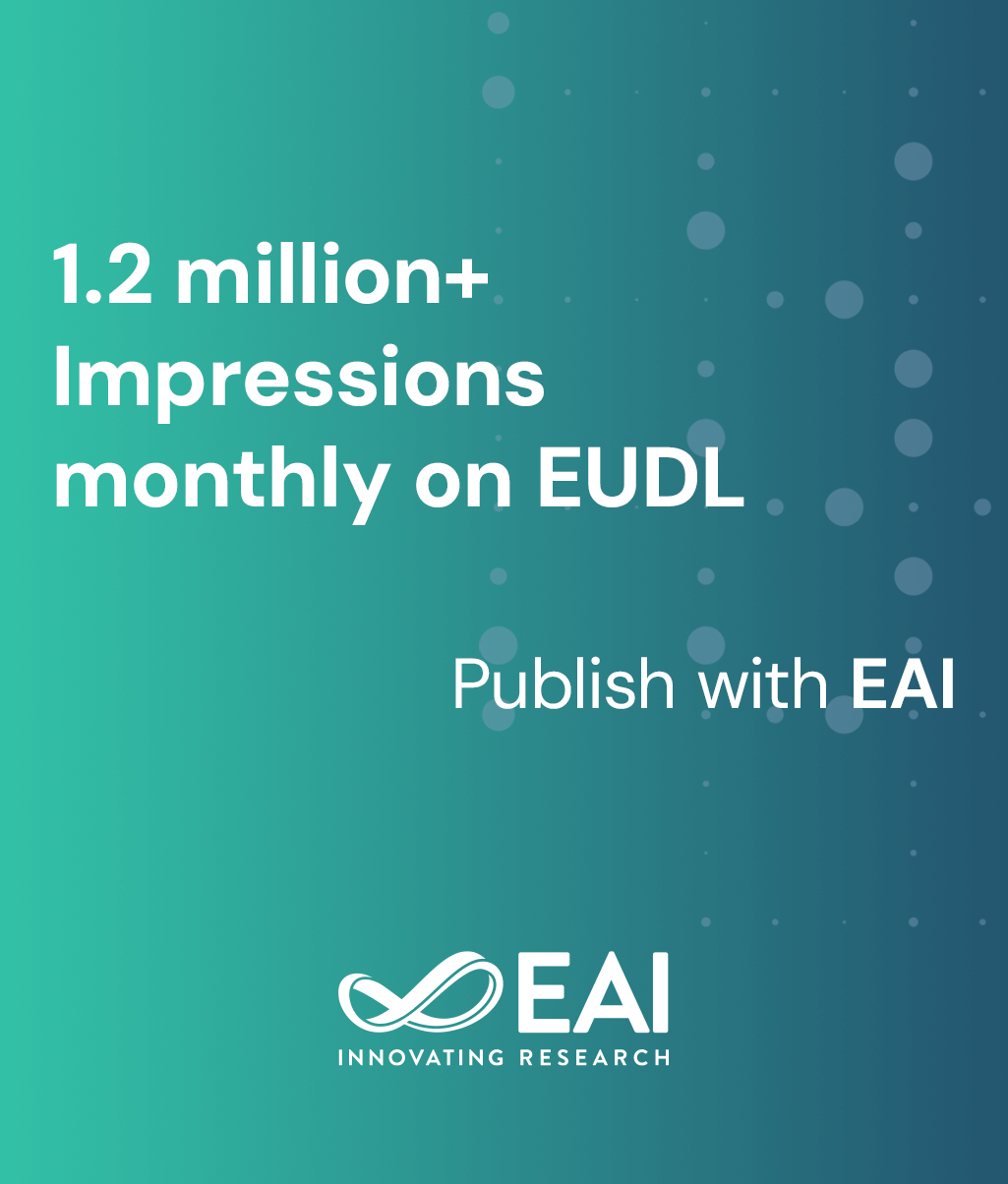
Research Article
Path Selection Logit Model Introducing Influence of Regret on Relative Utility Difference
@INPROCEEDINGS{10.4108/eai.15-12-2023.2345415, author={Zhong Wu and Lidan Ying and Haifei Yang}, title={Path Selection Logit Model Introducing Influence of Regret on Relative Utility Difference}, proceedings={Proceedings of the 3rd International Conference on Public Management and Big Data Analysis, PMBDA 2023, December 15--17, 2023, Nanjing, China}, publisher={EAI}, proceedings_a={PMBDA}, year={2024}, month={5}, keywords={transportation planning and management; psychology of regret; path selection; generalized logit model}, doi={10.4108/eai.15-12-2023.2345415} }
- Zhong Wu
Lidan Ying
Haifei Yang
Year: 2024
Path Selection Logit Model Introducing Influence of Regret on Relative Utility Difference
PMBDA
EAI
DOI: 10.4108/eai.15-12-2023.2345415
Abstract
This study extends the conventional stochastic regret minimization model and incorporates it into the path choice utility function as an expected regret psychological influence factor. This happens because of the limited explanatory capacity of the purely rational path choice-based model regarding actual travel. The weakness in the classic model's inference of the intensity of regret is fixed by the updated model, which employs the utility difference rather than the actual amount of the utility value. Two IIA characteristics—the path objective utility and the subjective regret psychological intensity brought on by the absolute utility difference—can be lessened by combining the improved utility function with the generalized logit model. The outcomes of the arithmetic examples demonstrate that the new model more accurately captures the influence of subjective regret psychology in path selection while resolving the IIA problems of the logit model. Furthermore, the enhanced model may simultaneously address the distributional implications of the random utility maximization and random regret minimization models, improving its capacity to elucidate actual choice behavior. When calibrated and applied to conventional network arithmetic allocation, the enhanced model's accuracy can reach 9.85%, capturing the network traffic flow allocation raw more accurately.