of small sample data can be utilized to the maximum. Moreover, to tackle the challenge posed by the high complexity of multimodal models, this paper uses low-rank tensor for multimodal fusion of text and images. With low model complexity, not only the unique information of a single modality is retained, but also the correlation between different modal elements is exploited to achieve a balance between low model complexity and high accuracy.