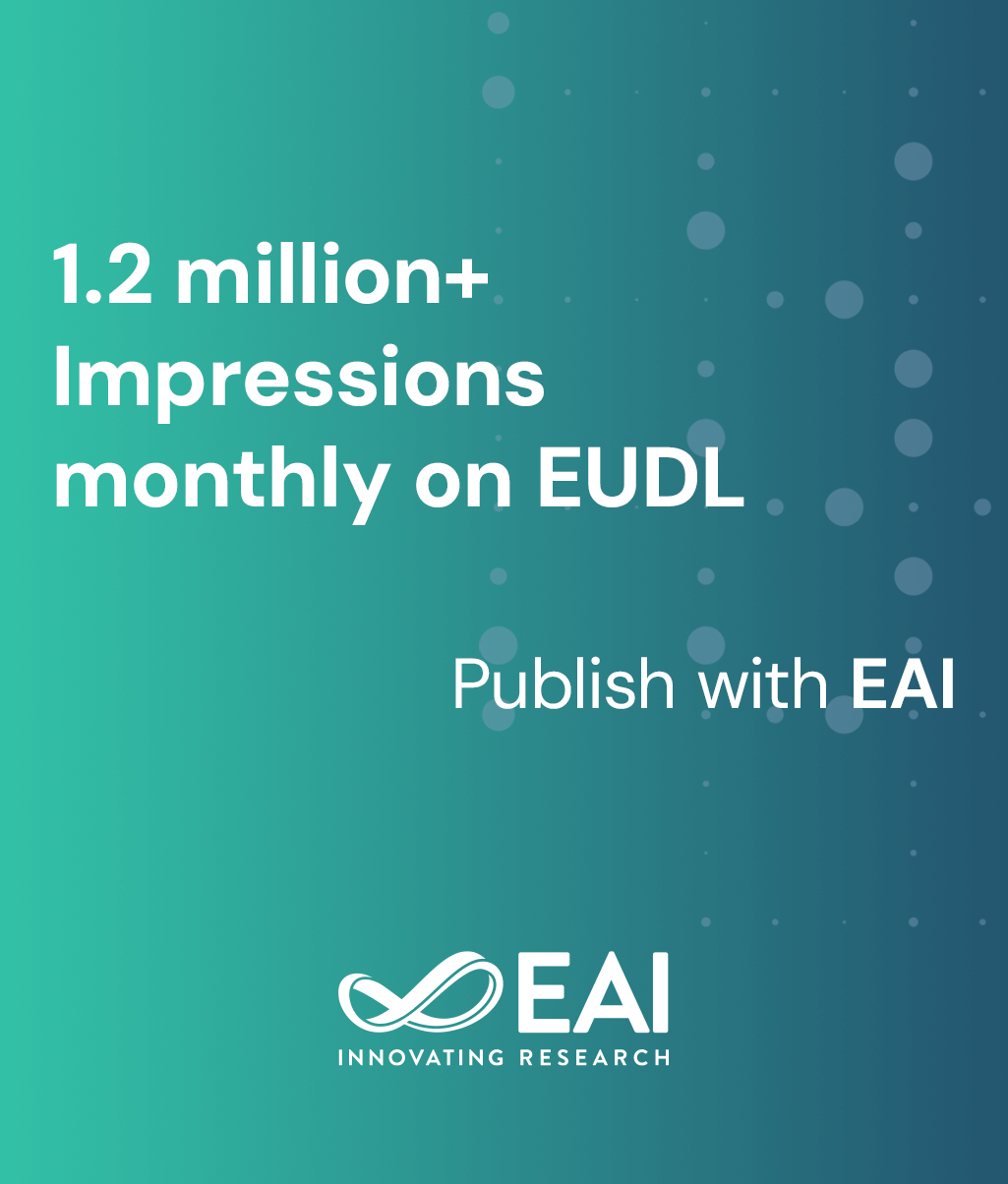
Research Article
Carbon Market Price Forecasting in China Using Probability Density Recurrent Networks
@INPROCEEDINGS{10.4108/eai.15-12-2023.2345396, author={Zijie Wei and Yongfei Hu and Pengyu Wang and Zhixin Han and Qianjiao Xie}, title={Carbon Market Price Forecasting in China Using Probability Density Recurrent Networks}, proceedings={Proceedings of the 3rd International Conference on Public Management and Big Data Analysis, PMBDA 2023, December 15--17, 2023, Nanjing, China}, publisher={EAI}, proceedings_a={PMBDA}, year={2024}, month={5}, keywords={time series; probability density recurrent networks; carbon price forecasting}, doi={10.4108/eai.15-12-2023.2345396} }
- Zijie Wei
Yongfei Hu
Pengyu Wang
Zhixin Han
Qianjiao Xie
Year: 2024
Carbon Market Price Forecasting in China Using Probability Density Recurrent Networks
PMBDA
EAI
DOI: 10.4108/eai.15-12-2023.2345396
Abstract
With the nationwide proliferation of carbon markets, the scarcity of carbon emissions allowances has become comparable to other commodities in traditional markets, endowing them with unique market value. Carbon prices play a central role in carbon market mechanisms, reflecting the outcomes of market competition and shaping government policies related to carbon emissions allocation. Consequently, the accurate prediction of carbon prices is essential for businesses to comprehend fluctuations in carbon prices, efficiently manage carbon emissions, and provide a sound foundation for trading decisions. In this study, we address data uncertainty by developing a probability density recurrent network. We investigate the intricate interrelationships and evolving patterns among data points using probability density distribution functions in time series data, enabling the creation of a prediction model to anticipate future data points. Multiple evaluation criteria corroborate the precision and effectiveness of the methodology employed in this research.