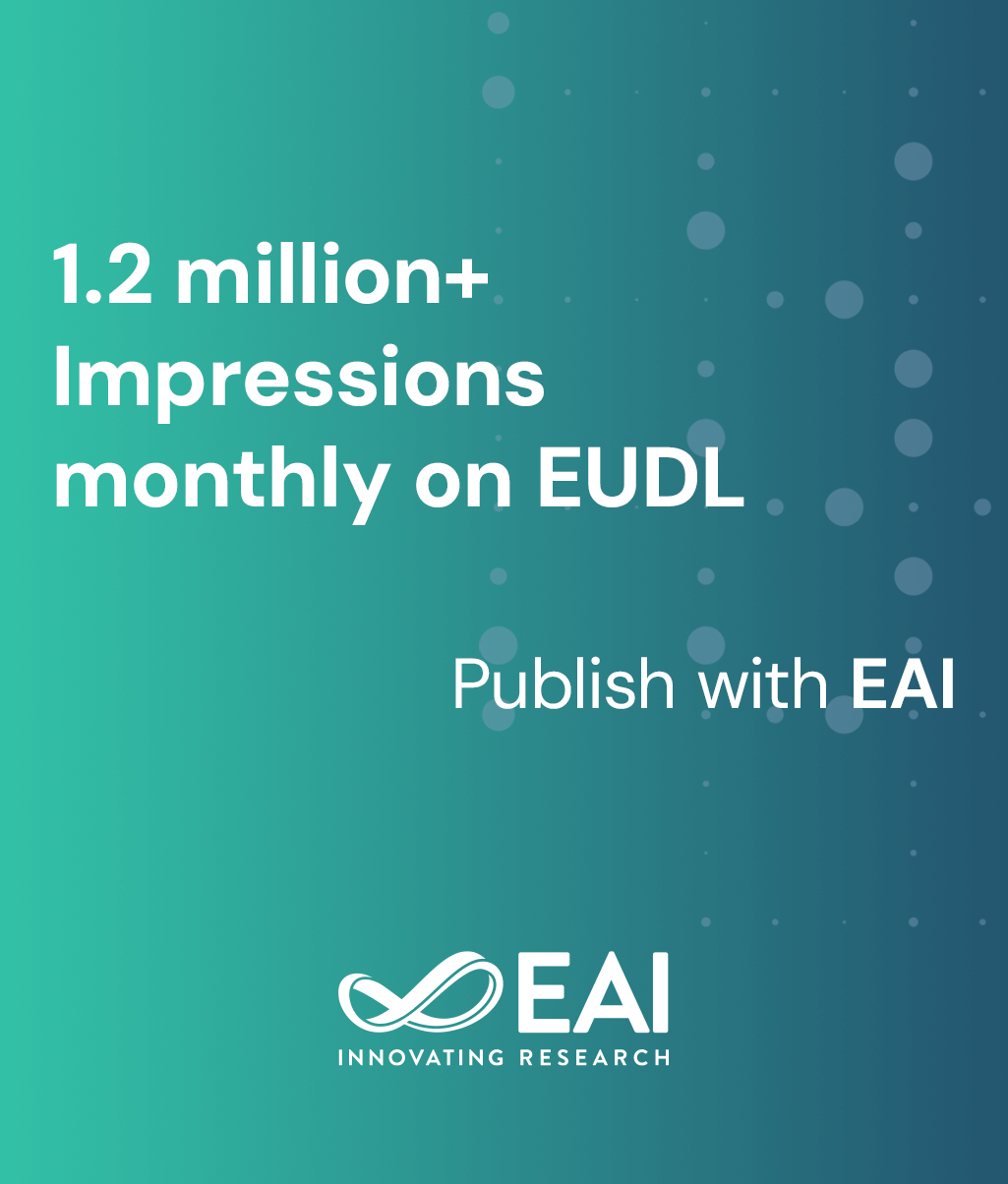
Research Article
Spatio-temporal Prediction of Air Quality using Distance Based Interpolation and Deep Learning Techniques
@ARTICLE{10.4108/eai.15-1-2021.168139, author={K. Krishna Rani Samal and Korra Sathya Babu and Santos Kumar Das}, title={Spatio-temporal Prediction of Air Quality using Distance Based Interpolation and Deep Learning Techniques}, journal={EAI Endorsed Transactions on Smart Cities}, volume={5}, number={14}, publisher={EAI}, journal_a={SC}, year={2021}, month={1}, keywords={Air quality, Deep Learning, LSTM, Inverse Distance Weighting, Spatio-temporal prediction}, doi={10.4108/eai.15-1-2021.168139} }
- K. Krishna Rani Samal
Korra Sathya Babu
Santos Kumar Das
Year: 2021
Spatio-temporal Prediction of Air Quality using Distance Based Interpolation and Deep Learning Techniques
SC
EAI
DOI: 10.4108/eai.15-1-2021.168139
Abstract
The harmful impact of air pollution has drawn raising concerns from ordinary citizens, researchers, policy makers, and smart city users. It is of great importance to identify air pollution levels at the spatial resolution on time so that its negative impact on human health and environment can be minimized. This paper proposed the CNN-BILSTM-IDW model, which aims to predict and spatially analyze the pollutant levelin the study area in advance using past observations. The neural network-based Convolutional Bidirectional Long short-term memory (CNN-BILSTM) network is employed to perform time series prediction over the next four weeks. Inverse Distance Weighting (IDW) is utilized to perform spatial prediction. The proposed CNN-BILSTM-IDW model provides almost 16% better prediction performance than the ordinary IDW method, which fails to predict spatial prediction at a high temporal period. The results of the presented comparative analysis signify the efficiency of the proposed model.
Copyright © 2021 K. Krishna Rani Samal et al., licensed to EAI. This is an open access article distributed under the terms of the Creative Commons Attribution license (http://creativecommons.org/licenses/by/3.0/), which permits unlimited use, distribution and reproduction in any medium so long as the original work is properly cited.