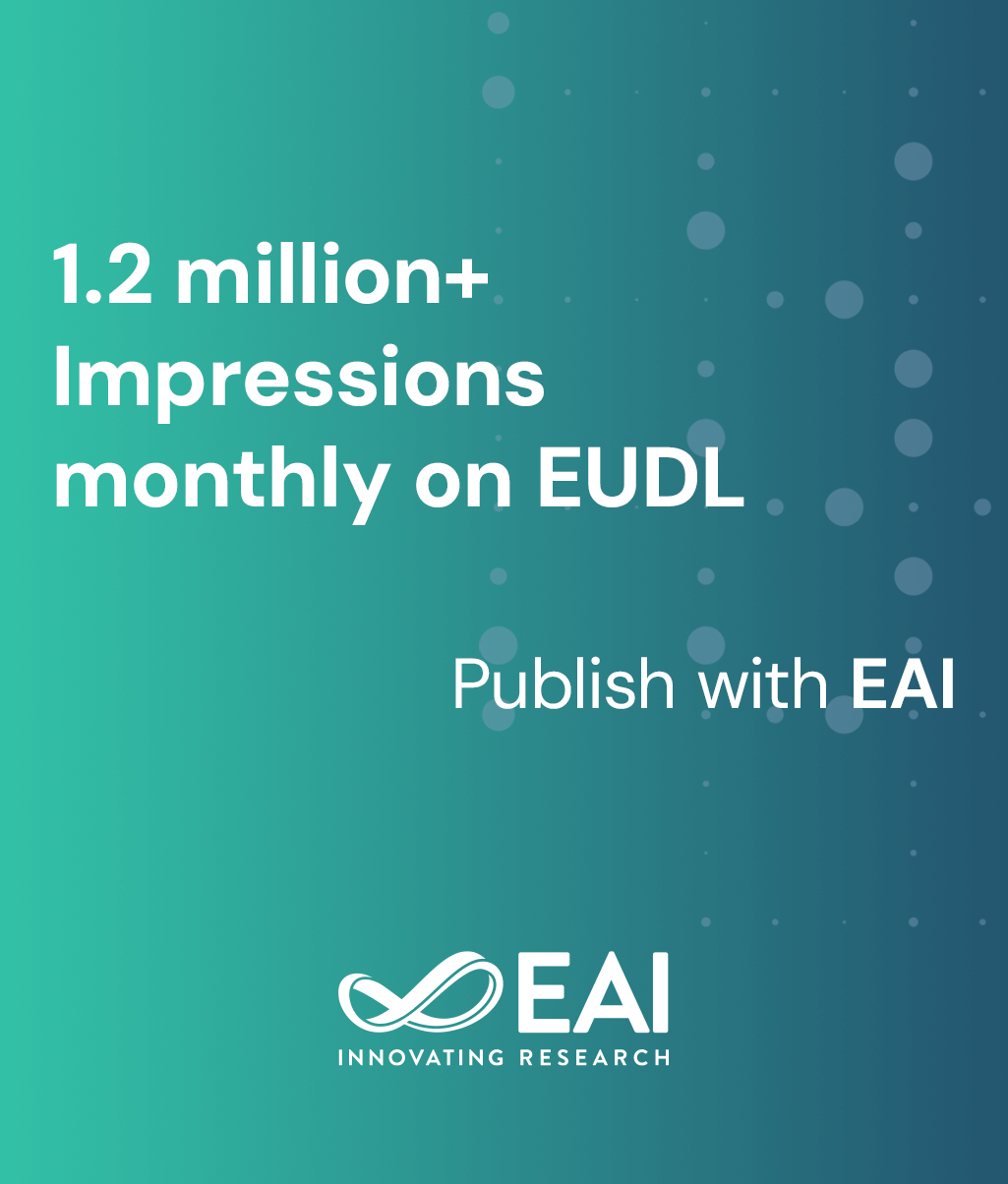
Research Article
Discovering Optimal Algorithm to Predict Diabetic Retinopathy using Novel Assessment Methods
@ARTICLE{10.4108/eai.13-7-2018.165505, author={Shiva Shankar Reddy and Nilambar Sethi and R. Rajender}, title={Discovering Optimal Algorithm to Predict Diabetic Retinopathy using Novel Assessment Methods}, journal={EAI Endorsed Transactions on Scalable Information Systems}, volume={8}, number={29}, publisher={EAI}, journal_a={SIS}, year={2020}, month={7}, keywords={Diabetic retinopathy, random forest, decision tree, adaptive boosting, bagging, support vector machine (SVM) using Gaussian kernel (GK), accuracy, Youden’s J index, concordance, Somers’ D statistic and balanced accuracy}, doi={10.4108/eai.13-7-2018.165505} }
- Shiva Shankar Reddy
Nilambar Sethi
R. Rajender
Year: 2020
Discovering Optimal Algorithm to Predict Diabetic Retinopathy using Novel Assessment Methods
SIS
EAI
DOI: 10.4108/eai.13-7-2018.165505
Abstract
Diabetic retinopathy is a diabetes complication that effects eyes. It disrupts the vasculature of the sensitive tissue present at the back of the eye. If this complication is untreated it may lead to blindness. The aim of this work is to train a model that efficiently predicts diabetic retinopathy. Machine learning techniques like Decision tree, Random forest, Adaptive boosting and Bagging are used as primary algorithms to train predictive models. An algorithm namely ‘Support Vector Machine using Gaussian kernel for retinopathy prediction’ is proposed in this work. The proposed algorithm is compared with the primary algorithms based on five evaluation metrics namely accuracy, Youden’s J index, concordance, Somers’ D statistic and balanced accuracy. From the results obtained the proposed algorithm obtained better values for all considered evaluation metrics. Thus the use of SVM with Gaussian kernel is proposed to be used for prediction of diabetic retinopathy.
Copyright © 2020 Shiva Shankar Reddy et al., licensed to EAI. This is an open access article distributed under the terms of the Creative Commons Attribution license, which permits unlimited use, distribution and reproduction in any medium so long as the original work is properly cited.