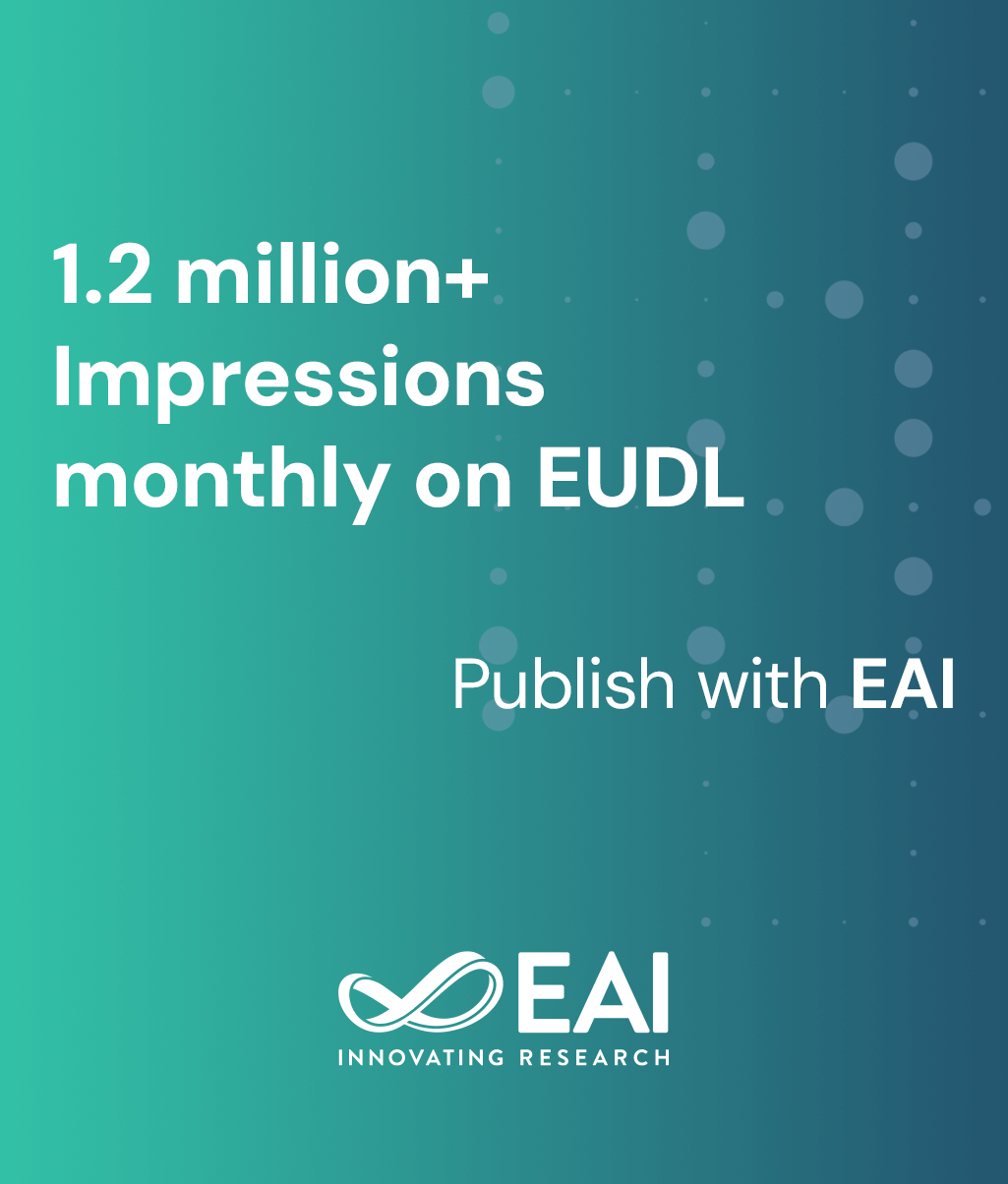
Research Article
CPAR: Cloud-Assisted Privacy-preserving Image Annotation with Randomized k-d Forest
@ARTICLE{10.4108/eai.13-7-2018.163999, author={Yifan Tian and Jiawei Yuan and Yantian Hou}, title={CPAR: Cloud-Assisted Privacy-preserving Image Annotation with Randomized k-d Forest}, journal={EAI Endorsed Transactions on Security and Safety}, volume={6}, number={22}, publisher={EAI}, journal_a={SESA}, year={2020}, month={4}, keywords={Image Annotation, Privacy-preserving, Cloud Computing}, doi={10.4108/eai.13-7-2018.163999} }
- Yifan Tian
Jiawei Yuan
Yantian Hou
Year: 2020
CPAR: Cloud-Assisted Privacy-preserving Image Annotation with Randomized k-d Forest
SESA
EAI
DOI: 10.4108/eai.13-7-2018.163999
Abstract
With the explosive growth in the number of pictures taken by smartphones, organizing and searching pictures has become important tasks. To efficiently fulfill these tasks, the key enabler is annotating images with proper keywords, with which keyword-based searching and organizing become available for images. Currently, smartphones usually synchronize photo albums with cloud storage platforms, and have their images annotated with the help of cloud computing. However, the “offloading-to-cloud” solution may cause privacy breach, since photos from smart photos contain various sensitive information. For privacy protection, existing research made effort to support cloud-based image annotation on encrypted images by utilizing cryptographic primitives. Nevertheless, for each annotation, it requires the cloud to perform linear checking on the large-scale encrypted dataset with high computational cost. This paper proposes a cloud-assisted privacy-preserving image annotation with randomized k-d forest, namely CPAR. With CPAR, users are able to automatically assign keywords to their images by leveraging the power of cloud with privacy protected. CPAR proposes a novel privacy-preserving randomized k-d forest structure, which significantly improves the annotation performance compared with existing research. Thorough analysis is carried out to demonstrate the security of CPAR. Experimental evaluation on the well-known IAPR TC-12 dataset validates the efficiency and effectiveness of CPAR.
Copyright © 2020 Y. Tian et al., licensed to EAI. This is an open access article distributed under the terms of the Creative Commons Attribution license (http://creativecommons.org/licenses/by/3.0/), which permits unlimited use, distribution and reproduction in any medium so long as the original work is properly cited.